Why is my data not statistically significant?
I'll answer
Earn 20 gold coins for an accepted answer.20
Earn 20 gold coins for an accepted answer.
40more
40more
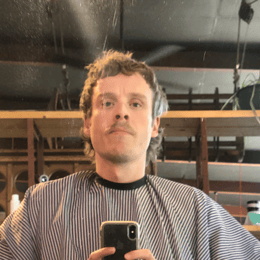
Lucas Scott
Works at Facebook, Lives in Menlo Park. Holds a degree in Computer Engineering from Stanford University.
As a statistician with extensive experience in data analysis, I can offer you some insights into why your data might not be statistically significant. Statistical significance is a critical concept in the field of statistics, and it's used to determine whether the results of a study are likely due to chance or not. Let's delve into some of the reasons why you might be facing this issue.
1. Sample Size: One of the most common reasons for non-significant results is a small sample size. The smaller the sample, the more likely it is that the results are due to random variation. Larger samples tend to provide more reliable results because they reduce the impact of random variation.
2. Effect Size: The effect size is the magnitude of the difference between groups or the strength of the relationship between variables. If the effect size is small, it may not be large enough to be detected as statistically significant, especially with a smaller sample size.
3. Variability Within the Data: High variability or spread in the data can make it difficult to detect a significant effect. This variability can be due to a number of factors, including measurement error, true differences among subjects, or the influence of confounding variables.
4. Power of the Test: The power of a statistical test is the probability that it will correctly reject a false null hypothesis (i.e., detect an effect when there is one). Low power can result from a small sample size, a small effect size, or high variability in the data.
5. Incorrect Statistical Assumptions: Many statistical tests have assumptions that must be met for the test to be valid. For example, t-tests assume that the data are normally distributed. If these assumptions are violated, the test may not be appropriate, and the results may not be significant.
6. Multiple Testing: If you're conducting multiple statistical tests on the same data set, the chance of finding at least one significant result by chance increases. This is known as the problem of multiple comparisons, and it can be addressed with corrections such as the Bonferroni correction.
7. Poor Study Design: A poorly designed study can lead to non-significant results. This might include issues such as not properly controlling for confounding variables, not using a representative sample, or not having a clear hypothesis to test.
8. Lack of Replication: Sometimes, a single study may not yield significant results, but replication of the study with similar methodology can increase confidence in the findings.
9. Publication Bias: There is a tendency in the scientific community to publish studies with significant results. This can lead to a skewed perception of what is considered "normal" in terms of statistical significance.
10. True Null Hypothesis: Lastly, it's possible that the null hypothesis is actually true, meaning there is no effect or difference to detect. This is a valid possibility that researchers must consider.
Understanding these factors can help you troubleshoot why your data might not be statistically significant. It's important to critically evaluate your study design, data collection methods, and analysis techniques to ensure that you're drawing accurate conclusions from your data.
1. Sample Size: One of the most common reasons for non-significant results is a small sample size. The smaller the sample, the more likely it is that the results are due to random variation. Larger samples tend to provide more reliable results because they reduce the impact of random variation.
2. Effect Size: The effect size is the magnitude of the difference between groups or the strength of the relationship between variables. If the effect size is small, it may not be large enough to be detected as statistically significant, especially with a smaller sample size.
3. Variability Within the Data: High variability or spread in the data can make it difficult to detect a significant effect. This variability can be due to a number of factors, including measurement error, true differences among subjects, or the influence of confounding variables.
4. Power of the Test: The power of a statistical test is the probability that it will correctly reject a false null hypothesis (i.e., detect an effect when there is one). Low power can result from a small sample size, a small effect size, or high variability in the data.
5. Incorrect Statistical Assumptions: Many statistical tests have assumptions that must be met for the test to be valid. For example, t-tests assume that the data are normally distributed. If these assumptions are violated, the test may not be appropriate, and the results may not be significant.
6. Multiple Testing: If you're conducting multiple statistical tests on the same data set, the chance of finding at least one significant result by chance increases. This is known as the problem of multiple comparisons, and it can be addressed with corrections such as the Bonferroni correction.
7. Poor Study Design: A poorly designed study can lead to non-significant results. This might include issues such as not properly controlling for confounding variables, not using a representative sample, or not having a clear hypothesis to test.
8. Lack of Replication: Sometimes, a single study may not yield significant results, but replication of the study with similar methodology can increase confidence in the findings.
9. Publication Bias: There is a tendency in the scientific community to publish studies with significant results. This can lead to a skewed perception of what is considered "normal" in terms of statistical significance.
10. True Null Hypothesis: Lastly, it's possible that the null hypothesis is actually true, meaning there is no effect or difference to detect. This is a valid possibility that researchers must consider.
Understanding these factors can help you troubleshoot why your data might not be statistically significant. It's important to critically evaluate your study design, data collection methods, and analysis techniques to ensure that you're drawing accurate conclusions from your data.
2024-04-21 04:06:11
reply(1)
Helpful(1122)
Helpful
Helpful(2)
Studied at the University of Seoul, Lives in Seoul, South Korea.
Not Due to Chance. In principle, a statistically significant result (usually a difference) is a result that's not attributed to chance. More technically, it means that if the Null Hypothesis is true (which means there really is no difference), there's a low probability of getting a result that large or larger.Oct 21, 2014
2023-06-18 08:00:35
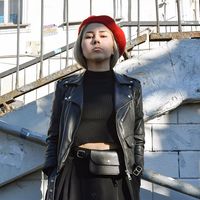
Harper Lee
QuesHub.com delivers expert answers and knowledge to you.
Not Due to Chance. In principle, a statistically significant result (usually a difference) is a result that's not attributed to chance. More technically, it means that if the Null Hypothesis is true (which means there really is no difference), there's a low probability of getting a result that large or larger.Oct 21, 2014