What does a Type 1 error mean?
I'll answer
Earn 20 gold coins for an accepted answer.20
Earn 20 gold coins for an accepted answer.
40more
40more
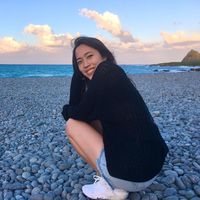
Isabella Gonzales
Studied at the University of Johannesburg, Lives in Johannesburg, South Africa.
In statistical hypothesis testing, a Type I error is a fundamental concept that is crucial for understanding the risks and implications of drawing conclusions from data. As a field expert in statistical analysis, I would like to provide a comprehensive explanation of what a Type I error means, its implications, and how it is distinguished from other types of errors in hypothesis testing.
Step 1: Understanding Hypothesis Testing
Hypothesis testing is a statistical method used to make decisions or draw conclusions about a population based on a sample of data. It involves the formulation of two competing statements about the population parameter, known as the null hypothesis (H0) and the alternative hypothesis (H1 or Ha). The null hypothesis typically represents a status quo or a claim that is being tested, while the alternative hypothesis represents the opposite of the null hypothesis or a research hypothesis that the researcher is interested in proving.
Step 2: Defining Type I Error
A Type I error occurs when the null hypothesis is incorrectly rejected. This means that the test concludes that there is a significant effect or difference when, in reality, there is none. It is also referred to as a "false positive" because it is akin to raising a false alarm. The probability of making a Type I error is denoted by the Greek letter alpha (α), which is also the significance level of the test. For example, if a test is conducted at a 5% significance level, there is a 5% chance of committing a Type I error if the null hypothesis is true.
Step 3: Consequences of Type I Error
The consequences of a Type I error can be significant, depending on the context of the study. In scientific research, it can lead to the publication of false findings. In a clinical trial, it might result in the approval of an ineffective treatment. In quality control, it could mean that a product that meets the required standards is mistakenly deemed defective.
**Step 4: Balancing Type I and Type II Errors**
It is important to note that the probability of making a Type I error is inversely related to the probability of making a Type II error (β), which is the probability of failing to reject a false null hypothesis (a "false negative"). There is often a trade-off between these two types of errors. Reducing the chance of a Type I error (making the test more conservative) increases the chance of a Type II error, and vice versa.
Step 5: Power of the Test
The power of a test is the probability that it will correctly reject a false null hypothesis. It is calculated as 1 - β. A higher power means a lower chance of a Type II error. The power of a test is influenced by several factors, including the sample size, the effect size, and the significance level.
Step 6: Minimizing Type I Errors
To minimize the risk of a Type I error, researchers can take several steps:
1. Set an appropriate significance level that balances the need for evidence against the risk of a false positive.
2. Use a sufficiently large sample size to increase the test's power.
3. Employ rigorous research methods to ensure the validity of the data and the test's assumptions.
Step 7: Practical Considerations
In practice, researchers must weigh the costs of Type I and Type II errors. For instance, in medical testing, the cost of a false positive (a healthy person being incorrectly diagnosed with a disease) might be less severe than the cost of a false negative (a sick person being missed). Therefore, the significance level might be set lower to reduce the risk of a false negative.
In conclusion, a Type I error is a critical concept in statistical hypothesis testing that requires a careful balance between the risks of false positives and false negatives. Understanding the implications of these errors and how to manage them is essential for drawing accurate and reliable conclusions from data.
Step 1: Understanding Hypothesis Testing
Hypothesis testing is a statistical method used to make decisions or draw conclusions about a population based on a sample of data. It involves the formulation of two competing statements about the population parameter, known as the null hypothesis (H0) and the alternative hypothesis (H1 or Ha). The null hypothesis typically represents a status quo or a claim that is being tested, while the alternative hypothesis represents the opposite of the null hypothesis or a research hypothesis that the researcher is interested in proving.
Step 2: Defining Type I Error
A Type I error occurs when the null hypothesis is incorrectly rejected. This means that the test concludes that there is a significant effect or difference when, in reality, there is none. It is also referred to as a "false positive" because it is akin to raising a false alarm. The probability of making a Type I error is denoted by the Greek letter alpha (α), which is also the significance level of the test. For example, if a test is conducted at a 5% significance level, there is a 5% chance of committing a Type I error if the null hypothesis is true.
Step 3: Consequences of Type I Error
The consequences of a Type I error can be significant, depending on the context of the study. In scientific research, it can lead to the publication of false findings. In a clinical trial, it might result in the approval of an ineffective treatment. In quality control, it could mean that a product that meets the required standards is mistakenly deemed defective.
**Step 4: Balancing Type I and Type II Errors**
It is important to note that the probability of making a Type I error is inversely related to the probability of making a Type II error (β), which is the probability of failing to reject a false null hypothesis (a "false negative"). There is often a trade-off between these two types of errors. Reducing the chance of a Type I error (making the test more conservative) increases the chance of a Type II error, and vice versa.
Step 5: Power of the Test
The power of a test is the probability that it will correctly reject a false null hypothesis. It is calculated as 1 - β. A higher power means a lower chance of a Type II error. The power of a test is influenced by several factors, including the sample size, the effect size, and the significance level.
Step 6: Minimizing Type I Errors
To minimize the risk of a Type I error, researchers can take several steps:
1. Set an appropriate significance level that balances the need for evidence against the risk of a false positive.
2. Use a sufficiently large sample size to increase the test's power.
3. Employ rigorous research methods to ensure the validity of the data and the test's assumptions.
Step 7: Practical Considerations
In practice, researchers must weigh the costs of Type I and Type II errors. For instance, in medical testing, the cost of a false positive (a healthy person being incorrectly diagnosed with a disease) might be less severe than the cost of a false negative (a sick person being missed). Therefore, the significance level might be set lower to reduce the risk of a false negative.
In conclusion, a Type I error is a critical concept in statistical hypothesis testing that requires a careful balance between the risks of false positives and false negatives. Understanding the implications of these errors and how to manage them is essential for drawing accurate and reliable conclusions from data.
2024-04-22 08:31:28
reply(1)
Helpful(1122)
Helpful
Helpful(2)
Works at the International Telecommunication Union, Lives in Geneva, Switzerland.
In statistical hypothesis testing, a type I error is the rejection of a true null hypothesis (also known as a "false positive" finding), while a type II error is retaining a false null hypothesis (also known as a "false negative" finding).
2023-06-27 08:24:55

Charlotte Scott
QuesHub.com delivers expert answers and knowledge to you.
In statistical hypothesis testing, a type I error is the rejection of a true null hypothesis (also known as a "false positive" finding), while a type II error is retaining a false null hypothesis (also known as a "false negative" finding).