Why do we use Anova?
I'll answer
Earn 20 gold coins for an accepted answer.20
Earn 20 gold coins for an accepted answer.
40more
40more
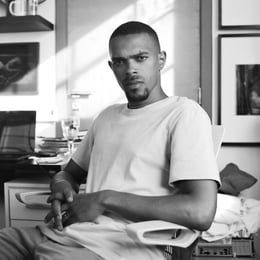
Lucas Garcia
Works at Tesla, Lives in San Francisco. Graduated from University of California, Berkeley with a degree in Mechanical Engineering.
As a statistical expert with a strong background in data analysis, I'm often asked about the utility and application of various statistical methods. One such method is Analysis of Variance, commonly abbreviated as ANOVA. This technique is a cornerstone in the field of statistics for its ability to discern significant differences between group means. Let's delve into why ANOVA is used and its significance in statistical analysis.
### Why Use ANOVA?
####
1. Testing Multiple Means
The primary reason for using ANOVA is to test for differences among three or more group means. It's a more sophisticated extension of the t-test, which is used to compare two means. When you have more than two groups, conducting multiple t-tests can be cumbersome and increase the risk of Type I errors (false positives). ANOVA provides a single test to compare all group means simultaneously.
####
2. Efficiency in Analysis
ANOVA is an efficient method for analyzing data from experiments where the independent variable is categorical, and the dependent variable is continuous. It is particularly useful when dealing with balanced designs, where all groups have an equal number of observations.
####
3. Assumption of Normality
One of the assumptions of ANOVA is that the data are normally distributed. This is important because it allows for the use of parametric statistical methods, which are generally more powerful than non-parametric methods.
####
4. Homogeneity of Variance
Another assumption is that the variances of the populations from which the samples are drawn are equal (homogeneity of variance). This assumption is critical because it underpins the validity of the ANOVA F-test.
####
5. Identifying Significant Effects
ANOVA is designed to identify significant effects or differences that cannot be attributed to random variation. It partitions the total variability in the data into components assignable to the different treatments (between-group variability) and the variability within the treatments (within-group variability).
####
6. Use in Designed Experiments
ANOVA is widely used in designed experiments, such as agricultural, industrial, and pharmaceutical research, where treatments are applied systematically, and the goal is to understand the effect of these treatments on the response variable.
#### 7.
Practical Applications
Beyond the laboratory, ANOVA has practical applications in various fields. For instance, it can be used in quality control to detect shifts in the process mean, in education to compare different teaching methods, or in business to evaluate the effectiveness of different marketing strategies.
#### 8.
Post-Hoc Analysis
When ANOVA indicates a significant difference, it is often followed up with post-hoc tests to determine which specific group means are different. This is crucial for understanding the nature of the differences found.
#### 9.
Simplicity and Interpretability
The results of an ANOVA test are straightforward to interpret. The F-ratio, which is the ratio of between-group variance to within-group variance, provides a clear measure of the effect size.
#### 10.
Statistical Power
ANOVA has good statistical power, meaning it is less likely to miss a true effect when one exists. This is particularly important in research where missing an effect can lead to incorrect conclusions.
### The Name "Analysis of Variance"
The name Analysis of Variance might seem counterintuitive at first, but it is quite fitting. The method does not directly analyze the means; instead, it analyzes the variance within the data. The logic behind this is that if the variance among the group means is significantly larger than the variance within the groups, it suggests that the different treatments or conditions are having an effect. This is the essence of how ANOVA makes inferences about the equality of means.
ANOVA is a powerful tool in the statistical toolkit, offering a robust framework for comparing group means under a variety of experimental conditions. It is a method that has stood the test of time and continues to be relevant in contemporary data analysis.
### Why Use ANOVA?
####
1. Testing Multiple Means
The primary reason for using ANOVA is to test for differences among three or more group means. It's a more sophisticated extension of the t-test, which is used to compare two means. When you have more than two groups, conducting multiple t-tests can be cumbersome and increase the risk of Type I errors (false positives). ANOVA provides a single test to compare all group means simultaneously.
####
2. Efficiency in Analysis
ANOVA is an efficient method for analyzing data from experiments where the independent variable is categorical, and the dependent variable is continuous. It is particularly useful when dealing with balanced designs, where all groups have an equal number of observations.
####
3. Assumption of Normality
One of the assumptions of ANOVA is that the data are normally distributed. This is important because it allows for the use of parametric statistical methods, which are generally more powerful than non-parametric methods.
####
4. Homogeneity of Variance
Another assumption is that the variances of the populations from which the samples are drawn are equal (homogeneity of variance). This assumption is critical because it underpins the validity of the ANOVA F-test.
####
5. Identifying Significant Effects
ANOVA is designed to identify significant effects or differences that cannot be attributed to random variation. It partitions the total variability in the data into components assignable to the different treatments (between-group variability) and the variability within the treatments (within-group variability).
####
6. Use in Designed Experiments
ANOVA is widely used in designed experiments, such as agricultural, industrial, and pharmaceutical research, where treatments are applied systematically, and the goal is to understand the effect of these treatments on the response variable.
#### 7.
Practical Applications
Beyond the laboratory, ANOVA has practical applications in various fields. For instance, it can be used in quality control to detect shifts in the process mean, in education to compare different teaching methods, or in business to evaluate the effectiveness of different marketing strategies.
#### 8.
Post-Hoc Analysis
When ANOVA indicates a significant difference, it is often followed up with post-hoc tests to determine which specific group means are different. This is crucial for understanding the nature of the differences found.
#### 9.
Simplicity and Interpretability
The results of an ANOVA test are straightforward to interpret. The F-ratio, which is the ratio of between-group variance to within-group variance, provides a clear measure of the effect size.
#### 10.
Statistical Power
ANOVA has good statistical power, meaning it is less likely to miss a true effect when one exists. This is particularly important in research where missing an effect can lead to incorrect conclusions.
### The Name "Analysis of Variance"
The name Analysis of Variance might seem counterintuitive at first, but it is quite fitting. The method does not directly analyze the means; instead, it analyzes the variance within the data. The logic behind this is that if the variance among the group means is significantly larger than the variance within the groups, it suggests that the different treatments or conditions are having an effect. This is the essence of how ANOVA makes inferences about the equality of means.
ANOVA is a powerful tool in the statistical toolkit, offering a robust framework for comparing group means under a variety of experimental conditions. It is a method that has stood the test of time and continues to be relevant in contemporary data analysis.
2024-04-21 20:20:15
reply(1)
Helpful(1122)
Helpful
Helpful(2)
Works at the International Committee of the Red Cross, Lives in Geneva, Switzerland.
The one-way analysis of variance (ANOVA) is used to determine whether there are any statistically significant differences between the means of two or more independent (unrelated) groups (although you tend to only see it used when there are a minimum of three, rather than two groups).
2023-06-21 08:49:28
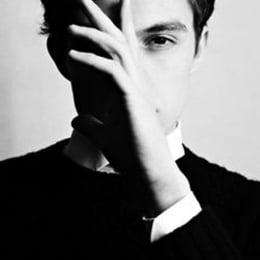
Daniel Wright
QuesHub.com delivers expert answers and knowledge to you.
The one-way analysis of variance (ANOVA) is used to determine whether there are any statistically significant differences between the means of two or more independent (unrelated) groups (although you tend to only see it used when there are a minimum of three, rather than two groups).