What is p value in context?
I'll answer
Earn 20 gold coins for an accepted answer.20
Earn 20 gold coins for an accepted answer.
40more
40more
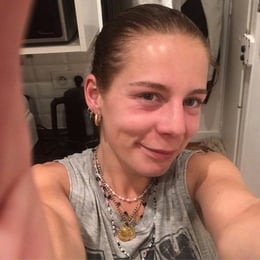
Sophia Moore
Studied at University of Oxford, Lives in Oxford, UK
As a domain expert in statistical analysis, I'm often asked about the significance of the P value in the context of hypothesis testing. The P value is a cornerstone concept in statistics that helps researchers make informed decisions about the results of their experiments and observations. It's a measure of the strength of the evidence against the null hypothesis, which is a statement of no effect or no difference.
In technical terms, a P value is the probability of obtaining an effect at least as extreme as the one in your sample data, **assuming the truth of the null hypothesis**. This means that if the null hypothesis were true, and there were truly no effect or no difference, the P value tells us how likely it is that we would observe a sample as extreme as the one we have, purely by chance.
### Importance of P Values
P values are crucial in scientific research for several reasons:
1. Decision Making: They provide a standardized way to make decisions about whether to reject the null hypothesis. A common threshold, or significance level, is 0.05, meaning if the P value is less than 0.05, the evidence is considered strong enough to reject the null hypothesis.
2. Quantifying Uncertainty: P values quantify the uncertainty associated with the results. A low P value indicates that the observed effect is unlikely to have occurred by chance alone.
3. Comparability: They allow for the comparison of results across different studies. If two studies report P values, it's possible to compare the strength of the evidence they provide.
4. Reproducibility: P values encourage researchers to replicate studies. A study with a low P value is more likely to be replicated to confirm the findings.
### Misinterpretations
Despite their utility, P values are often misunderstood and misused:
1. Certainty vs. Probability: A P value does not provide certainty that the null hypothesis is true or false; it is a statement about the probability of the observed data under the assumption that the null hypothesis is true.
2. Effect Size: A low P value does not necessarily mean a large effect size. It could be the result of a very large sample size that detects a small effect that may not be practically significant.
3. Confidence Intervals: P values are often used in conjunction with confidence intervals to provide a more complete picture of the results.
### Considerations
When interpreting P values, it's important to consider:
- Sample Size: Larger samples are more likely to produce statistically significant results, even if the effect is small.
- Multiple Comparisons: Performing many tests increases the chance of a Type I error (false positive), which can be mitigated by adjusting the P value threshold or using multiple comparison correction methods.
- Contextual Meaning: The significance of a P value should always be considered in the context of the study design, the size of the effect, and the potential impact on the field.
### Conclusion
The P value is a critical tool in statistical analysis, but it is not without its limitations. It should be interpreted carefully, with a clear understanding of what it does and does not tell us about the data. It is a measure of evidence against the null hypothesis, not a measure of the truth or falsity of a research hypothesis.
Understanding P values requires a solid grasp of statistical principles and a thoughtful approach to experimental design and data analysis. As researchers continue to refine their methods and reporting standards, the use of P values will hopefully become more nuanced and accurate, leading to better scientific inquiry and discovery.
In technical terms, a P value is the probability of obtaining an effect at least as extreme as the one in your sample data, **assuming the truth of the null hypothesis**. This means that if the null hypothesis were true, and there were truly no effect or no difference, the P value tells us how likely it is that we would observe a sample as extreme as the one we have, purely by chance.
### Importance of P Values
P values are crucial in scientific research for several reasons:
1. Decision Making: They provide a standardized way to make decisions about whether to reject the null hypothesis. A common threshold, or significance level, is 0.05, meaning if the P value is less than 0.05, the evidence is considered strong enough to reject the null hypothesis.
2. Quantifying Uncertainty: P values quantify the uncertainty associated with the results. A low P value indicates that the observed effect is unlikely to have occurred by chance alone.
3. Comparability: They allow for the comparison of results across different studies. If two studies report P values, it's possible to compare the strength of the evidence they provide.
4. Reproducibility: P values encourage researchers to replicate studies. A study with a low P value is more likely to be replicated to confirm the findings.
### Misinterpretations
Despite their utility, P values are often misunderstood and misused:
1. Certainty vs. Probability: A P value does not provide certainty that the null hypothesis is true or false; it is a statement about the probability of the observed data under the assumption that the null hypothesis is true.
2. Effect Size: A low P value does not necessarily mean a large effect size. It could be the result of a very large sample size that detects a small effect that may not be practically significant.
3. Confidence Intervals: P values are often used in conjunction with confidence intervals to provide a more complete picture of the results.
### Considerations
When interpreting P values, it's important to consider:
- Sample Size: Larger samples are more likely to produce statistically significant results, even if the effect is small.
- Multiple Comparisons: Performing many tests increases the chance of a Type I error (false positive), which can be mitigated by adjusting the P value threshold or using multiple comparison correction methods.
- Contextual Meaning: The significance of a P value should always be considered in the context of the study design, the size of the effect, and the potential impact on the field.
### Conclusion
The P value is a critical tool in statistical analysis, but it is not without its limitations. It should be interpreted carefully, with a clear understanding of what it does and does not tell us about the data. It is a measure of evidence against the null hypothesis, not a measure of the truth or falsity of a research hypothesis.
Understanding P values requires a solid grasp of statistical principles and a thoughtful approach to experimental design and data analysis. As researchers continue to refine their methods and reporting standards, the use of P values will hopefully become more nuanced and accurate, leading to better scientific inquiry and discovery.
2024-04-18 23:44:31
reply(1)
Helpful(1122)
Helpful
Helpful(2)
Works at Amazon, Lives in Seattle, WA
In technical terms, a P value is the probability of obtaining an effect at least as extreme as the one in your sample data, assuming the truth of the null hypothesis.Apr 17, 2014
2023-06-22 09:21:49

Harper Wilson
QuesHub.com delivers expert answers and knowledge to you.
In technical terms, a P value is the probability of obtaining an effect at least as extreme as the one in your sample data, assuming the truth of the null hypothesis.Apr 17, 2014