What does a significant test tell us?
I'll answer
Earn 20 gold coins for an accepted answer.20
Earn 20 gold coins for an accepted answer.
40more
40more
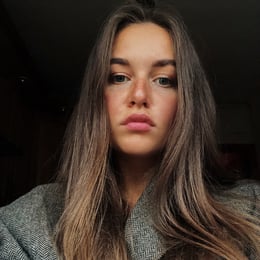
Skylar Gonzales
Studied at the University of Toronto, Lives in Toronto, Canada.
As a domain expert with a background in statistics and data analysis, I can provide a comprehensive answer to your question about what a significant test tells us.
A significant test is a crucial tool in statistical analysis that helps researchers and analysts determine whether the results of their study are due to chance or if there is a genuine relationship between the variables being studied. It is used to estimate the probability that a relationship observed in the data occurred only by chance, which is known as the p-value. The p-value represents the probability of obtaining results as extreme as, or more extreme than, those observed under the assumption that there is no true effect or relationship (the null hypothesis is true).
Here are the key points that a significant test can tell us:
1. Hypothesis Testing: A significant test begins with the formulation of a null hypothesis (H0) and an alternative hypothesis (H1 or Ha). The null hypothesis typically represents the status quo or a claim of no effect, while the alternative hypothesis represents the research hypothesis that the researcher is trying to prove.
2. p-value: The p-value is the cornerstone of a significant test. It is calculated based on the test statistic and the chosen significance level (alpha), which is a threshold set by the researcher to determine statistical significance. A low p-value (typically ≤ 0.05) indicates strong evidence against the null hypothesis, suggesting that the observed effect is likely not due to chance.
3. Type I and Type II Errors: A significant test also helps us understand the potential errors in our conclusions. A Type I error occurs when we reject the null hypothesis when it is actually true (a false positive), while a Type II error occurs when we fail to reject the null hypothesis when it is false (a false negative). The significance level (alpha) controls the probability of a Type I error.
4. Confidence Intervals: Along with hypothesis testing, significant tests can be used to calculate confidence intervals, which provide a range of values within which the true population parameter is likely to fall. The width of the confidence interval is inversely related to the level of confidence.
5. Power of a Test: The power of a test is the probability that it will correctly reject a false null hypothesis. It is influenced by the significance level, the size of the effect being studied, and the sample size. A higher power reduces the risk of a Type II error.
6. Effect Size: While significant tests tell us if there is a statistically significant relationship, they do not tell us how large that relationship is. Effect size measures provide this information, allowing researchers to understand the practical significance of their findings.
7.
Multiple Comparisons: When conducting multiple tests, the risk of Type I errors increases. Techniques such as Bonferroni correction or false discovery rate (FDR) control can be used to adjust for this.
8.
Robustness and Assumptions: A significant test's results are only as good as the assumptions it is based on. Tests often assume that the data are normally distributed, that observations are independent, and that the sample is representative of the population. Violations of these assumptions can lead to misleading results.
9.
Real-world Implications: Ultimately, a significant test helps researchers decide whether their findings are likely to hold true in the real world. It can guide decisions about further research, policy changes, or practical applications.
In conclusion, a significant test is a powerful tool for determining the reliability of observed relationships in data. It allows researchers to make informed decisions about the validity of their findings and the potential impact of those findings on the broader field of study.
A significant test is a crucial tool in statistical analysis that helps researchers and analysts determine whether the results of their study are due to chance or if there is a genuine relationship between the variables being studied. It is used to estimate the probability that a relationship observed in the data occurred only by chance, which is known as the p-value. The p-value represents the probability of obtaining results as extreme as, or more extreme than, those observed under the assumption that there is no true effect or relationship (the null hypothesis is true).
Here are the key points that a significant test can tell us:
1. Hypothesis Testing: A significant test begins with the formulation of a null hypothesis (H0) and an alternative hypothesis (H1 or Ha). The null hypothesis typically represents the status quo or a claim of no effect, while the alternative hypothesis represents the research hypothesis that the researcher is trying to prove.
2. p-value: The p-value is the cornerstone of a significant test. It is calculated based on the test statistic and the chosen significance level (alpha), which is a threshold set by the researcher to determine statistical significance. A low p-value (typically ≤ 0.05) indicates strong evidence against the null hypothesis, suggesting that the observed effect is likely not due to chance.
3. Type I and Type II Errors: A significant test also helps us understand the potential errors in our conclusions. A Type I error occurs when we reject the null hypothesis when it is actually true (a false positive), while a Type II error occurs when we fail to reject the null hypothesis when it is false (a false negative). The significance level (alpha) controls the probability of a Type I error.
4. Confidence Intervals: Along with hypothesis testing, significant tests can be used to calculate confidence intervals, which provide a range of values within which the true population parameter is likely to fall. The width of the confidence interval is inversely related to the level of confidence.
5. Power of a Test: The power of a test is the probability that it will correctly reject a false null hypothesis. It is influenced by the significance level, the size of the effect being studied, and the sample size. A higher power reduces the risk of a Type II error.
6. Effect Size: While significant tests tell us if there is a statistically significant relationship, they do not tell us how large that relationship is. Effect size measures provide this information, allowing researchers to understand the practical significance of their findings.
7.
Multiple Comparisons: When conducting multiple tests, the risk of Type I errors increases. Techniques such as Bonferroni correction or false discovery rate (FDR) control can be used to adjust for this.
8.
Robustness and Assumptions: A significant test's results are only as good as the assumptions it is based on. Tests often assume that the data are normally distributed, that observations are independent, and that the sample is representative of the population. Violations of these assumptions can lead to misleading results.
9.
Real-world Implications: Ultimately, a significant test helps researchers decide whether their findings are likely to hold true in the real world. It can guide decisions about further research, policy changes, or practical applications.
In conclusion, a significant test is a powerful tool for determining the reliability of observed relationships in data. It allows researchers to make informed decisions about the validity of their findings and the potential impact of those findings on the broader field of study.
2024-04-24 14:57:47
reply(1)
Helpful(1122)
Helpful
Helpful(2)
Studied at Massachusetts Institute of Technology (MIT), Lives in Cambridge. Currently conducting research in the field of renewable energy.
Tests for statistical significance are used to estimate the probability that a relationship observed in the data occurred only by chance; the probability that the variables are really unrelated in the population. They can be used to filter out unpromising hypotheses.
2023-06-18 07:44:26
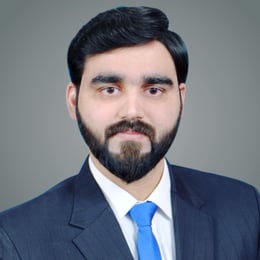
Ethan Hall
QuesHub.com delivers expert answers and knowledge to you.
Tests for statistical significance are used to estimate the probability that a relationship observed in the data occurred only by chance; the probability that the variables are really unrelated in the population. They can be used to filter out unpromising hypotheses.