How is the critical value calculated?
I'll answer
Earn 20 gold coins for an accepted answer.20
Earn 20 gold coins for an accepted answer.
40more
40more
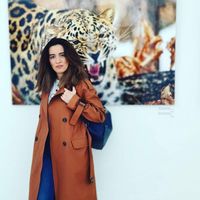
Harper Adams
Studied at the University of Zurich, Lives in Zurich, Switzerland.
As a statistical expert with a deep understanding of hypothesis testing and statistical inference, I can explain how the critical value is calculated in a hypothesis test. The critical value is a pivotal concept in determining whether to reject the null hypothesis based on the observed data. It is derived from the distribution of the test statistic under the null hypothesis and is used to define the rejection region of the test.
When conducting a hypothesis test, researchers typically start with a null hypothesis \( H_0 \) and an alternative hypothesis \( H_1 \). The null hypothesis represents a status quo or a claim that is being tested, while the alternative hypothesis represents the opposite of the null hypothesis or what the researcher is considering as a potential alternative.
The critical value approach involves the following steps:
1. State the Hypotheses: Clearly define \( H_0 \) and \( H_1 \), which respectively represent the null and alternative hypotheses.
2. Choose a Significance Level: Select a significance level, denoted by \( \alpha \), which is the probability of making a Type I error (rejecting the null hypothesis when it is true). Commonly used significance levels are 0.01, 0.05, and 0.10.
3. Determine the Test Statistic: Identify the appropriate test statistic for the hypothesis test. This could be a t-statistic, z-score, chi-square value, F-ratio, etc., depending on the nature of the data and the hypotheses.
4. **Find the Distribution of the Test Statistic**: Determine the sampling distribution of the test statistic under the null hypothesis. This could be a t-distribution, normal distribution, chi-square distribution, F-distribution, etc.
5. Calculate the Critical Value: The critical value is the value in the distribution of the test statistic such that the probability of observing a test statistic as extreme or more extreme than this value, when \( H_0 \) is true, is equal to \( \alpha \). For a one-tailed test, this value is found in the tail of the distribution corresponding to the alternative hypothesis. For a two-tailed test, the \( \alpha \) level is split between the two tails, and the critical values are found in both tails.
6. Make a Decision: Compare the observed value of the test statistic to the critical value(s). If the test statistic falls in the rejection region (i.e., it is more extreme than the critical value), the null hypothesis is rejected in favor of the alternative hypothesis. If it does not fall in the rejection region, the null hypothesis is not rejected.
It's important to note that the critical value is not the same as the p-value, although they are related. The p-value is the probability of observing a test statistic as extreme as, or more extreme than, the one calculated from the sample data, given that the null hypothesis is true. If the p-value is less than or equal to the significance level \( \alpha \), the null hypothesis is rejected.
The process of calculating the critical value is crucial for maintaining the integrity of the test and ensuring that the conclusions drawn from the data are statistically sound. It helps researchers balance the trade-off between the risks of Type I and Type II errors.
Now, let's translate the above explanation into Chinese.
When conducting a hypothesis test, researchers typically start with a null hypothesis \( H_0 \) and an alternative hypothesis \( H_1 \). The null hypothesis represents a status quo or a claim that is being tested, while the alternative hypothesis represents the opposite of the null hypothesis or what the researcher is considering as a potential alternative.
The critical value approach involves the following steps:
1. State the Hypotheses: Clearly define \( H_0 \) and \( H_1 \), which respectively represent the null and alternative hypotheses.
2. Choose a Significance Level: Select a significance level, denoted by \( \alpha \), which is the probability of making a Type I error (rejecting the null hypothesis when it is true). Commonly used significance levels are 0.01, 0.05, and 0.10.
3. Determine the Test Statistic: Identify the appropriate test statistic for the hypothesis test. This could be a t-statistic, z-score, chi-square value, F-ratio, etc., depending on the nature of the data and the hypotheses.
4. **Find the Distribution of the Test Statistic**: Determine the sampling distribution of the test statistic under the null hypothesis. This could be a t-distribution, normal distribution, chi-square distribution, F-distribution, etc.
5. Calculate the Critical Value: The critical value is the value in the distribution of the test statistic such that the probability of observing a test statistic as extreme or more extreme than this value, when \( H_0 \) is true, is equal to \( \alpha \). For a one-tailed test, this value is found in the tail of the distribution corresponding to the alternative hypothesis. For a two-tailed test, the \( \alpha \) level is split between the two tails, and the critical values are found in both tails.
6. Make a Decision: Compare the observed value of the test statistic to the critical value(s). If the test statistic falls in the rejection region (i.e., it is more extreme than the critical value), the null hypothesis is rejected in favor of the alternative hypothesis. If it does not fall in the rejection region, the null hypothesis is not rejected.
It's important to note that the critical value is not the same as the p-value, although they are related. The p-value is the probability of observing a test statistic as extreme as, or more extreme than, the one calculated from the sample data, given that the null hypothesis is true. If the p-value is less than or equal to the significance level \( \alpha \), the null hypothesis is rejected.
The process of calculating the critical value is crucial for maintaining the integrity of the test and ensuring that the conclusions drawn from the data are statistically sound. It helps researchers balance the trade-off between the risks of Type I and Type II errors.
Now, let's translate the above explanation into Chinese.
2024-04-25 23:01:52
reply(1)
Helpful(1122)
Helpful
Helpful(2)
Studied at the University of Barcelona, Lives in Barcelona, Spain.
Critical value approach. ... Determine the critical value by finding the value of the known distribution of the test statistic such that the probability of making a Type I error -- which is denoted -- (greek letter "alpha") and is called the "significance level of the test" -- is small (typically 0.01, 0.05, or 0.10).
2023-06-26 08:00:24

Benjamin Martinez
QuesHub.com delivers expert answers and knowledge to you.
Critical value approach. ... Determine the critical value by finding the value of the known distribution of the test statistic such that the probability of making a Type I error -- which is denoted -- (greek letter "alpha") and is called the "significance level of the test" -- is small (typically 0.01, 0.05, or 0.10).