What is the value of alpha?
I'll answer
Earn 20 gold coins for an accepted answer.20
Earn 20 gold coins for an accepted answer.
40more
40more
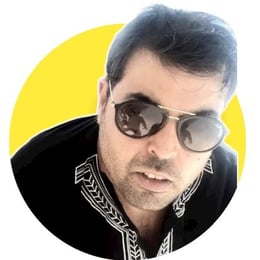
Ethan Turner
Works at the International Labour Organization, Lives in Geneva, Switzerland.
As an expert in statistical analysis, I'd like to clarify the concept of alpha level, which is a fundamental aspect of hypothesis testing. The alpha level, often denoted by the Greek letter α (alpha), is a threshold that helps researchers determine the statistical significance of their results. It is a critical parameter in the decision-making process of whether to reject or fail to reject the null hypothesis.
When conducting a statistical test, researchers set an alpha level before they start analyzing the data. This level is essentially the maximum acceptable probability of making a Type I error, which is the incorrect rejection of a true null hypothesis. In other words, if the p-value (the probability of observing the data given that the null hypothesis is true) is less than the alpha level, the null hypothesis is rejected, and the results are considered statistically significant.
The alpha level is typically set at 0.05, which means there is a 5% chance of committing a Type I error if the null hypothesis is true. However, researchers can choose a different alpha level based on the context of their study and the seriousness of the consequences of making a Type I error. For instance, in life-critical situations such as medical trials, a lower alpha level, such as 0.01 or even 0.001, might be used to minimize the risk of false positives.
It's important to note that the alpha level is not a measure of the strength of the evidence or the size of the effect. Instead, it is a threshold that defines the boundary between statistically significant and non-significant results. A statistically significant result does not necessarily imply a practically significant or meaningful effect.
Moreover, the choice of alpha level can have significant implications for the power of a test, which is the probability of correctly rejecting a false null hypothesis (1 - Type II error rate). A lower alpha level increases the stringency of the test and reduces the power, making it harder to detect true effects. Conversely, a higher alpha level increases the power but also increases the risk of Type I errors.
In summary, the alpha level is a crucial component in statistical testing that helps researchers balance the risks of Type I and Type II errors. It is a decision made before the analysis begins and is not adjusted based on the observed data. The alpha level should be chosen carefully, considering the specific context and consequences of the study.
When conducting a statistical test, researchers set an alpha level before they start analyzing the data. This level is essentially the maximum acceptable probability of making a Type I error, which is the incorrect rejection of a true null hypothesis. In other words, if the p-value (the probability of observing the data given that the null hypothesis is true) is less than the alpha level, the null hypothesis is rejected, and the results are considered statistically significant.
The alpha level is typically set at 0.05, which means there is a 5% chance of committing a Type I error if the null hypothesis is true. However, researchers can choose a different alpha level based on the context of their study and the seriousness of the consequences of making a Type I error. For instance, in life-critical situations such as medical trials, a lower alpha level, such as 0.01 or even 0.001, might be used to minimize the risk of false positives.
It's important to note that the alpha level is not a measure of the strength of the evidence or the size of the effect. Instead, it is a threshold that defines the boundary between statistically significant and non-significant results. A statistically significant result does not necessarily imply a practically significant or meaningful effect.
Moreover, the choice of alpha level can have significant implications for the power of a test, which is the probability of correctly rejecting a false null hypothesis (1 - Type II error rate). A lower alpha level increases the stringency of the test and reduces the power, making it harder to detect true effects. Conversely, a higher alpha level increases the power but also increases the risk of Type I errors.
In summary, the alpha level is a crucial component in statistical testing that helps researchers balance the risks of Type I and Type II errors. It is a decision made before the analysis begins and is not adjusted based on the observed data. The alpha level should be chosen carefully, considering the specific context and consequences of the study.
2024-04-18 02:19:13
reply(1)
Helpful(1122)
Helpful
Helpful(2)
Works at the International Maritime Organization, Lives in London, UK.
Before you run any statistical test, you must first determine your alpha level, which is also called the --significance level.-- By definition, the alpha level is the probability of rejecting the null hypothesis when the null hypothesis is true.Oct 1, 2012
2023-06-19 07:04:33
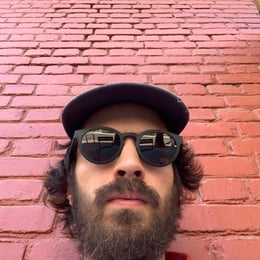
Charlotte Davis
QuesHub.com delivers expert answers and knowledge to you.
Before you run any statistical test, you must first determine your alpha level, which is also called the --significance level.-- By definition, the alpha level is the probability of rejecting the null hypothesis when the null hypothesis is true.Oct 1, 2012