What does the effect size indicate?
I'll answer
Earn 20 gold coins for an accepted answer.20
Earn 20 gold coins for an accepted answer.
40more
40more
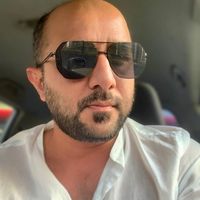
Ethan Campbell
Works at the International Committee of the Red Cross, Lives in Geneva, Switzerland.
As a domain expert in statistical analysis, I'd like to shed some light on the concept of effect size. Effect size is a crucial metric in the field of statistics and research methodology. It provides an indication of the magnitude of a phenomenon or the strength of the relationship between variables under study. Unlike significance tests, which tell us whether an effect is likely to be real or due to chance, effect sizes give us an idea of how big the effect is.
### Understanding Effect Size
Effect size is a standardized measure that can be calculated from a variety of statistical outputs. It is a numerical index that gives us a sense of how large the effect is in practical terms. This is important because it allows researchers to quantify the importance of their findings beyond just determining if they are statistically significant.
### Types of Effect Sizes
There are several types of effect sizes, each suited for different types of data and research designs:
1. **Standardized Mean Difference (e.g., Cohen's d):** This is one of the most common effect sizes used in experimental and quasi-experimental research. It expresses the mean difference between two groups in standard deviation units. Cohen's d is particularly useful for comparing the means of two groups when the sample sizes are unequal.
2. **Correlation Coefficient (e.g., Pearson's r):** In the context of correlational studies, the effect size can be the strength and direction of the linear relationship between two continuous variables.
3. Eta Squared (η²): This is used in ANOVA and is a measure of the proportion of total variance in the dependent variable that is accounted for by the independent variable.
4. Partial Eta Squared: Similar to eta squared but used in the context of multiple regression analysis to account for the unique contribution of a predictor variable.
5. Odds Ratio: Commonly used in logistic regression, it indicates the ratio of the odds of an event occurring in one group to the odds of it occurring in another.
### Interpreting Effect Sizes
Effect sizes are often interpreted in the context of small, medium, and large categories. Cohen's criteria are widely used for this purpose, where:
- Small effect size (d = 0.2): Indicates a small but noticeable effect.
- Medium effect size (d = 0.5): Indicates a moderate effect.
- Large effect size (d = 0.8): Indicates a large and substantial effect.
### Importance of Effect Size
1. Practical Significance: Effect size helps to determine the practical significance of the findings, which is essential for real-world applications.
2. Meta-Analysis: It is crucial for combining results from different studies in a meta-analysis.
3. Research Planning: Knowing the expected effect size can help in planning future studies by determining the required sample size.
4. Comparison Across Studies: It allows for comparison of effects across different studies, even if they use different measures or have different designs.
### Limitations
While effect size is a powerful tool, it also has limitations:
1. Context Dependence: The interpretation of what constitutes a small, medium, or large effect can vary depending on the context and the field of study.
2. Statistical vs. Practical Significance: A large effect size does not necessarily mean practical significance, and vice versa.
3. Publication Bias: Studies with larger effect sizes may be more likely to be published, which can skew the perception of what is typical in a field.
### Conclusion
Effect size is a critical concept in statistical analysis that complements significance testing by providing a measure of the magnitude of an effect. It is essential for understanding the real-world implications of research findings and for making informed decisions based on data.
### Understanding Effect Size
Effect size is a standardized measure that can be calculated from a variety of statistical outputs. It is a numerical index that gives us a sense of how large the effect is in practical terms. This is important because it allows researchers to quantify the importance of their findings beyond just determining if they are statistically significant.
### Types of Effect Sizes
There are several types of effect sizes, each suited for different types of data and research designs:
1. **Standardized Mean Difference (e.g., Cohen's d):** This is one of the most common effect sizes used in experimental and quasi-experimental research. It expresses the mean difference between two groups in standard deviation units. Cohen's d is particularly useful for comparing the means of two groups when the sample sizes are unequal.
2. **Correlation Coefficient (e.g., Pearson's r):** In the context of correlational studies, the effect size can be the strength and direction of the linear relationship between two continuous variables.
3. Eta Squared (η²): This is used in ANOVA and is a measure of the proportion of total variance in the dependent variable that is accounted for by the independent variable.
4. Partial Eta Squared: Similar to eta squared but used in the context of multiple regression analysis to account for the unique contribution of a predictor variable.
5. Odds Ratio: Commonly used in logistic regression, it indicates the ratio of the odds of an event occurring in one group to the odds of it occurring in another.
### Interpreting Effect Sizes
Effect sizes are often interpreted in the context of small, medium, and large categories. Cohen's criteria are widely used for this purpose, where:
- Small effect size (d = 0.2): Indicates a small but noticeable effect.
- Medium effect size (d = 0.5): Indicates a moderate effect.
- Large effect size (d = 0.8): Indicates a large and substantial effect.
### Importance of Effect Size
1. Practical Significance: Effect size helps to determine the practical significance of the findings, which is essential for real-world applications.
2. Meta-Analysis: It is crucial for combining results from different studies in a meta-analysis.
3. Research Planning: Knowing the expected effect size can help in planning future studies by determining the required sample size.
4. Comparison Across Studies: It allows for comparison of effects across different studies, even if they use different measures or have different designs.
### Limitations
While effect size is a powerful tool, it also has limitations:
1. Context Dependence: The interpretation of what constitutes a small, medium, or large effect can vary depending on the context and the field of study.
2. Statistical vs. Practical Significance: A large effect size does not necessarily mean practical significance, and vice versa.
3. Publication Bias: Studies with larger effect sizes may be more likely to be published, which can skew the perception of what is typical in a field.
### Conclusion
Effect size is a critical concept in statistical analysis that complements significance testing by providing a measure of the magnitude of an effect. It is essential for understanding the real-world implications of research findings and for making informed decisions based on data.
2024-04-07 09:24:26
reply(1)
Helpful(1122)
Helpful
Helpful(2)
Works at the International Air Transport Association, Lives in Montreal, Canada.
Effect size is a standard measure that can be calculated from any number of statistical outputs. One type of effect size, the standardized mean effect, expresses the mean difference between two groups in standard deviation units. Typically, you'll see this reported as Cohen's d, or simply referred to as --d.--
2023-06-24 08:24:54

Sophia Harris
QuesHub.com delivers expert answers and knowledge to you.
Effect size is a standard measure that can be calculated from any number of statistical outputs. One type of effect size, the standardized mean effect, expresses the mean difference between two groups in standard deviation units. Typically, you'll see this reported as Cohen's d, or simply referred to as --d.--