What is the usefulness of the chi square test 2024?
I'll answer
Earn 20 gold coins for an accepted answer.20
Earn 20 gold coins for an accepted answer.
40more
40more
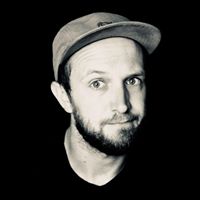
Lucas Harris
Works at Microsoft, Lives in Seattle. Graduated with honors from Carnegie Mellon University with a degree in Computer Science.
As a statistician with a keen interest in data analysis, I often find myself delving into the depths of statistical tests to uncover the stories that data can tell us. One such test that stands out for its versatility and utility is the Chi-square test. The Chi-square test is a statistical method used to determine whether there is a significant difference between the expected frequencies and the observed frequencies in a dataset. This test is particularly useful in various fields such as social sciences, market research, biology, and public health, where researchers need to compare observed data against expected outcomes under a null hypothesis.
The Chi-square test is based on the Chi-square distribution, which is a continuous probability distribution. It's a non-parametric test, meaning it doesn't assume a specific distribution of the data. This makes it a flexible tool for analyzing categorical data, where the data is divided into different categories or groups. The test compares the observed counts in each category with the counts that would be expected if the null hypothesis were true.
One of the key uses of the Chi-square test is in hypothesis testing. It can be used to test whether two categorical variables are independent of each other. For instance, researchers might use the Chi-square test to determine if there is a significant association between smoking and lung cancer, where the categories are 'smoker' or 'non-smoker' and 'having lung cancer' or 'not having lung cancer'.
Another important application is in goodness-of-fit tests. Here, the Chi-square test can be used to evaluate how well a set of observed data matches a theoretical distribution. For example, a researcher might want to check if the distribution of a certain trait in a population follows a normal distribution.
The test is also employed in contingency table analysis, where it can reveal relationships between two variables. For example, it can be used to analyze survey data to see if there is a significant difference in preferences for a product based on gender or age groups.
Furthermore, the Chi-square test is used in test of homogeneity, which is to determine if several groups come from the same population or if they differ significantly. This can be particularly useful in quality control, where manufacturers might want to ensure that products from different batches are of consistent quality.
One of the strengths of the Chi-square test is its robustness. It can handle large datasets and is relatively simple to perform, making it accessible to researchers who may not have a deep background in statistics. Additionally, the Chi-square test is scalable, meaning it can be applied to datasets of varying sizes without losing its effectiveness.
However, it's important to note that the Chi-square test has its limitations. It requires a sufficiently large sample size to ensure the validity of the results. If the expected frequencies in any of the cells of the contingency table are too low, the test may not be reliable. In such cases, alternative methods like Fisher's exact test might be more appropriate.
In conclusion, the Chi-square test is a powerful statistical tool for analyzing categorical data. It provides a way to test hypotheses, evaluate the fit of data to a theoretical model, and explore relationships between variables. Its wide range of applications and robustness make it a valuable asset in the field of statistics.
The Chi-square test is based on the Chi-square distribution, which is a continuous probability distribution. It's a non-parametric test, meaning it doesn't assume a specific distribution of the data. This makes it a flexible tool for analyzing categorical data, where the data is divided into different categories or groups. The test compares the observed counts in each category with the counts that would be expected if the null hypothesis were true.
One of the key uses of the Chi-square test is in hypothesis testing. It can be used to test whether two categorical variables are independent of each other. For instance, researchers might use the Chi-square test to determine if there is a significant association between smoking and lung cancer, where the categories are 'smoker' or 'non-smoker' and 'having lung cancer' or 'not having lung cancer'.
Another important application is in goodness-of-fit tests. Here, the Chi-square test can be used to evaluate how well a set of observed data matches a theoretical distribution. For example, a researcher might want to check if the distribution of a certain trait in a population follows a normal distribution.
The test is also employed in contingency table analysis, where it can reveal relationships between two variables. For example, it can be used to analyze survey data to see if there is a significant difference in preferences for a product based on gender or age groups.
Furthermore, the Chi-square test is used in test of homogeneity, which is to determine if several groups come from the same population or if they differ significantly. This can be particularly useful in quality control, where manufacturers might want to ensure that products from different batches are of consistent quality.
One of the strengths of the Chi-square test is its robustness. It can handle large datasets and is relatively simple to perform, making it accessible to researchers who may not have a deep background in statistics. Additionally, the Chi-square test is scalable, meaning it can be applied to datasets of varying sizes without losing its effectiveness.
However, it's important to note that the Chi-square test has its limitations. It requires a sufficiently large sample size to ensure the validity of the results. If the expected frequencies in any of the cells of the contingency table are too low, the test may not be reliable. In such cases, alternative methods like Fisher's exact test might be more appropriate.
In conclusion, the Chi-square test is a powerful statistical tool for analyzing categorical data. It provides a way to test hypotheses, evaluate the fit of data to a theoretical model, and explore relationships between variables. Its wide range of applications and robustness make it a valuable asset in the field of statistics.
2024-06-01 11:50:23
reply(1)
Helpful(1122)
Helpful
Helpful(2)
Studied at the University of Johannesburg, Lives in Johannesburg, South Africa.
Chi-square is a statistical test commonly used to compare observed data with data we would expect to obtain according to a specific hypothesis.
2023-06-18 08:25:01

Harper Martinez
QuesHub.com delivers expert answers and knowledge to you.
Chi-square is a statistical test commonly used to compare observed data with data we would expect to obtain according to a specific hypothesis.