What does it mean to have a large chi square value?
I'll answer
Earn 20 gold coins for an accepted answer.20
Earn 20 gold coins for an accepted answer.
40more
40more
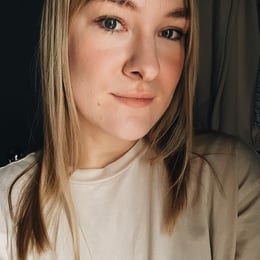
Ruby Turner
Studied at University College London (UCL), Lives in London, UK
As a statistician with a strong background in data analysis and hypothesis testing, I often encounter the chi-square (χ²) statistic in my work. The chi-square test is a powerful tool used to determine whether there is a significant difference between the expected frequencies and the observed frequencies in a dataset. It's widely used in various fields, including social sciences, biology, and engineering, to test hypotheses about categorical data.
When we talk about a large chi-square value, we are referring to a value that is significantly greater than what would be expected under the null hypothesis. The null hypothesis typically posits that there is no association between the variables being studied or that the observed data fits a particular model.
Here's a breakdown of what a large chi-square value might indicate:
1. Rejection of the Null Hypothesis: If the calculated chi-square value exceeds the critical value from the chi-square distribution table, it suggests that the observed data significantly deviates from the expected data under the null hypothesis. In such a case, we reject the null hypothesis in favor of the alternative hypothesis, which posits that there is an association or a discrepancy between the variables.
2. Degrees of Freedom (df): The chi-square statistic is influenced by the number of degrees of freedom. Degrees of freedom in the context of a chi-square test refer to the number of ways the data can vary independently. For example, if you have a table with r rows and c columns, the degrees of freedom would be (r-1)(c-1). The larger the degrees of freedom, the more spread out the chi-square distribution becomes, and thus, the critical value at which we reject the null hypothesis increases.
3. Critical Value: The critical value is a threshold derived from the chi-square distribution table. It is used to determine the decision rule for hypothesis testing. If your calculated chi-square statistic is greater than the critical value, it indicates a low probability that the observed data could have occurred by chance if the null hypothesis were true.
4. Association and Independence: In the context of a chi-square test for independence, a large chi-square value suggests that the variables are not independent; there is a statistically significant association between them.
5. Goodness of Fit: When used to test for goodness of fit, such as whether a sample comes from a population with a specific distribution, a large chi-square value indicates that the observed distribution of data significantly differs from the expected distribution.
6. Sample Size: The sample size also plays a crucial role. Larger sample sizes can lead to larger chi-square values because they provide more information and can detect smaller differences that might not be apparent in smaller samples.
7.
Significance Level (α): The significance level, often denoted by α, is the probability of rejecting the null hypothesis when it is true. A common significance level is 0.05, which means there is a 5% chance of rejecting the null hypothesis by chance if it is actually true. The chi-square value must be compared to the critical value at this significance level to determine the outcome of the test.
8. **Practical Significance vs. Statistical Significance**: While a large chi-square value provides statistical significance, it's also important to consider the practical significance of the findings. A statistically significant result may not necessarily be meaningful in a real-world context.
In conclusion, a large chi-square value is a signal that the data under analysis does not align with the expectations set by the null hypothesis. It's a statistical indication that there is a significant difference or association that warrants further investigation. However, it's essential to interpret these results within the context of the study and consider other factors such as effect size and the practical implications of the findings.
When we talk about a large chi-square value, we are referring to a value that is significantly greater than what would be expected under the null hypothesis. The null hypothesis typically posits that there is no association between the variables being studied or that the observed data fits a particular model.
Here's a breakdown of what a large chi-square value might indicate:
1. Rejection of the Null Hypothesis: If the calculated chi-square value exceeds the critical value from the chi-square distribution table, it suggests that the observed data significantly deviates from the expected data under the null hypothesis. In such a case, we reject the null hypothesis in favor of the alternative hypothesis, which posits that there is an association or a discrepancy between the variables.
2. Degrees of Freedom (df): The chi-square statistic is influenced by the number of degrees of freedom. Degrees of freedom in the context of a chi-square test refer to the number of ways the data can vary independently. For example, if you have a table with r rows and c columns, the degrees of freedom would be (r-1)(c-1). The larger the degrees of freedom, the more spread out the chi-square distribution becomes, and thus, the critical value at which we reject the null hypothesis increases.
3. Critical Value: The critical value is a threshold derived from the chi-square distribution table. It is used to determine the decision rule for hypothesis testing. If your calculated chi-square statistic is greater than the critical value, it indicates a low probability that the observed data could have occurred by chance if the null hypothesis were true.
4. Association and Independence: In the context of a chi-square test for independence, a large chi-square value suggests that the variables are not independent; there is a statistically significant association between them.
5. Goodness of Fit: When used to test for goodness of fit, such as whether a sample comes from a population with a specific distribution, a large chi-square value indicates that the observed distribution of data significantly differs from the expected distribution.
6. Sample Size: The sample size also plays a crucial role. Larger sample sizes can lead to larger chi-square values because they provide more information and can detect smaller differences that might not be apparent in smaller samples.
7.
Significance Level (α): The significance level, often denoted by α, is the probability of rejecting the null hypothesis when it is true. A common significance level is 0.05, which means there is a 5% chance of rejecting the null hypothesis by chance if it is actually true. The chi-square value must be compared to the critical value at this significance level to determine the outcome of the test.
8. **Practical Significance vs. Statistical Significance**: While a large chi-square value provides statistical significance, it's also important to consider the practical significance of the findings. A statistically significant result may not necessarily be meaningful in a real-world context.
In conclusion, a large chi-square value is a signal that the data under analysis does not align with the expectations set by the null hypothesis. It's a statistical indication that there is a significant difference or association that warrants further investigation. However, it's essential to interpret these results within the context of the study and consider other factors such as effect size and the practical implications of the findings.
2024-04-18 03:56:29
reply(1)
Helpful(1122)
Helpful
Helpful(2)
Studied at University of Michigan, Lives in Ann Arbor, MI
So for a test with 1 df (degree of freedom), the "critical" value of the chi-square statistic is 3.84. What does critical value mean? Basically, if the chi-square you calculated was bigger than the critical value in the table, then the data did not fit the model, which means you have to reject the null hypothesis.
2023-06-19 07:28:24

Oliver Lee
QuesHub.com delivers expert answers and knowledge to you.
So for a test with 1 df (degree of freedom), the "critical" value of the chi-square statistic is 3.84. What does critical value mean? Basically, if the chi-square you calculated was bigger than the critical value in the table, then the data did not fit the model, which means you have to reject the null hypothesis.