What are the types of errors?
I'll answer
Earn 20 gold coins for an accepted answer.20
Earn 20 gold coins for an accepted answer.
40more
40more
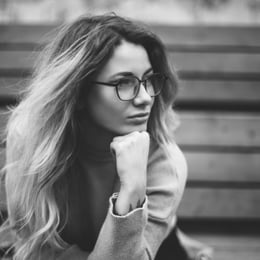
Scarlett Gonzales
Studied at the University of Cape Town, Lives in Cape Town, South Africa.
In the field of statistics, errors can occur during hypothesis testing, which is a method used to make decisions about the population based on sample data. These errors are classified into two main types: Type I and Type II errors. Understanding these errors is crucial for interpreting the results of statistical tests and making informed decisions.
### Type I Error
A Type I error, also known as a false positive, occurs when the null hypothesis (H0) is true, but it is rejected incorrectly. In other words, it's the mistake of finding evidence against something that is actually true. This error is associated with the significance level (α), which is the probability of rejecting the null hypothesis when it is true. The significance level is set by the researcher before conducting the test and is often set at 0.05, meaning there is a 5% chance of committing a Type I error.
For example, in a clinical trial, if a new drug is tested against a placebo, a Type I error would occur if the drug is declared effective when it is not. This could lead to unnecessary treatment costs and potential side effects for patients.
### Type II Error
Conversely, a Type II error, also known as a false negative, occurs when the null hypothesis is false, but it is not rejected. This means that the evidence is not strong enough to support the alternative hypothesis (H1), leading to the acceptance of a false null hypothesis. The probability of making a Type II error is denoted by β, and the power of a test (1 - β) is the probability of correctly rejecting a false null hypothesis.
In the context of the clinical trial, a Type II error would occur if the drug is not declared effective when it actually is. This could result in patients missing out on a beneficial treatment.
### Factors Influencing Errors
Several factors can influence the likelihood of committing these errors:
1. Sample Size: Larger samples tend to reduce the chance of both types of errors.
2. Effect Size: The magnitude of the difference between groups can affect the likelihood of detecting a true effect.
3. Significance Level (α): A lower α reduces the chance of a Type I error but increases the chance of a Type II error.
4. Power of the Test (1 - β): Higher power reduces the chance of a Type II error but does not affect the Type I error rate.
### Balancing Errors
In practice, researchers must balance the risks of committing Type I and Type II errors. Reducing one type of error often increases the risk of the other. For instance, increasing the sample size can decrease both types of errors but may not be feasible due to time or cost constraints.
### Conclusion
Understanding the types of errors in statistical hypothesis testing is essential for researchers and practitioners to interpret results accurately and make decisions that are both statistically valid and practically meaningful. It's important to design studies with an appropriate balance between the risks of these errors, considering the significance level, power, sample size, and the effect size.
### Type I Error
A Type I error, also known as a false positive, occurs when the null hypothesis (H0) is true, but it is rejected incorrectly. In other words, it's the mistake of finding evidence against something that is actually true. This error is associated with the significance level (α), which is the probability of rejecting the null hypothesis when it is true. The significance level is set by the researcher before conducting the test and is often set at 0.05, meaning there is a 5% chance of committing a Type I error.
For example, in a clinical trial, if a new drug is tested against a placebo, a Type I error would occur if the drug is declared effective when it is not. This could lead to unnecessary treatment costs and potential side effects for patients.
### Type II Error
Conversely, a Type II error, also known as a false negative, occurs when the null hypothesis is false, but it is not rejected. This means that the evidence is not strong enough to support the alternative hypothesis (H1), leading to the acceptance of a false null hypothesis. The probability of making a Type II error is denoted by β, and the power of a test (1 - β) is the probability of correctly rejecting a false null hypothesis.
In the context of the clinical trial, a Type II error would occur if the drug is not declared effective when it actually is. This could result in patients missing out on a beneficial treatment.
### Factors Influencing Errors
Several factors can influence the likelihood of committing these errors:
1. Sample Size: Larger samples tend to reduce the chance of both types of errors.
2. Effect Size: The magnitude of the difference between groups can affect the likelihood of detecting a true effect.
3. Significance Level (α): A lower α reduces the chance of a Type I error but increases the chance of a Type II error.
4. Power of the Test (1 - β): Higher power reduces the chance of a Type II error but does not affect the Type I error rate.
### Balancing Errors
In practice, researchers must balance the risks of committing Type I and Type II errors. Reducing one type of error often increases the risk of the other. For instance, increasing the sample size can decrease both types of errors but may not be feasible due to time or cost constraints.
### Conclusion
Understanding the types of errors in statistical hypothesis testing is essential for researchers and practitioners to interpret results accurately and make decisions that are both statistically valid and practically meaningful. It's important to design studies with an appropriate balance between the risks of these errors, considering the significance level, power, sample size, and the effect size.
2024-04-13 21:52:46
reply(1)
Helpful(1122)
Helpful
Helpful(2)
Studied at the University of Barcelona, Lives in Barcelona, Spain.
Type I and type II errors. ... In statistical hypothesis testing, a type I error is the rejection of a true null hypothesis (also known as a "false positive" finding), while a type II error is retaining a false null hypothesis (also known as a "false negative" finding).
2023-06-18 07:36:35
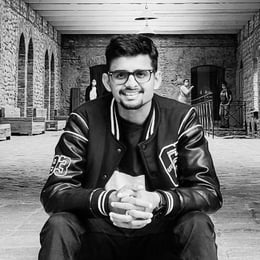
Benjamin Baker
QuesHub.com delivers expert answers and knowledge to you.
Type I and type II errors. ... In statistical hypothesis testing, a type I error is the rejection of a true null hypothesis (also known as a "false positive" finding), while a type II error is retaining a false null hypothesis (also known as a "false negative" finding).