Why is it bad to have a small sample size?
I'll answer
Earn 20 gold coins for an accepted answer.20
Earn 20 gold coins for an accepted answer.
40more
40more

Clara Price
Studied at McGill University, Lives in Montreal, Canada
As a seasoned statistician with extensive experience in data analysis and statistical modeling, I have often encountered the question of why a small sample size can be problematic in statistical research. Let's delve into the intricacies of this issue by examining the implications of sample size on the validity and reliability of statistical inferences.
Step 1: English Explanation
**Why is it bad to have a small sample size?**
**1. Reduced Representation of the Population:** The first reason to understand why a large sample size is beneficial is simple. Larger samples more closely approximate the population. Because the primary goal of inferential statistics is to generalize from a sample to a population, it is less of an inference if the sample size is large. Small samples may not capture the diversity and variability inherent in the population, leading to a biased representation.
2. Increased Variability: Smaller samples are subject to greater variability. When you have a small sample, the results of your statistical tests are more likely to be influenced by the specific individuals within that sample rather than the population as a whole. This can lead to a higher degree of sampling error, which is the difference between the sample statistic and the true population parameter.
3. Lower Statistical Power: Statistical power is the probability that a test will reject the null hypothesis when the alternative hypothesis is true (i.e., that there is a real effect). With a small sample size, the statistical power is reduced, making it more difficult to detect a true effect if one exists.
4. Reduced Confidence in Results: Confidence intervals provide a range within which we can be confident the true population parameter lies. With a small sample size, these intervals tend to be wider, reflecting greater uncertainty about the population parameter.
5. Greater Sensitivity to Outliers: Small samples are more susceptible to the influence of outliers. A single outlier can significantly skew the results of an analysis, leading to misleading conclusions.
**6. Limited Ability to Detect Subgroup Differences:** When the goal is to compare different subgroups within a population, a small sample size may not provide enough data to detect meaningful differences between these groups.
7. Potential for Overfitting: In predictive modeling, small sample sizes can lead to overfitting, where a model is too closely tailored to the sample data and may not generalize well to new, unseen data.
8. Ethical Considerations: In some fields, particularly in medical and psychological research, collecting a large sample size is not only a methodological choice but also an ethical imperative to ensure that the results are robust and applicable to a broader population.
9. Practical Limitations: Small sample sizes can sometimes be a result of practical constraints, such as limited funding or access to subjects. However, relying on small samples can limit the scope and impact of research findings.
10. Replicability Concerns: Studies based on small samples are often less replicable. The scientific method relies on the ability to reproduce results, and studies with larger sample sizes are generally more likely to be replicated successfully.
In conclusion, while small sample sizes can sometimes be unavoidable, they present numerous challenges that can compromise the integrity and applicability of statistical findings. It is always preferable to work with larger samples when possible to ensure that the results are robust and generalizable.
**
Step 1: English Explanation
**Why is it bad to have a small sample size?**
**1. Reduced Representation of the Population:** The first reason to understand why a large sample size is beneficial is simple. Larger samples more closely approximate the population. Because the primary goal of inferential statistics is to generalize from a sample to a population, it is less of an inference if the sample size is large. Small samples may not capture the diversity and variability inherent in the population, leading to a biased representation.
2. Increased Variability: Smaller samples are subject to greater variability. When you have a small sample, the results of your statistical tests are more likely to be influenced by the specific individuals within that sample rather than the population as a whole. This can lead to a higher degree of sampling error, which is the difference between the sample statistic and the true population parameter.
3. Lower Statistical Power: Statistical power is the probability that a test will reject the null hypothesis when the alternative hypothesis is true (i.e., that there is a real effect). With a small sample size, the statistical power is reduced, making it more difficult to detect a true effect if one exists.
4. Reduced Confidence in Results: Confidence intervals provide a range within which we can be confident the true population parameter lies. With a small sample size, these intervals tend to be wider, reflecting greater uncertainty about the population parameter.
5. Greater Sensitivity to Outliers: Small samples are more susceptible to the influence of outliers. A single outlier can significantly skew the results of an analysis, leading to misleading conclusions.
**6. Limited Ability to Detect Subgroup Differences:** When the goal is to compare different subgroups within a population, a small sample size may not provide enough data to detect meaningful differences between these groups.
7. Potential for Overfitting: In predictive modeling, small sample sizes can lead to overfitting, where a model is too closely tailored to the sample data and may not generalize well to new, unseen data.
8. Ethical Considerations: In some fields, particularly in medical and psychological research, collecting a large sample size is not only a methodological choice but also an ethical imperative to ensure that the results are robust and applicable to a broader population.
9. Practical Limitations: Small sample sizes can sometimes be a result of practical constraints, such as limited funding or access to subjects. However, relying on small samples can limit the scope and impact of research findings.
10. Replicability Concerns: Studies based on small samples are often less replicable. The scientific method relies on the ability to reproduce results, and studies with larger sample sizes are generally more likely to be replicated successfully.
In conclusion, while small sample sizes can sometimes be unavoidable, they present numerous challenges that can compromise the integrity and applicability of statistical findings. It is always preferable to work with larger samples when possible to ensure that the results are robust and generalizable.
**
2024-04-05 15:45:58
reply(1)
Helpful(1122)
Helpful
Helpful(2)
Studied at University of Oxford, Lives in Oxford, UK
The first reason to understand why a large sample size is beneficial is simple. Larger samples more closely approximate the population. Because the primary goal of inferential statistics is to generalize from a sample to a population, it is less of an inference if the sample size is large. ... Small samples are bad.
2023-06-23 09:46:20
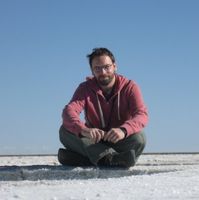
Oliver Gonzalez
QuesHub.com delivers expert answers and knowledge to you.
The first reason to understand why a large sample size is beneficial is simple. Larger samples more closely approximate the population. Because the primary goal of inferential statistics is to generalize from a sample to a population, it is less of an inference if the sample size is large. ... Small samples are bad.