What does the sum of squares tell us?
I'll answer
Earn 20 gold coins for an accepted answer.20
Earn 20 gold coins for an accepted answer.
40more
40more
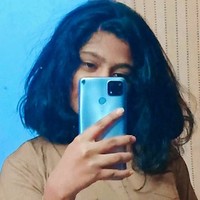
Elon Muskk
Doctor Elon
As a statistical expert with a strong background in data analysis, I'm often asked about the significance of the sum of squares in statistical studies. The sum of squares is a fundamental concept that is used to quantify the variation within a dataset. It is a measure of the total variability in the data, and it is often decomposed into components that can be attributed to different sources of variation. Understanding the sum of squares is crucial for various statistical methods, including regression analysis, analysis of variance (ANOVA), and principal component analysis (PCA).
Let's delve into the concept of the sum of squares and its implications in more detail.
### What is the Sum of Squares (SST)?
The sum of squares, also known as the total sum of squares (TSS), is calculated by taking each observation in a dataset, subtracting the overall mean of the dataset, squaring the result, and then summing these squared differences for all observations. The formula for the total sum of squares is:
\[ TSS = \sum_{i=1}^{n} (x_i - \bar{x})^2 \]
where \( x_i \) represents each observation, \( \bar{x} \) is the mean of all observations, and \( n \) is the total number of observations.
### Why is the Sum of Squares Important?
1. Quantifying Variability: The sum of squares provides a numerical measure of the variability or dispersion within a dataset. A larger TSS indicates a greater degree of variability among the observations.
2. Decomposition into Components: The total sum of squares can be decomposed into parts that represent the sum of squares due to regression (SSR) and the sum of squares due to error (SSE). This partitioning is essential in regression analysis, where it helps to understand how much of the variability in the dependent variable can be explained by the independent variables.
3. Analysis of Variance (ANOVA): In ANOVA, the sum of squares is used to test for differences between group means. By comparing the sum of squares between groups (SSB) and within groups (SSW), researchers can determine if the variation between groups is significantly different from the variation within groups.
4. Efficiency in Model Selection: The sum of squares is used to calculate the coefficient of determination (R²), which is a measure of the proportion of the variance in the dependent variable that is predictable from the independent variable(s).
5. Principal Component Analysis (PCA): In PCA, the sum of squares is used to identify the principal components that explain the most variance in the data. This is particularly useful for dimensionality reduction and feature extraction in large datasets.
6. Statistical Inference: The sum of squares plays a role in hypothesis testing and confidence interval estimation. It is used to calculate test statistics such as the F-statistic in ANOVA and the t-statistic in regression analysis.
### Interpreting the Sum of Squares
When interpreting the sum of squares, it's important to consider the context of the analysis. For instance, in regression analysis, a high SSR relative to TSS indicates that the model is doing a good job of explaining the variability in the dependent variable. Conversely, a high SSE suggests that there is unexplained variability, which could be due to random error or omitted variables.
### Limitations and Considerations
While the sum of squares is a powerful tool, it's not without limitations. It's sensitive to outliers and leverage points, which can disproportionately influence the results. Additionally, the sum of squares does not take into account the scale of the variables, which can be a concern when comparing different datasets or variables.
In conclusion, the sum of squares is a versatile and essential concept in statistical analysis. It provides a measure of total variability, allows for the decomposition of variability into meaningful components, and is integral to many statistical methods and models. Understanding how to calculate and interpret the sum of squares is key to drawing accurate and insightful conclusions from data.
In statistical data analysis the total sum of squares (TSS or SST) is a quantity that appears as part of a standard way of presenting results of such analyses. It is defined as being the sum, over all observations, of the squared differences of each observation from the overall mean.
评论(0)
Helpful(2)
Helpful
Helpful(2)

You can visit websites to obtain more detailed answers.
QuesHub.com delivers expert answers and knowledge to you.
In statistical data analysis the total sum of squares (TSS or SST) is a quantity that appears as part of a standard way of presenting results of such analyses. It is defined as being the sum, over all observations, of the squared differences of each observation from the overall mean.