What is the meaning of 95 confidence interval?
I'll answer
Earn 20 gold coins for an accepted answer.20
Earn 20 gold coins for an accepted answer.
40more
40more
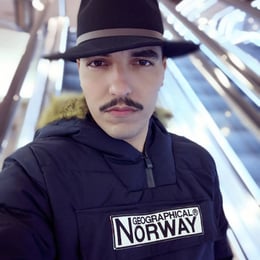
Ethan Carter
Works at Microsoft, Lives in Redmond, WA
As a subject matter expert in statistics, I'm often asked about the concept of a "confidence interval." It's a fundamental concept in inferential statistics that is used to estimate a population parameter based on sample data. Let's delve into the meaning of a 95% confidence interval.
Firstly, it's important to understand that a confidence interval is not a range within which an individual data point from the population is expected to fall. Instead, it's a range that we can be confident will contain the true population parameter, such as the mean, proportion, or difference between groups. This confidence level is expressed as a percentage, and in the case of a 95% confidence interval, it means that if we were to take many samples from the population and calculate the confidence interval for each, then theoretically, 95% of those intervals would contain the true population parameter.
The statement "A 95% confidence interval has a 0.95 probability of containing the population mean" is a common way to express this concept. However, it's crucial to clarify that it's not the interval itself that has the probability, but rather the method of calculating the interval. The interval is either correct or incorrect in containing the parameter; it's the process that has the probabilistic nature.
When we say "95% of the population distribution is contained in the confidence interval," we might be misinterpreting the concept. It's not the entire population distribution that fits within the interval, but rather the parameter estimate (like the mean) that we are confident about. The interval does not encompass 95% of the individual data points in the population, but rather it's a range around the sample mean that should capture the true population mean 95% of the time when the sampling process is repeated.
To calculate a confidence interval, statisticians use a formula that includes the sample statistic (like the sample mean), the standard error of the statistic, and the critical value from a distribution (often the normal or t-distribution) that corresponds to the desired confidence level. The width of the confidence interval is influenced by several factors:
1. Sample Size: Larger samples generally lead to narrower intervals because they provide more information about the population.
2. Variability in the Data: Greater variability (as measured by the standard deviation) leads to wider intervals.
3. Confidence Level: Higher confidence levels (e.g., 99% vs. 95%) result in wider intervals to compensate for the increased certainty.
4. Shape of the Distribution: If the data are not normally distributed, adjustments may need to be made, such as using a t-distribution instead of a z-distribution.
It's also worth noting that a confidence interval does not provide a "range of possible values" for the parameter in the same way that a range of observed data might. It's a range that is constructed to be likely to contain the parameter based on the data and the chosen confidence level. It does not mean that there is a 95% chance that the parameter is within that interval; rather, if we were to conduct the same study many times, 95% of the calculated intervals would contain the true parameter.
In conclusion, a 95% confidence interval is a statistical tool that provides an estimated range for an unknown population parameter with a certain level of confidence. It's a powerful tool for researchers and analysts, but it's also one that requires a nuanced understanding to be used and interpreted correctly.
Firstly, it's important to understand that a confidence interval is not a range within which an individual data point from the population is expected to fall. Instead, it's a range that we can be confident will contain the true population parameter, such as the mean, proportion, or difference between groups. This confidence level is expressed as a percentage, and in the case of a 95% confidence interval, it means that if we were to take many samples from the population and calculate the confidence interval for each, then theoretically, 95% of those intervals would contain the true population parameter.
The statement "A 95% confidence interval has a 0.95 probability of containing the population mean" is a common way to express this concept. However, it's crucial to clarify that it's not the interval itself that has the probability, but rather the method of calculating the interval. The interval is either correct or incorrect in containing the parameter; it's the process that has the probabilistic nature.
When we say "95% of the population distribution is contained in the confidence interval," we might be misinterpreting the concept. It's not the entire population distribution that fits within the interval, but rather the parameter estimate (like the mean) that we are confident about. The interval does not encompass 95% of the individual data points in the population, but rather it's a range around the sample mean that should capture the true population mean 95% of the time when the sampling process is repeated.
To calculate a confidence interval, statisticians use a formula that includes the sample statistic (like the sample mean), the standard error of the statistic, and the critical value from a distribution (often the normal or t-distribution) that corresponds to the desired confidence level. The width of the confidence interval is influenced by several factors:
1. Sample Size: Larger samples generally lead to narrower intervals because they provide more information about the population.
2. Variability in the Data: Greater variability (as measured by the standard deviation) leads to wider intervals.
3. Confidence Level: Higher confidence levels (e.g., 99% vs. 95%) result in wider intervals to compensate for the increased certainty.
4. Shape of the Distribution: If the data are not normally distributed, adjustments may need to be made, such as using a t-distribution instead of a z-distribution.
It's also worth noting that a confidence interval does not provide a "range of possible values" for the parameter in the same way that a range of observed data might. It's a range that is constructed to be likely to contain the parameter based on the data and the chosen confidence level. It does not mean that there is a 95% chance that the parameter is within that interval; rather, if we were to conduct the same study many times, 95% of the calculated intervals would contain the true parameter.
In conclusion, a 95% confidence interval is a statistical tool that provides an estimated range for an unknown population parameter with a certain level of confidence. It's a powerful tool for researchers and analysts, but it's also one that requires a nuanced understanding to be used and interpreted correctly.
2024-04-06 16:36:31
reply(1)
Helpful(1122)
Helpful
Helpful(2)
Studied at the University of Zurich, Lives in Zurich, Switzerland.
If repeated samples were taken and the 95% confidence interval was computed for each sample, 95% of the intervals would contain the population mean. A 95% confidence interval has a 0.95 probability of containing the population mean. 95% of the population distribution is contained in the confidence interval.
2023-06-19 08:24:55
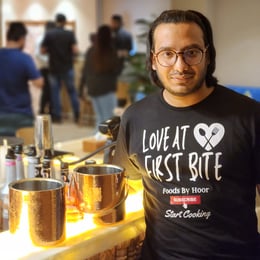
Julian Carter
QuesHub.com delivers expert answers and knowledge to you.
If repeated samples were taken and the 95% confidence interval was computed for each sample, 95% of the intervals would contain the population mean. A 95% confidence interval has a 0.95 probability of containing the population mean. 95% of the population distribution is contained in the confidence interval.