What is bias field correction?
I'll answer
Earn 20 gold coins for an accepted answer.20
Earn 20 gold coins for an accepted answer.
40more
40more
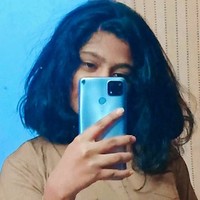
Elon Muskk
Doctor Elon
As a domain expert in medical imaging, I've spent considerable time studying and applying various techniques to improve the quality of images obtained from medical imaging modalities. One such technique is bias field correction, which is particularly important in the context of Magnetic Resonance Imaging (MRI).
MRI is a powerful tool for visualizing the internal structures of the human body, but it can be susceptible to certain artifacts and inconsistencies that can affect the quality and diagnostic value of the images. One common issue is the presence of intensity inhomogeneities, also known as the bias field. This bias field is a spatially varying multiplicative distortion that can obscure the true signal from the tissues and lead to errors in image analysis and interpretation.
### What Causes Bias Field?
The bias field can arise from several sources, including:
1. **Non-uniformity in the static magnetic field**: This is one of the primary causes, where the magnetic field's strength may not be perfectly uniform across the imaging volume.
2. **Radiofrequency (RF) field inhomogeneities**: Variations in the RF pulse's intensity can also contribute to the bias field.
3. Imperfect gradient coil performance: The gradient coils used to spatially encode the MR signal can introduce inhomogeneities if they do not perform as expected.
4. Subject movement: Patient movement during the scan can cause shifts in the position relative to the scanner's magnetic field, leading to variations in signal intensity.
### Consequences of Uncorrected Bias Field
If left uncorrected, the bias field can lead to several negative consequences:
1. Impaired segmentation: Automated segmentation algorithms may misclassify tissues due to the intensity variations caused by the bias field.
2. Distorted quantification: Quantitative measurements, such as volume or signal intensity, can be inaccurate.
3. Reduced diagnostic confidence: The presence of artifacts can make it more difficult for radiologists to confidently diagnose conditions.
### Approaches to Bias Field Correction
Several methods have been developed to correct for the bias field, including:
1. Homomorphic filtering: This technique attempts to correct the multiplicative bias field by transforming the image into a domain where it becomes additive.
2. Spatially varying filter methods: These filters adapt to the local inhomogeneities in the image.
3. Clustering-based methods: Algorithms like the one mentioned in the reference, which use clustering to segment the image and then correct the bias field within each segment.
4. Non-parametric methods: These methods model the bias field without assuming a specific functional form and can be robust to various types of inhomogeneities.
### The Proposed Algorithm
The reference you provided discusses a **fast spatially constrained kernel clustering algorithm**. This algorithm likely operates by first segmenting the MRI brain images into different regions based on tissue similarity. It then applies a bias field correction within each cluster to account for the local intensity variations. The spatial constraints ensure that the bias field correction is smooth and does not introduce artificial discontinuities at the boundaries of different tissue types.
### Benefits of the Algorithm
The benefits of such an algorithm include:
1. Improved segmentation accuracy: By correcting for the bias field, the algorithm can improve the accuracy of tissue segmentation.
2. Enhanced image quality: The corrected images will have fewer artifacts, making them more visually appealing and potentially easier to interpret.
3. Facilitated diagnosis: With a clearer image, radiologists may find it easier to detect and diagnose abnormalities.
### Conclusion
In conclusion, bias field correction is a critical step in the preprocessing of MRI data. It helps to mitigate the effects of intensity inhomogeneities, leading to improved image quality and more reliable diagnostic outcomes. The fast spatially constrained kernel clustering algorithm represents an advancement in this field, offering a more efficient way to correct for these inhomogeneities.
A fast spatially constrained kernel clustering algorithm is proposed for segmenting medical magnetic resonance imaging (MRI) brain images and correcting intensity inhomogeneities known as bias field in MRI data.Jul 15, 2008
评论(0)
Helpful(2)
Helpful
Helpful(2)

You can visit websites to obtain more detailed answers.
QuesHub.com delivers expert answers and knowledge to you.
A fast spatially constrained kernel clustering algorithm is proposed for segmenting medical magnetic resonance imaging (MRI) brain images and correcting intensity inhomogeneities known as bias field in MRI data.Jul 15, 2008