What is a good P value in statistics?
I'll answer
Earn 20 gold coins for an accepted answer.20
Earn 20 gold coins for an accepted answer.
40more
40more
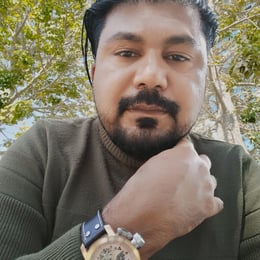
Oliver Kim
Works at the International Renewable Energy Agency, Lives in Abu Dhabi, UAE.
As a statistical expert with a deep understanding of hypothesis testing and its implications, I can provide an insightful explanation on what constitutes a good P-value in statistics. The concept of the P-value is central to statistical inference, and it is used to evaluate the strength of the evidence against a null hypothesis.
The P-value is a statistic that measures the strength of the evidence against the null hypothesis. It is calculated by assuming the null hypothesis is true and then determining the probability of observing a test statistic as extreme as, or more extreme than, the one calculated from the data. It is important to note that a P-value is not the probability that the null hypothesis is true or false; rather, it is a measure of how incompatible the data are with the null hypothesis.
In many scientific fields, a P-value of less than 0.05 is traditionally considered to be statistically significant. This threshold is somewhat arbitrary but has been widely adopted because it provides a balance between the risks of making a Type I error (rejecting a true null hypothesis) and a Type II error (failing to reject a false null hypothesis). A P-value below this threshold suggests that the observed data would be unlikely to occur if the null hypothesis were true, indicating strong evidence against the null hypothesis.
Conversely, a P-value greater than 0.05 is typically considered to indicate weak evidence against the null hypothesis. This does not mean that the null hypothesis is true, but rather that the data are not sufficiently strong to reject it. It is important to recognize that failing to reject the null hypothesis is not the same as proving it to be true.
However, it is crucial to understand that the choice of a P-value threshold is not a one-size-fits-all approach. Different fields may use different thresholds based on the costs of different types of errors, the variability of the data, and the potential impact of the results. For instance, in medical trials, a P-value of less than 0.01 might be required to demonstrate efficacy due to the high stakes involved.
Moreover, the interpretation of P-values has been a subject of debate, with some statisticians advocating for a more nuanced understanding of their meaning. They argue that P-values should not be used as a binary decision tool ("significant" or "not significant") but rather as a continuous measure of evidence. This perspective encourages researchers to consider the size of the P-value, the size of the effect, and the precision of the estimate when interpreting results.
In recent years, there has been a push towards using alternative metrics such as Bayesian methods, which provide a different framework for statistical inference. Bayesian methods do not use P-values but instead offer a probability distribution over the parameter space, which can give a more comprehensive view of the uncertainty in the estimates.
In conclusion, a good P-value in statistics is one that is appropriate for the context of the study and the questions being asked. It is essential to consider the P-value in conjunction with other factors, such as the study design, the quality of the data, and the potential consequences of the decision based on the results. The P-value is a tool, not a definitive answer, and its interpretation should be made with care and consideration.
The P-value is a statistic that measures the strength of the evidence against the null hypothesis. It is calculated by assuming the null hypothesis is true and then determining the probability of observing a test statistic as extreme as, or more extreme than, the one calculated from the data. It is important to note that a P-value is not the probability that the null hypothesis is true or false; rather, it is a measure of how incompatible the data are with the null hypothesis.
In many scientific fields, a P-value of less than 0.05 is traditionally considered to be statistically significant. This threshold is somewhat arbitrary but has been widely adopted because it provides a balance between the risks of making a Type I error (rejecting a true null hypothesis) and a Type II error (failing to reject a false null hypothesis). A P-value below this threshold suggests that the observed data would be unlikely to occur if the null hypothesis were true, indicating strong evidence against the null hypothesis.
Conversely, a P-value greater than 0.05 is typically considered to indicate weak evidence against the null hypothesis. This does not mean that the null hypothesis is true, but rather that the data are not sufficiently strong to reject it. It is important to recognize that failing to reject the null hypothesis is not the same as proving it to be true.
However, it is crucial to understand that the choice of a P-value threshold is not a one-size-fits-all approach. Different fields may use different thresholds based on the costs of different types of errors, the variability of the data, and the potential impact of the results. For instance, in medical trials, a P-value of less than 0.01 might be required to demonstrate efficacy due to the high stakes involved.
Moreover, the interpretation of P-values has been a subject of debate, with some statisticians advocating for a more nuanced understanding of their meaning. They argue that P-values should not be used as a binary decision tool ("significant" or "not significant") but rather as a continuous measure of evidence. This perspective encourages researchers to consider the size of the P-value, the size of the effect, and the precision of the estimate when interpreting results.
In recent years, there has been a push towards using alternative metrics such as Bayesian methods, which provide a different framework for statistical inference. Bayesian methods do not use P-values but instead offer a probability distribution over the parameter space, which can give a more comprehensive view of the uncertainty in the estimates.
In conclusion, a good P-value in statistics is one that is appropriate for the context of the study and the questions being asked. It is essential to consider the P-value in conjunction with other factors, such as the study design, the quality of the data, and the potential consequences of the decision based on the results. The P-value is a tool, not a definitive answer, and its interpretation should be made with care and consideration.
2024-04-30 13:55:42
reply(1)
Helpful(1122)
Helpful
Helpful(2)
Works at the World Health Organization, Lives in Geneva, Switzerland.
A small p-value (typically -- 0.05) indicates strong evidence against the null hypothesis, so you reject the null hypothesis. A large p-value (> 0.05) indicates weak evidence against the null hypothesis, so you fail to reject the null hypothesis.
2023-06-27 09:30:32
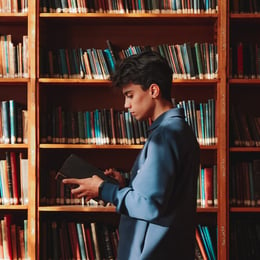
Daniel Lee
QuesHub.com delivers expert answers and knowledge to you.
A small p-value (typically -- 0.05) indicates strong evidence against the null hypothesis, so you reject the null hypothesis. A large p-value (> 0.05) indicates weak evidence against the null hypothesis, so you fail to reject the null hypothesis.