Why is effect size important in statistics?
I'll answer
Earn 20 gold coins for an accepted answer.20
Earn 20 gold coins for an accepted answer.
40more
40more
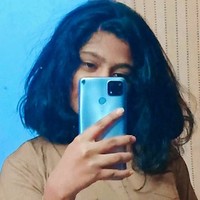
Elon Muskk
Doctor Elon
As a statistical expert with a deep understanding of the nuances involved in data analysis, I can explain why effect size is crucial in statistics. Effect size is a measure of the strength or magnitude of a phenomenon. It's an essential concept because it allows researchers to quantify the practical significance of their findings, not just the statistical significance.
Statistical significance tells us whether the observed results are likely to have occurred by chance. It's determined by a p-value, which is the probability of observing the data (or something more extreme) if the null hypothesis is true. A common threshold for significance is a p-value less than 0.05, indicating that there's less than a 5% chance that the results occurred by random chance.
However, statistical significance does not necessarily imply that the findings are large or important in a real-world context. This is where effect size comes into play. It provides an estimate of how large the effect is, which can be critical for making decisions based on the results of a study.
### Importance of Effect Size:
1. Practical Significance: Even if a result is statistically significant, if the effect size is very small, it may not be meaningful in practical terms. For example, a new drug might show a statistically significant improvement over a placebo, but if the improvement is minimal, it may not be worth the cost or potential side effects.
2. Sample Size Influence: Statistical significance can be influenced by the sample size. A large sample size can lead to statistically significant results even for very small effect sizes. This is known as the "playground bully" problem, where the sheer size of the sample can overpower the effect size and lead to significant results that aren't practically important.
3. Replicability: Small effect sizes can be harder to replicate because they are more susceptible to random variation. Larger effect sizes are more likely to be consistent across different studies and samples.
4. Research Planning: Knowing the effect size can help in planning future research. It can guide decisions about the necessary sample size to detect an effect of that magnitude.
5. Interpretation of Results: Effect size provides a common metric for comparing the strength of effects across different studies, even if the studies are in different fields or use different measurement scales.
6. Meta-Analysis: In meta-analytical techniques, effect sizes are aggregated across multiple studies to draw conclusions about the overall effect. This would not be possible if we only considered statistical significance.
7. Clinical or Applied Decision Making: In fields like medicine or psychology, effect sizes can inform decisions about treatment efficacy or intervention strategies.
8. Avoiding False Negatives and Positives: Focusing solely on statistical significance without considering effect size can lead to false conclusions. A large effect size with a non-significant p-value (due to small sample size) might be more informative than a small effect size with a significant p-value.
9. Publication Bias: Studies with statistically significant results are more likely to be published, which can skew the literature towards significant findings, regardless of the size of the effect.
10. Transparency and Open Science: Reporting effect sizes promotes transparency in research and supports the principles of open science, as it allows others to understand the magnitude of the findings, not just whether they are statistically significant.
In summary, while statistical significance is a gatekeeper for determining if an effect is likely non-random, effect size is the key to understanding the importance of that effect. It's the bridge between statistical analysis and real-world application.
Statistical significance is the probability that the observed difference between two groups is due to chance. ... Statistical significance, on the other hand, depends upon both sample size and effect size. For this reason, P values are considered to be confounded because of their dependence on sample size.
评论(0)
Helpful(2)
Helpful
Helpful(2)

You can visit websites to obtain more detailed answers.
QuesHub.com delivers expert answers and knowledge to you.
Statistical significance is the probability that the observed difference between two groups is due to chance. ... Statistical significance, on the other hand, depends upon both sample size and effect size. For this reason, P values are considered to be confounded because of their dependence on sample size.