What does it mean to achieve statistical significance?
I'll answer
Earn 20 gold coins for an accepted answer.20
Earn 20 gold coins for an accepted answer.
40more
40more
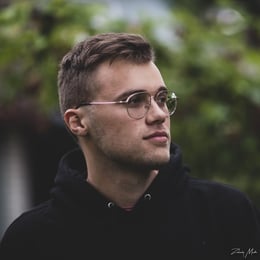
Samuel Hernandez
Works at Microsoft, Lives in Redmond, WA
As a statistical expert with a focus on data analysis and interpretation, I often encounter the concept of "statistical significance" in my work. Achieving statistical significance is a critical step in validating the results of a study or experiment. It provides a way to assess whether the observed effects or relationships are likely due to the variables being studied, rather than just random variation or noise in the data.
When we talk about statistical significance, we're referring to the probability that the observed results could have occurred by chance if there truly were no effect or relationship. In other words, it's a measure of the strength of the evidence against the null hypothesis, which typically posits that there is no effect or no difference.
### Steps to Determine Statistical Significance:
1. Formulate the Hypotheses: The first step is to clearly define the null hypothesis (H0) and the alternative hypothesis (H1). The null hypothesis usually states that there is no effect or no relationship, while the alternative hypothesis suggests there is an effect or a relationship.
2. Choose a Significance Level: This is a threshold probability, denoted by α (alpha), which is the probability of rejecting the null hypothesis when it is actually true (a false positive). Commonly used significance levels are 0.05, 0.01, and 0.001.
3. Collect and Analyze the Data: After formulating the hypotheses and choosing a significance level, the next step is to collect data and perform the appropriate statistical tests. The choice of test depends on the nature of the data and the hypotheses being tested.
4. Calculate the Test Statistic: This is a numerical value computed from the sample data that indicates the degree to which the null hypothesis is supported or contradicted by the data.
5. Determine the P-value: The P-value is the probability of obtaining results as extreme as, or more extreme than, the observed results under the assumption that the null hypothesis is true. If the P-value is less than or equal to the chosen significance level, the results are considered statistically significant.
6. Interpret the Results: If the P-value is less than the significance level, we reject the null hypothesis in favor of the alternative hypothesis. This suggests that the observed effect is statistically significant and likely not due to chance.
### Importance of Statistical Significance:
- Decision-Making: It helps researchers and practitioners make informed decisions about whether to accept or reject the null hypothesis.
- Replicability: Statistically significant results are more likely to be replicable, which is crucial for the scientific process.
- Effect Size: While statistical significance tells us if an effect is likely non-random, it does not tell us how large or important the effect is. This is where the concept of effect size comes into play.
### Limitations and Considerations:
- P-hacking: The practice of manipulating the data or the statistical analysis to achieve significance is known as P-hacking and can lead to false conclusions.
- Sample Size: A larger sample size increases the likelihood of finding statistical significance, even for very small effects.
- Statistical vs. Practical Significance: A result can be statistically significant but may not be practically significant or meaningful in a real-world context.
In conclusion, achieving statistical significance is a rigorous process that helps to establish the credibility of research findings. It is not a measure of the importance or the truth of a finding but rather an indication that the results are unlikely to be due to random chance.
When we talk about statistical significance, we're referring to the probability that the observed results could have occurred by chance if there truly were no effect or relationship. In other words, it's a measure of the strength of the evidence against the null hypothesis, which typically posits that there is no effect or no difference.
### Steps to Determine Statistical Significance:
1. Formulate the Hypotheses: The first step is to clearly define the null hypothesis (H0) and the alternative hypothesis (H1). The null hypothesis usually states that there is no effect or no relationship, while the alternative hypothesis suggests there is an effect or a relationship.
2. Choose a Significance Level: This is a threshold probability, denoted by α (alpha), which is the probability of rejecting the null hypothesis when it is actually true (a false positive). Commonly used significance levels are 0.05, 0.01, and 0.001.
3. Collect and Analyze the Data: After formulating the hypotheses and choosing a significance level, the next step is to collect data and perform the appropriate statistical tests. The choice of test depends on the nature of the data and the hypotheses being tested.
4. Calculate the Test Statistic: This is a numerical value computed from the sample data that indicates the degree to which the null hypothesis is supported or contradicted by the data.
5. Determine the P-value: The P-value is the probability of obtaining results as extreme as, or more extreme than, the observed results under the assumption that the null hypothesis is true. If the P-value is less than or equal to the chosen significance level, the results are considered statistically significant.
6. Interpret the Results: If the P-value is less than the significance level, we reject the null hypothesis in favor of the alternative hypothesis. This suggests that the observed effect is statistically significant and likely not due to chance.
### Importance of Statistical Significance:
- Decision-Making: It helps researchers and practitioners make informed decisions about whether to accept or reject the null hypothesis.
- Replicability: Statistically significant results are more likely to be replicable, which is crucial for the scientific process.
- Effect Size: While statistical significance tells us if an effect is likely non-random, it does not tell us how large or important the effect is. This is where the concept of effect size comes into play.
### Limitations and Considerations:
- P-hacking: The practice of manipulating the data or the statistical analysis to achieve significance is known as P-hacking and can lead to false conclusions.
- Sample Size: A larger sample size increases the likelihood of finding statistical significance, even for very small effects.
- Statistical vs. Practical Significance: A result can be statistically significant but may not be practically significant or meaningful in a real-world context.
In conclusion, achieving statistical significance is a rigorous process that helps to establish the credibility of research findings. It is not a measure of the importance or the truth of a finding but rather an indication that the results are unlikely to be due to random chance.
2024-04-20 01:18:35
reply(1)
Helpful(1122)
Helpful
Helpful(2)
Works at the International Monetary Fund, Lives in Washington, D.C., USA.
Statistically significant is the likelihood that a relationship between two or more variables is caused by something other than random chance. Statistical hypothesis testing is used to determine whether the result of a data set is statistically significant.
2023-06-22 08:49:36
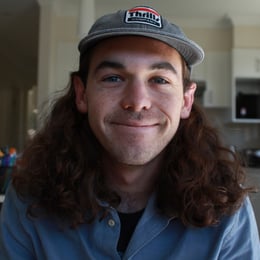
Elijah Foster
QuesHub.com delivers expert answers and knowledge to you.
Statistically significant is the likelihood that a relationship between two or more variables is caused by something other than random chance. Statistical hypothesis testing is used to determine whether the result of a data set is statistically significant.