What is a good value for R Squared?
I'll answer
Earn 20 gold coins for an accepted answer.20
Earn 20 gold coins for an accepted answer.
40more
40more
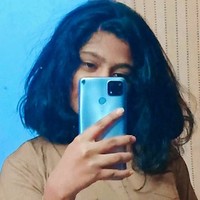
Elon Muskk
Doctor Elon
As a domain expert in statistical analysis, I often encounter questions regarding the interpretation of various statistical measures, including the concept of R-squared. R-squared, denoted as \( R^2 \), is a statistical tool used to assess the performance of a regression model. It provides an indication of how well the observed outcomes are replicated by the model.
Step 1: Understanding R-squared
R-squared is a statistical measure of how close the data are to the fitted regression line. It is also known as the coefficient of determination, or the coefficient of multiple determination for multiple regression. It essentially measures the proportion of the variance in the dependent variable that is predictable from the independent variable(s).
The value of \( R^2 \) ranges from 0 to 1. A value of 0% indicates that the model explains none of the variability of the dependent variable around its mean, while a value of 100% indicates that the model explains all the variability of the response data around its mean.
Step 2: Interpreting R-squared Values
When interpreting \( R^2 \) values, it's important to consider the context of the data and the field of study. Here are some general guidelines:
- 0.00 to 0.20: Indicates that the model explains a very small amount of the variability in the response variable.
- 0.21 to 0.40: The model explains a fair amount of the variability.
- 0.41 to 0.60: The model explains a moderate amount of the variability.
- 0.61 to 0.80: The model explains a substantial portion of the variability.
- 0.81 to 1.00: The model explains almost all of the variability.
However, a high \( R^2 \) value does not necessarily mean the model is good. It is possible to have a model with a high \( R^2 \) that is overfitted to the data, meaning it may not generalize well to new, unseen data.
**Step 3: Considerations for a Good R-squared Value**
A good value for \( R^2 \) depends on several factors:
1. Field of Study: In some fields, a lower \( R^2 \) might be acceptable due to the nature of the data or the complexity of the phenomena being studied.
2. Model Complexity: A more complex model with more predictors might achieve a higher \( R^2 \), but it could also be more prone to overfitting.
3. Data Quality: The quality of the data used in the model can greatly affect the \( R^2 \) value. Poor quality data can lead to misleading results.
4. Research Questions: The specific research questions and objectives can influence what constitutes a good \( R^2 \) value. For predictive models, a higher \( R^2 \) might be desirable, whereas for explanatory models, a lower value might be acceptable.
5. Comparative Analysis: It's often helpful to compare the \( R^2 \) of your model to those of similar models in the literature.
Step 4: Beyond R-squared
While \( R^2 \) is a useful statistic, it should not be the sole measure of a model's performance. Other metrics such as adjusted \( R^2 \), which accounts for the number of predictors in the model, and the root-mean-square error (RMSE), which measures the average magnitude of the residuals, should also be considered.
Step 5: Conclusion
In conclusion, there is no one-size-fits-all answer to what constitutes a good \( R^2 \) value. It is a context-dependent measure that should be interpreted alongside other statistical measures and in light of the research questions and objectives. It is also crucial to ensure that the model is not overfitted and that it can generalize well to new data.
R-squared is a statistical measure of how close the data are to the fitted regression line. It is also known as the coefficient of determination, or the coefficient of multiple determination for multiple regression. 0% indicates that the model explains none of the variability of the response data around its mean.Apr 22, 2015
评论(0)
Helpful(2)
Helpful
Helpful(2)

You can visit websites to obtain more detailed answers.
QuesHub.com delivers expert answers and knowledge to you.
R-squared is a statistical measure of how close the data are to the fitted regression line. It is also known as the coefficient of determination, or the coefficient of multiple determination for multiple regression. 0% indicates that the model explains none of the variability of the response data around its mean.Apr 22, 2015