What is the level of significance in statistics?
I'll answer
Earn 20 gold coins for an accepted answer.20
Earn 20 gold coins for an accepted answer.
40more
40more

Benjamin Martinez
Works at the International Committee of the Red Cross, Lives in Geneva, Switzerland.
As a statistical expert with a deep understanding of the intricacies of statistical analysis, I would like to explain the concept of significance level in statistics. The significance level, often denoted by the Greek letter alpha (α), is a fundamental concept in hypothesis testing and plays a crucial role in determining the validity of research findings.
When conducting a statistical test, researchers typically start with a null hypothesis (H0), which represents the status quo or the assumption that there is no effect or no difference. The alternative hypothesis (H1 or Ha) posits that there is an effect or a difference. The significance level is the threshold that determines whether the results of the test are statistically significant, meaning that the observed data would be unlikely to occur if the null hypothesis were true.
The significance level is the probability of rejecting the null hypothesis when it is actually true, which is also known as a type I error. This error is also referred to as a false positive. The significance level is a pre-determined threshold that researchers set before conducting the test to control the risk of making this type of error.
Typically, the significance level is set at or below 5%, which means that there is a 5% chance of rejecting the null hypothesis when it is true. This threshold is arbitrary but has become a standard in many fields because it provides a balance between avoiding false positives and detecting true effects. However, in some fields, such as drug testing, a lower significance level, such as 1%, might be used to minimize the risk of approving a harmful drug.
The process of determining statistical significance involves calculating a test statistic, which is then compared to a critical value from a statistical distribution. If the test statistic is more extreme than the critical value, or if the calculated p-value is less than the significance level, the null hypothesis is rejected in favor of the alternative hypothesis.
The p-value is a related concept that represents the probability of observing the test results under the assumption that the null hypothesis is true. A low p-value indicates strong evidence against the null hypothesis. If the p-value is less than the significance level, it suggests that the results are statistically significant, and the null hypothesis is rejected.
It's important to note that statistical significance does not necessarily imply practical significance. A result might be statistically significant but still be too small to be meaningful in a real-world context. Researchers must also consider the size of the effect, the confidence intervals, and the context of the findings when interpreting the results.
Moreover, the choice of the significance level should be based on the consequences of type I and type II errors (the latter being a false negative), the costs of these errors, and the goals of the research. For instance, in life-threatening situations, a lower significance level might be more appropriate to avoid making a decision that could harm patients.
In conclusion, the significance level is a critical concept in statistical analysis that helps researchers make informed decisions about the validity of their findings. It is a tool for controlling the risk of false positives and is set to balance the need for reliable evidence with the potential for missing true effects.
When conducting a statistical test, researchers typically start with a null hypothesis (H0), which represents the status quo or the assumption that there is no effect or no difference. The alternative hypothesis (H1 or Ha) posits that there is an effect or a difference. The significance level is the threshold that determines whether the results of the test are statistically significant, meaning that the observed data would be unlikely to occur if the null hypothesis were true.
The significance level is the probability of rejecting the null hypothesis when it is actually true, which is also known as a type I error. This error is also referred to as a false positive. The significance level is a pre-determined threshold that researchers set before conducting the test to control the risk of making this type of error.
Typically, the significance level is set at or below 5%, which means that there is a 5% chance of rejecting the null hypothesis when it is true. This threshold is arbitrary but has become a standard in many fields because it provides a balance between avoiding false positives and detecting true effects. However, in some fields, such as drug testing, a lower significance level, such as 1%, might be used to minimize the risk of approving a harmful drug.
The process of determining statistical significance involves calculating a test statistic, which is then compared to a critical value from a statistical distribution. If the test statistic is more extreme than the critical value, or if the calculated p-value is less than the significance level, the null hypothesis is rejected in favor of the alternative hypothesis.
The p-value is a related concept that represents the probability of observing the test results under the assumption that the null hypothesis is true. A low p-value indicates strong evidence against the null hypothesis. If the p-value is less than the significance level, it suggests that the results are statistically significant, and the null hypothesis is rejected.
It's important to note that statistical significance does not necessarily imply practical significance. A result might be statistically significant but still be too small to be meaningful in a real-world context. Researchers must also consider the size of the effect, the confidence intervals, and the context of the findings when interpreting the results.
Moreover, the choice of the significance level should be based on the consequences of type I and type II errors (the latter being a false negative), the costs of these errors, and the goals of the research. For instance, in life-threatening situations, a lower significance level might be more appropriate to avoid making a decision that could harm patients.
In conclusion, the significance level is a critical concept in statistical analysis that helps researchers make informed decisions about the validity of their findings. It is a tool for controlling the risk of false positives and is set to balance the need for reliable evidence with the potential for missing true effects.
2024-04-20 16:37:11
reply(1)
Helpful(1122)
Helpful
Helpful(2)
Works at Adobe, Lives in San Jose, CA
The null hypothesis is rejected if the p-value is less than a predetermined level, --. -- is called the significance level, and is the probability of rejecting the null hypothesis given that it is true (a type I error). It is usually set at or below 5%.
2023-06-25 07:44:23
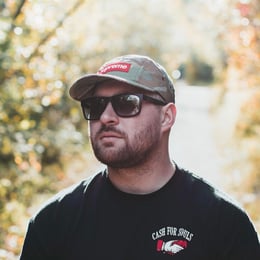
Owen Martinez
QuesHub.com delivers expert answers and knowledge to you.
The null hypothesis is rejected if the p-value is less than a predetermined level, --. -- is called the significance level, and is the probability of rejecting the null hypothesis given that it is true (a type I error). It is usually set at or below 5%.