What effects p value?
I'll answer
Earn 20 gold coins for an accepted answer.20
Earn 20 gold coins for an accepted answer.
40more
40more
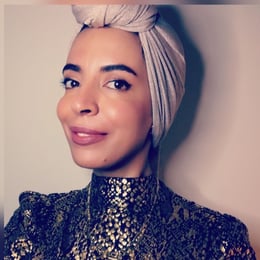
Isabella Young
Studied at the University of Amsterdam, Lives in Amsterdam, Netherlands.
As a statistical expert with a deep understanding of hypothesis testing and p-values, I can explain the factors that affect the p-value in a study. The p-value is a crucial component in statistical analysis, as it provides a measure of the strength of the evidence against the null hypothesis. It is calculated based on the observed data and the assumptions of the test. Here are several factors that can influence the p-value:
1. Sample Size: Larger sample sizes typically lead to smaller p-values for the same effect size, because the larger the sample, the more precise the estimate of the population parameter. This increased precision can make it easier to detect an effect that is statistically significant.
2. Effect Size: The magnitude of the effect being studied also influences the p-value. A larger effect size is more likely to result in a smaller p-value, indicating a stronger evidence against the null hypothesis.
3. Variability Within the Data: Greater variability or dispersion in the data can lead to a larger p-value. This is because variability can obscure the signal of the effect, making it harder to distinguish from random noise.
4. Level of Significance (Alpha): The alpha level, often set at 0.05, is the threshold for determining statistical significance. A lower alpha level will result in a larger p-value being required to reject the null hypothesis.
5. Test Statistic: The specific test statistic used can affect the p-value. Different tests have different sensitivities to effects and can yield different p-values for the same set of data.
6. Distribution Assumptions: Many statistical tests are based on certain assumptions about the distribution of the data. Violations of these assumptions can affect the p-value. For example, if a test assumes normality and the data are not normally distributed, the p-value may not be accurate.
7.
Multiple Testing: Performing multiple statistical tests on the same dataset can increase the likelihood of obtaining a significant result by chance (Type I error). This is known as the multiple comparisons problem and can be corrected using methods like the Bonferroni correction.
8.
Outliers: Extreme values or outliers can have a disproportionate influence on the p-value, particularly in small samples. They can either inflate or deflate the p-value, depending on their direction relative to the hypothesized effect.
9.
Data Quality: The quality of the data collected can greatly affect the p-value. Errors in data collection, measurement errors, or non-response bias can all lead to misleading p-values.
10.
Random Sampling: The p-value assumes that the sample is drawn randomly from the population. If the sample is not representative, the p-value may not accurately reflect the population.
It's important to note that a p-value is not the probability that the null hypothesis is true or the probability that the alternative hypothesis is true. Instead, it is the probability of observing a test statistic as extreme as, or more extreme than, the one calculated from my sample data, under the assumption that the null hypothesis is true.
Now, let's move on to the translation:
1. Sample Size: Larger sample sizes typically lead to smaller p-values for the same effect size, because the larger the sample, the more precise the estimate of the population parameter. This increased precision can make it easier to detect an effect that is statistically significant.
2. Effect Size: The magnitude of the effect being studied also influences the p-value. A larger effect size is more likely to result in a smaller p-value, indicating a stronger evidence against the null hypothesis.
3. Variability Within the Data: Greater variability or dispersion in the data can lead to a larger p-value. This is because variability can obscure the signal of the effect, making it harder to distinguish from random noise.
4. Level of Significance (Alpha): The alpha level, often set at 0.05, is the threshold for determining statistical significance. A lower alpha level will result in a larger p-value being required to reject the null hypothesis.
5. Test Statistic: The specific test statistic used can affect the p-value. Different tests have different sensitivities to effects and can yield different p-values for the same set of data.
6. Distribution Assumptions: Many statistical tests are based on certain assumptions about the distribution of the data. Violations of these assumptions can affect the p-value. For example, if a test assumes normality and the data are not normally distributed, the p-value may not be accurate.
7.
Multiple Testing: Performing multiple statistical tests on the same dataset can increase the likelihood of obtaining a significant result by chance (Type I error). This is known as the multiple comparisons problem and can be corrected using methods like the Bonferroni correction.
8.
Outliers: Extreme values or outliers can have a disproportionate influence on the p-value, particularly in small samples. They can either inflate or deflate the p-value, depending on their direction relative to the hypothesized effect.
9.
Data Quality: The quality of the data collected can greatly affect the p-value. Errors in data collection, measurement errors, or non-response bias can all lead to misleading p-values.
10.
Random Sampling: The p-value assumes that the sample is drawn randomly from the population. If the sample is not representative, the p-value may not accurately reflect the population.
It's important to note that a p-value is not the probability that the null hypothesis is true or the probability that the alternative hypothesis is true. Instead, it is the probability of observing a test statistic as extreme as, or more extreme than, the one calculated from my sample data, under the assumption that the null hypothesis is true.
Now, let's move on to the translation:
2024-04-02 07:15:30
reply(1)
Helpful(1122)
Helpful
Helpful(2)
Studied at the University of Lagos, Lives in Lagos, Nigeria.
The significance test yields a p-value that gives the likelihood of the study effect, given that the null hypothesis is true. For example, a p-value of .02 means that, assuming that the treatment has no effect, and given the sample size, an effect as large as the observed effect would be seen in only 2% of studies.
2023-06-27 09:13:55
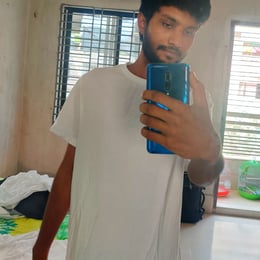
Julian Gonzales
QuesHub.com delivers expert answers and knowledge to you.
The significance test yields a p-value that gives the likelihood of the study effect, given that the null hypothesis is true. For example, a p-value of .02 means that, assuming that the treatment has no effect, and given the sample size, an effect as large as the observed effect would be seen in only 2% of studies.