What is the f value in statistics?
I'll answer
Earn 20 gold coins for an accepted answer.20
Earn 20 gold coins for an accepted answer.
40more
40more
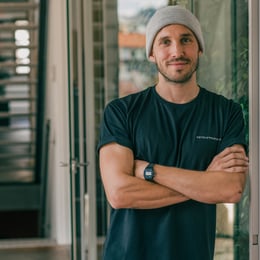
Liam Patel
Works at GreenTech Innovations, Lives in Bangalore, India.
As a statistical expert with a deep understanding of the field, I am well-equipped to discuss the nuances of statistical tests and their applications. One of the key concepts in statistics is the F-value, which plays a pivotal role in various analyses, particularly when comparing models or assessing variances.
The F-value is derived from the F-distribution, a type of continuous probability distribution that arises naturally in the process of comparing variances of two or more groups. It is named after the statistician Ronald Fisher, who introduced the concept in the context of the analysis of variance (ANOVA).
### Understanding the F-Value
The F-value is calculated as the ratio of two variance estimates. It is used to test the null hypothesis that there is no significant difference between the variances of two groups. The formula for the F-value in the context of comparing two variances (say, \( \sigma_1^2 \) and \( \sigma_2^2 \)) is:
\[ F = \frac{\sigma_1^2 / \nu_1}{\sigma_2^2 / \nu_2} \]
Where \( \nu_1 \) and \( \nu_2 \) are the degrees of freedom associated with the two variances.
### Applications of the F-Value
The F-value is most commonly used in the following statistical tests:
1. ANOVA (Analysis of Variance): This is a collection of procedures used to compare means of two or more groups. It is based on the F-distribution and is used to determine if there are any statistically significant differences between the group means.
2. Regression Analysis: In the context of regression, the F-test is used to determine whether the regression as a whole is statistically significant. It compares the total variability in the data that can be explained by the model against the variability that cannot be explained.
3. Fisher's Combined Test: This is used when multiple independent tests are performed, and the results are combined to increase the power of the test.
4. Two-Factor ANOVA: When looking at the interaction between two categorical variables, the F-test helps determine if there is a significant interaction effect.
### Significance of the F-Value
The significance of the F-value is determined by comparing it to the critical value from the F-distribution table, which is based on the degrees of freedom and the chosen significance level (often denoted as \( \alpha \)).
- If the calculated F-value is greater than the critical value, the null hypothesis is rejected, indicating that there is a significant difference between the variances or models being compared.
- If the F-value is less than or equal to the critical value, the null hypothesis is not rejected, suggesting that there is not enough evidence to conclude a significant difference.
### Assumptions and Limitations
When using the F-test, it is important to consider the following assumptions:
1. Normality: The data should be normally distributed within each group.
2. Independence: Observations should be independent of one another.
3. Homogeneity of Variance: This is specifically important in ANOVA, where the assumption is that the variances of the populations are equal.
Failure to meet these assumptions can lead to misleading results.
### Conclusion
The F-value is a critical component in statistical analysis, particularly for model comparison and variance assessment. It provides a standardized measure that allows for the comparison of variances across different groups or models. Understanding how to calculate and interpret the F-value is essential for drawing accurate conclusions from statistical data.
Now, let's proceed with the translation into Chinese.
The F-value is derived from the F-distribution, a type of continuous probability distribution that arises naturally in the process of comparing variances of two or more groups. It is named after the statistician Ronald Fisher, who introduced the concept in the context of the analysis of variance (ANOVA).
### Understanding the F-Value
The F-value is calculated as the ratio of two variance estimates. It is used to test the null hypothesis that there is no significant difference between the variances of two groups. The formula for the F-value in the context of comparing two variances (say, \( \sigma_1^2 \) and \( \sigma_2^2 \)) is:
\[ F = \frac{\sigma_1^2 / \nu_1}{\sigma_2^2 / \nu_2} \]
Where \( \nu_1 \) and \( \nu_2 \) are the degrees of freedom associated with the two variances.
### Applications of the F-Value
The F-value is most commonly used in the following statistical tests:
1. ANOVA (Analysis of Variance): This is a collection of procedures used to compare means of two or more groups. It is based on the F-distribution and is used to determine if there are any statistically significant differences between the group means.
2. Regression Analysis: In the context of regression, the F-test is used to determine whether the regression as a whole is statistically significant. It compares the total variability in the data that can be explained by the model against the variability that cannot be explained.
3. Fisher's Combined Test: This is used when multiple independent tests are performed, and the results are combined to increase the power of the test.
4. Two-Factor ANOVA: When looking at the interaction between two categorical variables, the F-test helps determine if there is a significant interaction effect.
### Significance of the F-Value
The significance of the F-value is determined by comparing it to the critical value from the F-distribution table, which is based on the degrees of freedom and the chosen significance level (often denoted as \( \alpha \)).
- If the calculated F-value is greater than the critical value, the null hypothesis is rejected, indicating that there is a significant difference between the variances or models being compared.
- If the F-value is less than or equal to the critical value, the null hypothesis is not rejected, suggesting that there is not enough evidence to conclude a significant difference.
### Assumptions and Limitations
When using the F-test, it is important to consider the following assumptions:
1. Normality: The data should be normally distributed within each group.
2. Independence: Observations should be independent of one another.
3. Homogeneity of Variance: This is specifically important in ANOVA, where the assumption is that the variances of the populations are equal.
Failure to meet these assumptions can lead to misleading results.
### Conclusion
The F-value is a critical component in statistical analysis, particularly for model comparison and variance assessment. It provides a standardized measure that allows for the comparison of variances across different groups or models. Understanding how to calculate and interpret the F-value is essential for drawing accurate conclusions from statistical data.
Now, let's proceed with the translation into Chinese.
2024-04-12 23:26:29
reply(1)
Helpful(1122)
Helpful
Helpful(2)
Works at the International Seabed Authority, Lives in Kingston, Jamaica.
An F-test is any statistical test in which the test statistic has an F-distribution under the null hypothesis. It is most often used when comparing statistical models that have been fitted to a data set, in order to identify the model that best fits the population from which the data were sampled.
2023-06-26 09:30:34
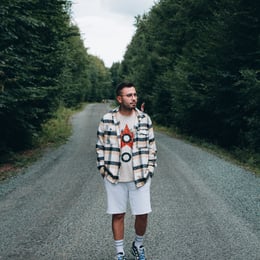
Lucas Baker
QuesHub.com delivers expert answers and knowledge to you.
An F-test is any statistical test in which the test statistic has an F-distribution under the null hypothesis. It is most often used when comparing statistical models that have been fitted to a data set, in order to identify the model that best fits the population from which the data were sampled.