What is the meaning of p value?
I'll answer
Earn 20 gold coins for an accepted answer.20
Earn 20 gold coins for an accepted answer.
40more
40more

Alexander Davis
Works at Apple, Lives in Cupertino, CA
As a statistical expert with a strong background in data analysis, I am well-versed in the interpretation and significance of various statistical measures, including the p-value. The p-value is a crucial concept in hypothesis testing, which is a fundamental process in statistical inference.
The p-value, or calculated probability, is a statistical measure that indicates the strength of the evidence against the null hypothesis (H0). It is the probability of obtaining results as extreme as, or more extreme than, the observed results, assuming that the null hypothesis is true. The null hypothesis is a statement about a population that is typically set up to be tested and potentially refuted by the data.
Here are several key points to understand about the p-value:
1. Definition of 'Extreme': The term 'extreme' is relative to the direction of the alternative hypothesis (H1). If H1 suggests a positive association or effect, 'extreme' would mean results in the same direction as the alternative hypothesis. Conversely, if H1 suggests a negative association, 'extreme' would mean results in the opposite direction.
2. Role in Hypothesis Testing: The p-value plays a pivotal role in determining whether to reject the null hypothesis in favor of the alternative. If the p-value is below a predetermined significance level (often denoted as α, and commonly set at 0.05), the evidence is considered statistically significant, and the null hypothesis is rejected.
3. Significance Level (α): This is the threshold p-value below which the null hypothesis is rejected. It represents the probability of a Type I error, which is the incorrect rejection of a true null hypothesis.
4. Confidence Interval: The p-value is closely related to the concept of a confidence interval. A 95% confidence interval, for example, means that if the experiment were repeated many times, we would expect the true parameter to fall within the calculated interval in 95% of the cases.
5. Interpretation: A low p-value (typically ≤ 0.05) suggests that the observed data would be unlikely if the null hypothesis were true, indicating potential evidence for an effect or association. However, it does not prove causation or establish the size or importance of the effect.
6. Misinterpretations: It's important to note that a p-value is not the probability that the null hypothesis is true or the probability that the alternative hypothesis is true. It is also not a measure of the size of the effect or the importance of the result.
7.
Multiple Testing: When conducting multiple statistical tests, the likelihood of obtaining at least one significant result by chance increases. This is known as the multiple comparisons problem and can be addressed through methods like the Bonferroni correction.
8.
Effect Size: The p-value should be considered alongside the effect size, which measures the magnitude of the difference or strength of the association in the study.
9.
Publication Bias: There is a tendency in some academic fields to only publish studies with statistically significant results, which can skew the perception of the evidence base. This is known as publication bias.
10.
Real-world Context: The p-value must always be interpreted within the context of the study design, the quality of the data, and the external validity of the findings.
In summary, the p-value is a statistical tool that helps researchers assess whether the results of their study are likely due to chance or reflect a genuine effect. It is a critical component of the scientific method but must be used and interpreted with care.
The p-value, or calculated probability, is a statistical measure that indicates the strength of the evidence against the null hypothesis (H0). It is the probability of obtaining results as extreme as, or more extreme than, the observed results, assuming that the null hypothesis is true. The null hypothesis is a statement about a population that is typically set up to be tested and potentially refuted by the data.
Here are several key points to understand about the p-value:
1. Definition of 'Extreme': The term 'extreme' is relative to the direction of the alternative hypothesis (H1). If H1 suggests a positive association or effect, 'extreme' would mean results in the same direction as the alternative hypothesis. Conversely, if H1 suggests a negative association, 'extreme' would mean results in the opposite direction.
2. Role in Hypothesis Testing: The p-value plays a pivotal role in determining whether to reject the null hypothesis in favor of the alternative. If the p-value is below a predetermined significance level (often denoted as α, and commonly set at 0.05), the evidence is considered statistically significant, and the null hypothesis is rejected.
3. Significance Level (α): This is the threshold p-value below which the null hypothesis is rejected. It represents the probability of a Type I error, which is the incorrect rejection of a true null hypothesis.
4. Confidence Interval: The p-value is closely related to the concept of a confidence interval. A 95% confidence interval, for example, means that if the experiment were repeated many times, we would expect the true parameter to fall within the calculated interval in 95% of the cases.
5. Interpretation: A low p-value (typically ≤ 0.05) suggests that the observed data would be unlikely if the null hypothesis were true, indicating potential evidence for an effect or association. However, it does not prove causation or establish the size or importance of the effect.
6. Misinterpretations: It's important to note that a p-value is not the probability that the null hypothesis is true or the probability that the alternative hypothesis is true. It is also not a measure of the size of the effect or the importance of the result.
7.
Multiple Testing: When conducting multiple statistical tests, the likelihood of obtaining at least one significant result by chance increases. This is known as the multiple comparisons problem and can be addressed through methods like the Bonferroni correction.
8.
Effect Size: The p-value should be considered alongside the effect size, which measures the magnitude of the difference or strength of the association in the study.
9.
Publication Bias: There is a tendency in some academic fields to only publish studies with statistically significant results, which can skew the perception of the evidence base. This is known as publication bias.
10.
Real-world Context: The p-value must always be interpreted within the context of the study design, the quality of the data, and the external validity of the findings.
In summary, the p-value is a statistical tool that helps researchers assess whether the results of their study are likely due to chance or reflect a genuine effect. It is a critical component of the scientific method but must be used and interpreted with care.
2024-04-05 19:51:43
reply(1)
Helpful(1122)
Helpful
Helpful(2)
Studied at the University of Tokyo, Lives in Tokyo, Japan.
The P value, or calculated probability, is the probability of finding the observed, or more extreme, results when the null hypothesis (H 0) of a study question is true -C the definition of 'extreme' depends on how the hypothesis is being tested.
2023-06-25 08:25:04
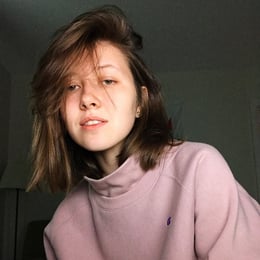
Lily Gonzales
QuesHub.com delivers expert answers and knowledge to you.
The P value, or calculated probability, is the probability of finding the observed, or more extreme, results when the null hypothesis (H 0) of a study question is true -C the definition of 'extreme' depends on how the hypothesis is being tested.