What do the results of a chi square test mean?
I'll answer
Earn 20 gold coins for an accepted answer.20
Earn 20 gold coins for an accepted answer.
40more
40more
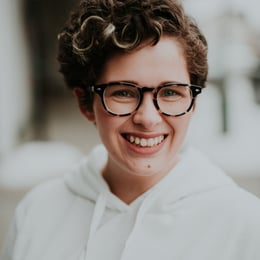
Charlotte Harris
Studied at University of Oxford, Lives in Oxford, UK
As a statistician with extensive experience in data analysis and interpretation, I often encounter the need to apply and explain statistical tests. One of the most commonly used tests in this field is the Chi-square test. The Chi-square test is a statistical tool that helps us determine whether there is a significant association between two categorical variables in a population.
The results of a Chi-square test can be interpreted in several ways, but fundamentally, they tell us about the likelihood that the observed distribution of data within categories is due to random chance rather than a true underlying relationship. Here's a more detailed breakdown:
### Purpose of the Chi-square Test
1. Goodness of Fit: The Chi-square test is often used to assess whether a sample data matches a theoretical distribution. For instance, if we expect a certain distribution of outcomes (like a fair coin toss resulting in 50% heads and 50% tails), the test can tell us if our observed data significantly deviates from this expectation.
2. Test of Independence: It can also be used to determine if two categorical variables are independent of each other. For example, in a study examining the relationship between smoking status and lung cancer, the Chi-square test can help determine if there's a statistically significant link between these two variables.
### Interpreting the Results
1. Chi-square Statistic (χ²): This is the test statistic computed from the observed and expected frequencies in the dataset. A larger Chi-square value indicates a greater difference between the observed and expected data.
2. Degrees of Freedom (df): This is a key component in the Chi-square test that is based on the number of categories in the dataset. The formula for degrees of freedom is typically (number of rows - 1) * (number of columns - 1).
3. p-value: Perhaps the most critical piece of information, the p-value tells us how likely it is that the observed results occurred by chance if there truly is no association between the variables. A low p-value (usually ≤ 0.05) suggests that the differences are statistically significant, and we can reject the null hypothesis that there is no association.
4. Effect Size: While the Chi-square test tells us if there's a significant association, it doesn't tell us how strong that association is. Measures like Cramer's V or Phi coefficient can provide insight into the strength of the relationship.
5. Contingency Table: The Chi-square test often involves creating a contingency table that displays the frequencies of categorical variables in a two-dimensional table. This table is crucial for calculating the expected frequencies against which the observed frequencies are compared.
### Assumptions and Limitations
- The data should be in categorical form.
- The observations should be independent of each other.
- The expected frequency in each cell of the table should be at least 5 for the test to be valid. If this assumption is violated, a Fisher's Exact Test might be more appropriate.
### Real-world Applications
The Chi-square test is widely used in social sciences, biology, marketing, and quality control, among other fields. It's a versatile test that can handle large datasets and complex categorical relationships.
In conclusion, the Chi-square test is a powerful statistical method for analyzing categorical data. It provides a way to determine if observed distributions are likely due to chance or if they suggest a non-random association between variables. Understanding the results requires careful consideration of the Chi-square statistic, degrees of freedom, p-value, and the context of the data.
The results of a Chi-square test can be interpreted in several ways, but fundamentally, they tell us about the likelihood that the observed distribution of data within categories is due to random chance rather than a true underlying relationship. Here's a more detailed breakdown:
### Purpose of the Chi-square Test
1. Goodness of Fit: The Chi-square test is often used to assess whether a sample data matches a theoretical distribution. For instance, if we expect a certain distribution of outcomes (like a fair coin toss resulting in 50% heads and 50% tails), the test can tell us if our observed data significantly deviates from this expectation.
2. Test of Independence: It can also be used to determine if two categorical variables are independent of each other. For example, in a study examining the relationship between smoking status and lung cancer, the Chi-square test can help determine if there's a statistically significant link between these two variables.
### Interpreting the Results
1. Chi-square Statistic (χ²): This is the test statistic computed from the observed and expected frequencies in the dataset. A larger Chi-square value indicates a greater difference between the observed and expected data.
2. Degrees of Freedom (df): This is a key component in the Chi-square test that is based on the number of categories in the dataset. The formula for degrees of freedom is typically (number of rows - 1) * (number of columns - 1).
3. p-value: Perhaps the most critical piece of information, the p-value tells us how likely it is that the observed results occurred by chance if there truly is no association between the variables. A low p-value (usually ≤ 0.05) suggests that the differences are statistically significant, and we can reject the null hypothesis that there is no association.
4. Effect Size: While the Chi-square test tells us if there's a significant association, it doesn't tell us how strong that association is. Measures like Cramer's V or Phi coefficient can provide insight into the strength of the relationship.
5. Contingency Table: The Chi-square test often involves creating a contingency table that displays the frequencies of categorical variables in a two-dimensional table. This table is crucial for calculating the expected frequencies against which the observed frequencies are compared.
### Assumptions and Limitations
- The data should be in categorical form.
- The observations should be independent of each other.
- The expected frequency in each cell of the table should be at least 5 for the test to be valid. If this assumption is violated, a Fisher's Exact Test might be more appropriate.
### Real-world Applications
The Chi-square test is widely used in social sciences, biology, marketing, and quality control, among other fields. It's a versatile test that can handle large datasets and complex categorical relationships.
In conclusion, the Chi-square test is a powerful statistical method for analyzing categorical data. It provides a way to determine if observed distributions are likely due to chance or if they suggest a non-random association between variables. Understanding the results requires careful consideration of the Chi-square statistic, degrees of freedom, p-value, and the context of the data.
2024-04-04 06:59:07
reply(1)
Helpful(1122)
Helpful
Helpful(2)
Studied at the University of Cambridge, Lives in Cambridge, UK.
The Chi-square test is intended to test how likely it is that an observed distribution is due to chance. It is also called a "goodness of fit" statistic, because it measures how well the observed distribution of data fits with the distribution that is expected if the variables are independent.
2023-06-17 07:28:23

Zoe Clark
QuesHub.com delivers expert answers and knowledge to you.
The Chi-square test is intended to test how likely it is that an observed distribution is due to chance. It is also called a "goodness of fit" statistic, because it measures how well the observed distribution of data fits with the distribution that is expected if the variables are independent.