How does sample size increase power?
I'll answer
Earn 20 gold coins for an accepted answer.20
Earn 20 gold coins for an accepted answer.
40more
40more

Oliver Hall
Works at the International Renewable Energy Agency, Lives in Abu Dhabi, UAE.
As a statistical expert with a focus on research methodology and data analysis, I often come across the concept of sample size and its impact on the power of a statistical test. Understanding the relationship between sample size and statistical power is crucial for designing effective experiments and making informed decisions based on data.
Power in statistical testing refers to the probability that a test correctly rejects a false null hypothesis (i.e., the probability of avoiding a Type II error). It is an essential consideration when planning a study because it directly affects the likelihood of detecting an effect if there is one to be found.
### How Sample Size Affects Power
1. Definition of Power: Statistical power is the probability that a test will reject the null hypothesis when the alternative hypothesis is true. It is calculated as \(1 - \beta\), where \(\beta\) is the probability of a Type II error.
2. Role of Sample Size: The sample size (\(n\)) is a critical factor in determining the power of a test. As the sample size increases, the power of the test generally increases as well.
3. Central Limit Theorem: One of the reasons larger sample sizes increase power is the Central Limit Theorem, which states that the distribution of the sample means will approach a normal distribution as the sample size becomes large, regardless of the shape of the population distribution.
4. Standard Error: A larger sample size reduces the standard error of the estimate. The standard error is a measure of the variability of the sample mean as an estimate of the population mean. With a smaller standard error, the confidence intervals around the sample mean become narrower, making it easier to detect a true effect.
5. Test Statistic Distribution: As mentioned in the reference content, as \(n\) increases, the distribution of the test statistic becomes narrower. This narrowing effect allows for a more precise estimation of the population parameter, which in turn increases the likelihood of correctly rejecting a false null hypothesis.
6. Type I Error Rate: It's important to note that while increasing sample size can increase power, it also affects the probability of a Type I error. However, this probability is controlled by the significance level (\(\alpha\)) that is set before conducting the test and is not directly influenced by the sample size.
7.
Practical Implications: In practical terms, a higher power means that the study is more sensitive to detecting differences or effects. This is particularly important in fields where missing a real effect (a Type II error) can have serious consequences.
8.
Ethical Considerations: Increasing sample size can also have ethical implications, as it may require more participants or subjects, which could raise issues related to consent, privacy, and the use of resources.
9.
Cost and Feasibility: On the flip side, a larger sample size can increase the cost and logistical complexity of a study. Researchers must balance the need for adequate power with the practical constraints of their research design.
10.
Sample Size Determination: Before conducting a study, researchers often use power analysis to determine the appropriate sample size. This involves specifying the desired power, the significance level, the effect size one wishes to detect, and the variability expected in the data.
### Conclusion
Increasing the sample size is one of the most straightforward ways to enhance the power of a statistical test. However, it is not without trade-offs, including the potential for increased Type I error rates if not properly accounted for, ethical considerations, and practical constraints. Researchers must carefully consider these factors when planning their studies to ensure that they are both statistically robust and ethically sound.
Power in statistical testing refers to the probability that a test correctly rejects a false null hypothesis (i.e., the probability of avoiding a Type II error). It is an essential consideration when planning a study because it directly affects the likelihood of detecting an effect if there is one to be found.
### How Sample Size Affects Power
1. Definition of Power: Statistical power is the probability that a test will reject the null hypothesis when the alternative hypothesis is true. It is calculated as \(1 - \beta\), where \(\beta\) is the probability of a Type II error.
2. Role of Sample Size: The sample size (\(n\)) is a critical factor in determining the power of a test. As the sample size increases, the power of the test generally increases as well.
3. Central Limit Theorem: One of the reasons larger sample sizes increase power is the Central Limit Theorem, which states that the distribution of the sample means will approach a normal distribution as the sample size becomes large, regardless of the shape of the population distribution.
4. Standard Error: A larger sample size reduces the standard error of the estimate. The standard error is a measure of the variability of the sample mean as an estimate of the population mean. With a smaller standard error, the confidence intervals around the sample mean become narrower, making it easier to detect a true effect.
5. Test Statistic Distribution: As mentioned in the reference content, as \(n\) increases, the distribution of the test statistic becomes narrower. This narrowing effect allows for a more precise estimation of the population parameter, which in turn increases the likelihood of correctly rejecting a false null hypothesis.
6. Type I Error Rate: It's important to note that while increasing sample size can increase power, it also affects the probability of a Type I error. However, this probability is controlled by the significance level (\(\alpha\)) that is set before conducting the test and is not directly influenced by the sample size.
7.
Practical Implications: In practical terms, a higher power means that the study is more sensitive to detecting differences or effects. This is particularly important in fields where missing a real effect (a Type II error) can have serious consequences.
8.
Ethical Considerations: Increasing sample size can also have ethical implications, as it may require more participants or subjects, which could raise issues related to consent, privacy, and the use of resources.
9.
Cost and Feasibility: On the flip side, a larger sample size can increase the cost and logistical complexity of a study. Researchers must balance the need for adequate power with the practical constraints of their research design.
10.
Sample Size Determination: Before conducting a study, researchers often use power analysis to determine the appropriate sample size. This involves specifying the desired power, the significance level, the effect size one wishes to detect, and the variability expected in the data.
### Conclusion
Increasing the sample size is one of the most straightforward ways to enhance the power of a statistical test. However, it is not without trade-offs, including the potential for increased Type I error rates if not properly accounted for, ethical considerations, and practical constraints. Researchers must carefully consider these factors when planning their studies to ensure that they are both statistically robust and ethically sound.
2024-04-28 02:43:03
reply(1)
Helpful(1122)
Helpful
Helpful(2)
Works at the International Energy Agency, Lives in Paris, France.
The price of this increased power is that as -- goes up, so does the probability of a Type I error should the null hypothesis in fact be true. The sample size n. As n increases, so does the power of the significance test. This is because a larger sample size narrows the distribution of the test statistic.
2023-06-23 08:41:24
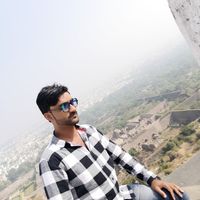
Lucas Patel
QuesHub.com delivers expert answers and knowledge to you.
The price of this increased power is that as -- goes up, so does the probability of a Type I error should the null hypothesis in fact be true. The sample size n. As n increases, so does the power of the significance test. This is because a larger sample size narrows the distribution of the test statistic.