What does significance testing mean?
I'll answer
Earn 20 gold coins for an accepted answer.20
Earn 20 gold coins for an accepted answer.
40more
40more

Samuel Hernandez
Works at Intel, Lives in Santa Clara, CA
As a statistical expert with a deep understanding of significance testing, I'd like to elaborate on what it means and how it's applied in the field of statistics.
Significance testing is a cornerstone of inferential statistics, which is the process of drawing conclusions about a population based on a sample. It involves the use of statistical tests to determine whether the results of a study are statistically significant, meaning they are unlikely to have occurred by chance alone.
### Purpose of Significance Testing
The primary purpose of significance testing is to provide evidence against the null hypothesis (H0), which typically represents a default or status quo position. By challenging the null hypothesis, researchers can determine if there is sufficient evidence to support an alternative hypothesis (H1), which represents a claim of interest.
### The Null Hypothesis (H0)
The null hypothesis is a statement of no effect or no difference. It is a hypothesis that assumes there is no significant relationship between the variables being studied or that the results are due to random chance. For example, if a pharmaceutical company is testing a new drug, the null hypothesis might be that the drug has no effect on the condition it is intended to treat.
### The Alternative Hypothesis (H1)
The alternative hypothesis, on the other hand, is what the researcher is actually interested in proving. It is a statement that there is an effect or a difference. In the drug example, the alternative hypothesis would be that the drug does have an effect on the condition.
### Significance Level (α)
The significance level, denoted by α (alpha), is a threshold that determines when we reject the null hypothesis. It is the probability of rejecting the null hypothesis when it is actually true, also known as a Type I error. Commonly used significance levels are 0.05, 0.01, and 0.001.
### P-value
The P-value is a statistic that measures the strength of the evidence against the null hypothesis. It is the probability of observing a test statistic as extreme as, or more extreme than, the one calculated from the data, assuming the null hypothesis is true. If the P-value is less than or equal to the significance level, the result is considered statistically significant, and the null hypothesis is rejected in favor of the alternative hypothesis.
### Types of Significance Tests
There are various types of significance tests, including:
1. t-test: Used to compare the means of two groups.
2. ANOVA (Analysis of Variance): Used when comparing the means of three or more groups.
3. Chi-square test: Used to determine if there is a significant association between two categorical variables.
4. Correlation tests: Used to measure the strength and direction of the relationship between two quantitative variables.
### Steps in Significance Testing
1. **State the null and alternative hypotheses.**
2. Choose a significance level (α).
3. Collect and summarize the data.
4. Select an appropriate statistical test.
5. **Calculate the test statistic and the P-value.**
6. **Make a decision to reject or fail to reject the null hypothesis based on the P-value and the significance level.**
### Interpretation of Results
The results of a significance test should be interpreted with caution. A statistically significant result does not necessarily imply a large or practically significant effect. It simply means that the observed effect is unlikely to be due to chance.
### Conclusion
Significance testing is a powerful tool in statistical analysis, but it is not without its limitations. It is crucial to consider the context, the design of the study, and the assumptions underlying the statistical test when interpreting the results. Moreover, the significance level should be chosen before collecting the data to prevent bias.
Significance testing is not just about crunching numbers; it's about making informed decisions based on evidence. It's a method that helps scientists, researchers, and statisticians to distinguish between real effects and random variations in their data.
Significance testing is a cornerstone of inferential statistics, which is the process of drawing conclusions about a population based on a sample. It involves the use of statistical tests to determine whether the results of a study are statistically significant, meaning they are unlikely to have occurred by chance alone.
### Purpose of Significance Testing
The primary purpose of significance testing is to provide evidence against the null hypothesis (H0), which typically represents a default or status quo position. By challenging the null hypothesis, researchers can determine if there is sufficient evidence to support an alternative hypothesis (H1), which represents a claim of interest.
### The Null Hypothesis (H0)
The null hypothesis is a statement of no effect or no difference. It is a hypothesis that assumes there is no significant relationship between the variables being studied or that the results are due to random chance. For example, if a pharmaceutical company is testing a new drug, the null hypothesis might be that the drug has no effect on the condition it is intended to treat.
### The Alternative Hypothesis (H1)
The alternative hypothesis, on the other hand, is what the researcher is actually interested in proving. It is a statement that there is an effect or a difference. In the drug example, the alternative hypothesis would be that the drug does have an effect on the condition.
### Significance Level (α)
The significance level, denoted by α (alpha), is a threshold that determines when we reject the null hypothesis. It is the probability of rejecting the null hypothesis when it is actually true, also known as a Type I error. Commonly used significance levels are 0.05, 0.01, and 0.001.
### P-value
The P-value is a statistic that measures the strength of the evidence against the null hypothesis. It is the probability of observing a test statistic as extreme as, or more extreme than, the one calculated from the data, assuming the null hypothesis is true. If the P-value is less than or equal to the significance level, the result is considered statistically significant, and the null hypothesis is rejected in favor of the alternative hypothesis.
### Types of Significance Tests
There are various types of significance tests, including:
1. t-test: Used to compare the means of two groups.
2. ANOVA (Analysis of Variance): Used when comparing the means of three or more groups.
3. Chi-square test: Used to determine if there is a significant association between two categorical variables.
4. Correlation tests: Used to measure the strength and direction of the relationship between two quantitative variables.
### Steps in Significance Testing
1. **State the null and alternative hypotheses.**
2. Choose a significance level (α).
3. Collect and summarize the data.
4. Select an appropriate statistical test.
5. **Calculate the test statistic and the P-value.**
6. **Make a decision to reject or fail to reject the null hypothesis based on the P-value and the significance level.**
### Interpretation of Results
The results of a significance test should be interpreted with caution. A statistically significant result does not necessarily imply a large or practically significant effect. It simply means that the observed effect is unlikely to be due to chance.
### Conclusion
Significance testing is a powerful tool in statistical analysis, but it is not without its limitations. It is crucial to consider the context, the design of the study, and the assumptions underlying the statistical test when interpreting the results. Moreover, the significance level should be chosen before collecting the data to prevent bias.
Significance testing is not just about crunching numbers; it's about making informed decisions based on evidence. It's a method that helps scientists, researchers, and statisticians to distinguish between real effects and random variations in their data.
2024-04-11 02:49:57
reply(1)
Helpful(1122)
Helpful
Helpful(2)
Studied at Stanford University, Lives in Palo Alto. Entrepreneur in the tech industry, specializing in software development.
A statistical test that challenges a hypothesis to determine whether the alternative hypothesis produces a pre-established significance level. The significance test attempts to disprove the concept of "chance" and reject a null hypothesis by adhering to observed patterns.
2023-06-18 08:24:48
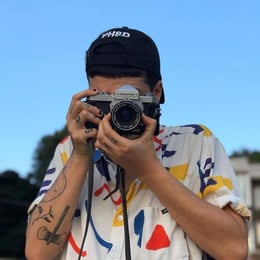
Ethan Roberts
QuesHub.com delivers expert answers and knowledge to you.
A statistical test that challenges a hypothesis to determine whether the alternative hypothesis produces a pre-established significance level. The significance test attempts to disprove the concept of "chance" and reject a null hypothesis by adhering to observed patterns.