What does the effect size tell us?
I'll answer
Earn 20 gold coins for an accepted answer.20
Earn 20 gold coins for an accepted answer.
40more
40more
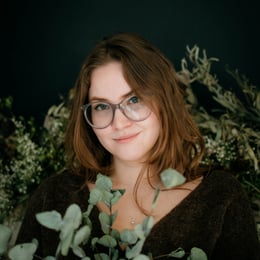
Olivia Mitchell
Studied at Princeton University, Lives in Princeton, NJ
As a statistical expert with a deep understanding of research methodologies and data analysis, I'm often asked about the importance of effect size in the context of experimental studies. The concept of effect size is crucial for interpreting the results of a statistical test and understanding the practical significance of the findings, beyond the mere statistical significance.
Effect size is essentially a measure of the strength or magnitude of the effect that an independent variable has on a dependent variable. It provides an estimate of how large the difference is between two or more groups or conditions. It's important to note that effect size is not a measure of statistical significance; rather, it complements statistical significance by addressing the question of how meaningful the observed effect is in a real-world context.
There are several types of effect sizes, each with its own method of calculation and interpretation:
1. Standardized Effect Size: This is a way to quantify the magnitude of the effect in a way that is independent of the sample size. Common standardized effect sizes include Cohen's d, Hedges' g, and Glass's Δ. These effect sizes are particularly useful when comparing the size of effects across different studies or when the original data are not available.
2. Unstandardized Effect Size: This type of effect size is expressed in the original units of measurement. For example, if you're looking at the effect of a teaching method on test scores, an unstandardized effect size might be the difference in average test scores between two groups of students.
3. Proportional Reduction in Error (PRE): This effect size is used in the context of models and represents the proportion by which the prediction error is reduced when a predictor variable is included in the model.
4. Risk Ratios and Odds Ratios: These are commonly used in medical and epidemiological studies to express the effect size in terms of the probability of an event occurring in different groups.
Understanding effect size is important for several reasons:
- Practical Significance: Statistical significance alone does not tell us whether the observed effect is large enough to be meaningful in a real-world context. An effect may be statistically significant but trivial in size, which is where effect size comes in to provide a more complete picture.
- Sample Size Considerations: Larger samples are more likely to detect statistically significant effects, even if those effects are small. Effect size helps to interpret the relevance of findings regardless of the sample size.
- Meta-Analysis: Effect sizes are essential for meta-analyses, which are used to combine the results of multiple studies. They allow for a quantitative synthesis of findings across studies that may have used different measures or sample sizes.
- Research Planning: Knowing the expected effect size can help researchers plan their studies more effectively. It can inform decisions about sample size, power, and the resources needed to detect an effect if it exists.
- Communication of Results: When reporting research findings, including the effect size can help other researchers and practitioners understand the magnitude of the findings and their potential impact.
Now, let's move on to the translation of the above explanation into Chinese.
Effect size is essentially a measure of the strength or magnitude of the effect that an independent variable has on a dependent variable. It provides an estimate of how large the difference is between two or more groups or conditions. It's important to note that effect size is not a measure of statistical significance; rather, it complements statistical significance by addressing the question of how meaningful the observed effect is in a real-world context.
There are several types of effect sizes, each with its own method of calculation and interpretation:
1. Standardized Effect Size: This is a way to quantify the magnitude of the effect in a way that is independent of the sample size. Common standardized effect sizes include Cohen's d, Hedges' g, and Glass's Δ. These effect sizes are particularly useful when comparing the size of effects across different studies or when the original data are not available.
2. Unstandardized Effect Size: This type of effect size is expressed in the original units of measurement. For example, if you're looking at the effect of a teaching method on test scores, an unstandardized effect size might be the difference in average test scores between two groups of students.
3. Proportional Reduction in Error (PRE): This effect size is used in the context of models and represents the proportion by which the prediction error is reduced when a predictor variable is included in the model.
4. Risk Ratios and Odds Ratios: These are commonly used in medical and epidemiological studies to express the effect size in terms of the probability of an event occurring in different groups.
Understanding effect size is important for several reasons:
- Practical Significance: Statistical significance alone does not tell us whether the observed effect is large enough to be meaningful in a real-world context. An effect may be statistically significant but trivial in size, which is where effect size comes in to provide a more complete picture.
- Sample Size Considerations: Larger samples are more likely to detect statistically significant effects, even if those effects are small. Effect size helps to interpret the relevance of findings regardless of the sample size.
- Meta-Analysis: Effect sizes are essential for meta-analyses, which are used to combine the results of multiple studies. They allow for a quantitative synthesis of findings across studies that may have used different measures or sample sizes.
- Research Planning: Knowing the expected effect size can help researchers plan their studies more effectively. It can inform decisions about sample size, power, and the resources needed to detect an effect if it exists.
- Communication of Results: When reporting research findings, including the effect size can help other researchers and practitioners understand the magnitude of the findings and their potential impact.
Now, let's move on to the translation of the above explanation into Chinese.
2024-04-28 20:38:59
reply(1)
Helpful(1122)
Helpful
Helpful(2)
Works at the International Criminal Court, Lives in The Hague, Netherlands.
The simple definition of effect size is the magnitude, or size, of an effect. Statistical significance (e.g., p < .05) tells us there was a difference between two groups or more based on some treatment or sorting variable.
2023-06-23 08:24:51
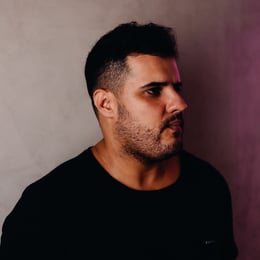
Dominic Young
QuesHub.com delivers expert answers and knowledge to you.
The simple definition of effect size is the magnitude, or size, of an effect. Statistical significance (e.g., p < .05) tells us there was a difference between two groups or more based on some treatment or sorting variable.