What is meant by Type 1 and Type 2 error?
I'll answer
Earn 20 gold coins for an accepted answer.20
Earn 20 gold coins for an accepted answer.
40more
40more

Parker Lewis
Works at the United Nations, Lives in New York, NY, USA.
In the realm of statistical hypothesis testing, understanding the concepts of Type I and Type II errors is crucial for interpreting the results of experiments and studies accurately. These errors represent different kinds of incorrect conclusions that can be drawn from statistical data, and they have significant implications for decision-making in various fields, including science, medicine, economics, and social sciences.
Type I Error (False Positive):
A Type I error occurs when the null hypothesis (H0) is incorrectly rejected. This means that there is a false detection of an effect or a relationship that does not actually exist. The null hypothesis typically represents the default position that there is no effect or no difference between groups. When a Type I error is made, the researcher concludes that there is a significant effect when in reality there is none.
The probability of making a Type I error is denoted by the Greek letter alpha (α), which is also known as the significance level of the test. This level is set by the researcher before conducting the study and is often set at 0.05, meaning there is a 5% chance of making a Type I error if the null hypothesis is true.
Type II Error (False Negative):
Conversely, a Type II error happens when the null hypothesis is incorrectly not rejected. This means that the researcher fails to detect an effect or a relationship that actually exists. In other words, a Type II error is like missing out on a true positive finding. The probability of making a Type II error is represented by the Greek letter beta (β), and the power of a test, which is the probability of correctly rejecting the null hypothesis when it is false (1 - β), is a key consideration in study design.
Balancing Type I and Type II Errors:
There is often a trade-off between the two types of errors. Reducing the likelihood of a Type I error (making the test more stringent) can increase the likelihood of a Type II error, and vice versa. This balance is critical in study design and is influenced by the consequences of each type of error. For example, in medical testing, a false positive might lead to unnecessary treatment, while a false negative could mean a missed diagnosis.
Factors Influencing Errors:
Several factors can influence the likelihood of committing Type I and Type II errors, including:
1. Sample Size: Larger samples generally reduce the chance of making a Type II error because they provide more information about the population.
2. Effect Size: A larger effect size is easier to detect, reducing the risk of a Type II error.
3. Variability: Greater variability within the data can make it more difficult to detect an effect, increasing the chance of a Type II error.
4. Significance Level (α): A lower α increases the chance of a Type II error because it makes the test less sensitive to detecting true effects.
5. Power of the Test (1 - β): A higher powered test is less likely to make a Type II error.
Practical Examples:
- In a clinical trial, a Type I error might lead to the approval of an ineffective drug, causing harm to patients who receive it under the false belief of its efficacy.
- A Type II error in the same context might result in the non-approval of a genuinely effective drug, depriving patients of a beneficial treatment.
Statistical Tests and Errors:
Different statistical tests have varying sensitivities to these errors. For instance, tests with high power are less prone to Type II errors but might be more susceptible to Type I errors if not carefully calibrated.
Understanding and managing the risks of Type I and Type II errors is essential for researchers to make informed decisions based on data and to ensure the validity and reliability of their findings.
Type I Error (False Positive):
A Type I error occurs when the null hypothesis (H0) is incorrectly rejected. This means that there is a false detection of an effect or a relationship that does not actually exist. The null hypothesis typically represents the default position that there is no effect or no difference between groups. When a Type I error is made, the researcher concludes that there is a significant effect when in reality there is none.
The probability of making a Type I error is denoted by the Greek letter alpha (α), which is also known as the significance level of the test. This level is set by the researcher before conducting the study and is often set at 0.05, meaning there is a 5% chance of making a Type I error if the null hypothesis is true.
Type II Error (False Negative):
Conversely, a Type II error happens when the null hypothesis is incorrectly not rejected. This means that the researcher fails to detect an effect or a relationship that actually exists. In other words, a Type II error is like missing out on a true positive finding. The probability of making a Type II error is represented by the Greek letter beta (β), and the power of a test, which is the probability of correctly rejecting the null hypothesis when it is false (1 - β), is a key consideration in study design.
Balancing Type I and Type II Errors:
There is often a trade-off between the two types of errors. Reducing the likelihood of a Type I error (making the test more stringent) can increase the likelihood of a Type II error, and vice versa. This balance is critical in study design and is influenced by the consequences of each type of error. For example, in medical testing, a false positive might lead to unnecessary treatment, while a false negative could mean a missed diagnosis.
Factors Influencing Errors:
Several factors can influence the likelihood of committing Type I and Type II errors, including:
1. Sample Size: Larger samples generally reduce the chance of making a Type II error because they provide more information about the population.
2. Effect Size: A larger effect size is easier to detect, reducing the risk of a Type II error.
3. Variability: Greater variability within the data can make it more difficult to detect an effect, increasing the chance of a Type II error.
4. Significance Level (α): A lower α increases the chance of a Type II error because it makes the test less sensitive to detecting true effects.
5. Power of the Test (1 - β): A higher powered test is less likely to make a Type II error.
Practical Examples:
- In a clinical trial, a Type I error might lead to the approval of an ineffective drug, causing harm to patients who receive it under the false belief of its efficacy.
- A Type II error in the same context might result in the non-approval of a genuinely effective drug, depriving patients of a beneficial treatment.
Statistical Tests and Errors:
Different statistical tests have varying sensitivities to these errors. For instance, tests with high power are less prone to Type II errors but might be more susceptible to Type I errors if not carefully calibrated.
Understanding and managing the risks of Type I and Type II errors is essential for researchers to make informed decisions based on data and to ensure the validity and reliability of their findings.
2024-04-05 22:51:22
reply(1)
Helpful(1122)
Helpful
Helpful(2)
Studied at the University of Cape Town, Lives in Cape Town, South Africa.
Type I and type II errors. ... In statistical hypothesis testing, a type I error is the rejection of a true null hypothesis (also known as a "false positive" finding), while a type II error is retaining a false null hypothesis (also known as a "false negative" finding).
2023-06-21 07:20:19
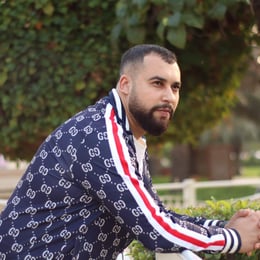
Julian Martinez
QuesHub.com delivers expert answers and knowledge to you.
Type I and type II errors. ... In statistical hypothesis testing, a type I error is the rejection of a true null hypothesis (also known as a "false positive" finding), while a type II error is retaining a false null hypothesis (also known as a "false negative" finding).