What is 2 standard deviations?
I'll answer
Earn 20 gold coins for an accepted answer.20
Earn 20 gold coins for an accepted answer.
40more
40more

Ava Collins
Studied at University of California, Berkeley, Lives in San Francisco. Entrepreneur passionate about technology and innovation.
As a domain expert in statistics, I'm delighted to provide an in-depth explanation of the concept of "2 standard deviations", which is a fundamental concept in the field of statistics and data analysis.
In statistics, standard deviation is a measure of the amount of variation or dispersion in a set of values. A low standard deviation indicates that the values tend to be close to the mean (also called the expected value) of the set, while a high standard deviation indicates that the values are spread out over a wider range.
When we talk about "2 standard deviations," we're referring to a range that extends from the mean minus two standard deviations to the mean plus two standard deviations. This concept is particularly important when dealing with a normal distribution, which is a type of continuous probability distribution for a real-valued random variable. The normal distribution is defined by its mean (\( \mu \)) and variance (\( \sigma^2 \)), and it is also known as the Gaussian distribution.
The significance of 2 standard deviations in the context of a normal distribution is rooted in the empirical rule, which is a statistical rule that states that for a normal distribution:
- Approximately 68% of the data values fall within one standard deviation (\( \mu \pm \sigma \)) of the mean.
- Approximately 95% of the data values fall within two standard deviations (\( \mu \pm 2\sigma \)) of the mean.
- Approximately 99.7% of the data values fall within three standard deviations (\( \mu \pm 3\sigma \)) of the mean.
This rule is often visualized using a bell curve, where the majority of the data is clustered around the mean, and the frequency of data points decreases as you move further away from the mean.
The concept of 2 standard deviations is also used in constructing confidence intervals. For instance, a 95% confidence interval for the mean of a population, based on a sample mean, is often calculated as the sample mean plus or minus 2 times the standard error (which is the standard deviation divided by the square root of the sample size).
In practical applications, understanding 2 standard deviations can help in various fields such as finance, where it can be used to measure the volatility of investments, or in quality control, where it can help identify outliers in manufacturing processes.
It's important to note that the empirical rule is an approximation and it holds true only for distributions that are perfectly normal. In real-world scenarios, distributions may not be perfectly normal, and the percentages may vary slightly.
In conclusion, the concept of 2 standard deviations is a powerful tool in statistical analysis that provides a way to quantify the spread of data and to make inferences about the population from which a sample is drawn. It is a cornerstone of many statistical methods and is widely used in both theoretical and applied statistics.
In statistics, standard deviation is a measure of the amount of variation or dispersion in a set of values. A low standard deviation indicates that the values tend to be close to the mean (also called the expected value) of the set, while a high standard deviation indicates that the values are spread out over a wider range.
When we talk about "2 standard deviations," we're referring to a range that extends from the mean minus two standard deviations to the mean plus two standard deviations. This concept is particularly important when dealing with a normal distribution, which is a type of continuous probability distribution for a real-valued random variable. The normal distribution is defined by its mean (\( \mu \)) and variance (\( \sigma^2 \)), and it is also known as the Gaussian distribution.
The significance of 2 standard deviations in the context of a normal distribution is rooted in the empirical rule, which is a statistical rule that states that for a normal distribution:
- Approximately 68% of the data values fall within one standard deviation (\( \mu \pm \sigma \)) of the mean.
- Approximately 95% of the data values fall within two standard deviations (\( \mu \pm 2\sigma \)) of the mean.
- Approximately 99.7% of the data values fall within three standard deviations (\( \mu \pm 3\sigma \)) of the mean.
This rule is often visualized using a bell curve, where the majority of the data is clustered around the mean, and the frequency of data points decreases as you move further away from the mean.
The concept of 2 standard deviations is also used in constructing confidence intervals. For instance, a 95% confidence interval for the mean of a population, based on a sample mean, is often calculated as the sample mean plus or minus 2 times the standard error (which is the standard deviation divided by the square root of the sample size).
In practical applications, understanding 2 standard deviations can help in various fields such as finance, where it can be used to measure the volatility of investments, or in quality control, where it can help identify outliers in manufacturing processes.
It's important to note that the empirical rule is an approximation and it holds true only for distributions that are perfectly normal. In real-world scenarios, distributions may not be perfectly normal, and the percentages may vary slightly.
In conclusion, the concept of 2 standard deviations is a powerful tool in statistical analysis that provides a way to quantify the spread of data and to make inferences about the population from which a sample is drawn. It is a cornerstone of many statistical methods and is widely used in both theoretical and applied statistics.
2024-04-07 08:18:13
reply(1)
Helpful(1122)
Helpful
Helpful(2)
Studied at the University of Copenhagen, Lives in Copenhagen, Denmark.
If a data distribution is approximately normal then about 68 percent of the data values are within one standard deviation of the mean (mathematically, -- -- --, where -- is the arithmetic mean), about 95 percent are within two standard deviations (-- -- 2--), and about 99.7 percent lie within three standard deviations (-- -- 3-- ...
2023-06-25 11:09:53
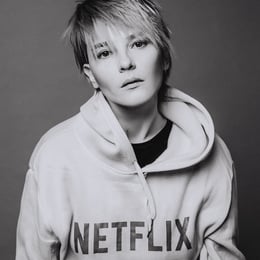
Mason Hall
QuesHub.com delivers expert answers and knowledge to you.
If a data distribution is approximately normal then about 68 percent of the data values are within one standard deviation of the mean (mathematically, -- -- --, where -- is the arithmetic mean), about 95 percent are within two standard deviations (-- -- 2--), and about 99.7 percent lie within three standard deviations (-- -- 3-- ...