What is variability due to sampling?
I'll answer
Earn 20 gold coins for an accepted answer.20
Earn 20 gold coins for an accepted answer.
40more
40more

Harper Gray
Studied at the University of Barcelona, Lives in Barcelona, Spain.
As a statistical expert with a deep understanding of the intricacies of data analysis, I can provide an insightful explanation of variability due to sampling. This concept is fundamental in statistical inference, which is the process of drawing conclusions from data that are subject to random variation.
Sampling variability refers to the fluctuations that occur in the results of statistical analyses when different samples are drawn from the same population. It is a natural consequence of sampling because each sample is a subset of the population and may not perfectly represent the entire population.
When we take a sample from a population, we are essentially making an estimate about the population based on that subset of data. However, due to the inherent randomness in the selection process, different samples will generally yield different results. This is where sampling variability comes into play.
The variance (\( \sigma^2 \)) and standard deviation (\( \sigma \)) are indeed common measures of variability. Variance is the average of the squared differences from the mean, and standard deviation is the square root of the variance. These measures give us an indication of how much the data points in a sample are spread out from the mean.
In the context of sampling variability, we often talk about the variability of the sample mean (\( \bar{x} \)). The sample mean is the average of the values in a sample. When we say that there is variability in the sample mean, we mean that if we were to take multiple samples from the same population, the means of those samples would differ from each other and from the true population mean.
The degree of sampling variability is influenced by several factors:
1. Sample Size (n): Larger samples tend to have less variability because they are more representative of the population.
2. Population Variance: A population with a high variance will have greater sampling variability because there is more spread in the data.
3. Sampling Method: The method used to select the sample (e.g., simple random sampling, stratified sampling) can affect the variability.
4. Population Size (N): The size of the population can also influence the variability, especially when the sample size is a significant proportion of the population.
To manage and understand sampling variability, statisticians use concepts like the standard error of the mean, which is the standard deviation of the sampling distribution of the mean. The standard error is a measure of how much the sample mean is expected to vary from the true population mean.
In practical terms, sampling variability is crucial for constructing confidence intervals and conducting hypothesis tests. It allows researchers to make inferences about the population while acknowledging the uncertainty inherent in their estimates.
In conclusion, sampling variability is a critical concept in statistics that reflects the natural fluctuations in estimates that arise from taking different samples from a population. It is essential for making valid statistical inferences and for understanding the limitations of our conclusions when working with sample data.
Sampling variability refers to the fluctuations that occur in the results of statistical analyses when different samples are drawn from the same population. It is a natural consequence of sampling because each sample is a subset of the population and may not perfectly represent the entire population.
When we take a sample from a population, we are essentially making an estimate about the population based on that subset of data. However, due to the inherent randomness in the selection process, different samples will generally yield different results. This is where sampling variability comes into play.
The variance (\( \sigma^2 \)) and standard deviation (\( \sigma \)) are indeed common measures of variability. Variance is the average of the squared differences from the mean, and standard deviation is the square root of the variance. These measures give us an indication of how much the data points in a sample are spread out from the mean.
In the context of sampling variability, we often talk about the variability of the sample mean (\( \bar{x} \)). The sample mean is the average of the values in a sample. When we say that there is variability in the sample mean, we mean that if we were to take multiple samples from the same population, the means of those samples would differ from each other and from the true population mean.
The degree of sampling variability is influenced by several factors:
1. Sample Size (n): Larger samples tend to have less variability because they are more representative of the population.
2. Population Variance: A population with a high variance will have greater sampling variability because there is more spread in the data.
3. Sampling Method: The method used to select the sample (e.g., simple random sampling, stratified sampling) can affect the variability.
4. Population Size (N): The size of the population can also influence the variability, especially when the sample size is a significant proportion of the population.
To manage and understand sampling variability, statisticians use concepts like the standard error of the mean, which is the standard deviation of the sampling distribution of the mean. The standard error is a measure of how much the sample mean is expected to vary from the true population mean.
In practical terms, sampling variability is crucial for constructing confidence intervals and conducting hypothesis tests. It allows researchers to make inferences about the population while acknowledging the uncertainty inherent in their estimates.
In conclusion, sampling variability is a critical concept in statistics that reflects the natural fluctuations in estimates that arise from taking different samples from a population. It is essential for making valid statistical inferences and for understanding the limitations of our conclusions when working with sample data.
2024-04-26 21:58:18
reply(1)
Helpful(1122)
Helpful
Helpful(2)
Studied at Harvard University, Lives in Cambridge, MA
Sampling variability is how much an estimate varies between samples. ... The variance (--2) and standard deviation (--) are common measures of variability. You might also see reference to the variability of the sample mean (x&772;), which is just another way of saying the sample mean differs from sample to sample.May 18, 2015
2023-06-17 09:46:19
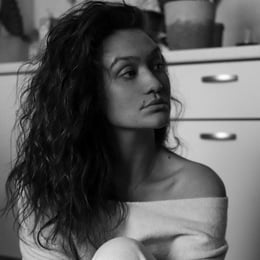
Charlotte Baker
QuesHub.com delivers expert answers and knowledge to you.
Sampling variability is how much an estimate varies between samples. ... The variance (--2) and standard deviation (--) are common measures of variability. You might also see reference to the variability of the sample mean (x&772;), which is just another way of saying the sample mean differs from sample to sample.May 18, 2015