What is a statistically significant difference?
I'll answer
Earn 20 gold coins for an accepted answer.20
Earn 20 gold coins for an accepted answer.
40more
40more

Charlotte Clark
Studied at the University of Lagos, Lives in Lagos, Nigeria.
As a statistical expert with a deep understanding of data analysis and interpretation, I often encounter the term "statistically significant difference." This concept is crucial in scientific research and decision-making processes where we need to determine if the observed differences between groups or over time are meaningful or just due to random variation.
When we talk about a statistically significant difference, we are referring to a scenario where two or more groups or conditions are compared, and we find a difference in their means, proportions, or other statistical measures. The key question is whether this difference is likely to have occurred by chance or if it's indicative of a real effect. To answer this, we use statistical tests that help us quantify the probability that the observed difference is due to random chance alone.
The null hypothesis (H0) is a fundamental concept in this context. It typically assumes that there is no difference between the groups or conditions being compared. The alternative hypothesis (H1), on the other hand, posits that there is a difference. Statistical tests are designed to evaluate the evidence against the null hypothesis.
To determine statistical significance, researchers set a significance level, often denoted as α (alpha), which is the probability of rejecting the null hypothesis when it is actually true (Type I error). A common significance level is 0.05, which means there is a 5% chance of observing a difference as extreme as, or more extreme than, the one observed if there truly is no difference. If the p-value, the probability of obtaining the observed results (or more extreme) under the assumption that the null hypothesis is true, is less than α, the result is considered statistically significant.
It's important to note that statistical significance does not imply practical significance. A statistically significant result means that the result is unlikely to be due to chance, but it does not necessarily mean that the result is large enough to be important in a real-world context. For this reason, researchers also consider the effect size, which measures the magnitude of the difference or the strength of the relationship.
Moreover, statistical significance can be influenced by the sample size. Larger sample sizes can lead to statistically significant results even for very small differences, which might not be meaningful in practice. This is known as the problem of **"statistical significance without practical significance"**.
In addition to the p-value, other measures such as confidence intervals provide a range within which the true population parameter is likely to fall. A 95% confidence interval, for instance, suggests that if we were to repeat the study many times, 95% of the confidence intervals calculated from the sample data would contain the true population parameter.
In conclusion, a statistically significant difference is a key concept in statistical analysis that helps researchers make inferences about populations from sample data. It is a measure of the likelihood that observed differences are not due to chance, but it must be interpreted in the context of the study's design, the effect size, and the practical implications of the findings.
When we talk about a statistically significant difference, we are referring to a scenario where two or more groups or conditions are compared, and we find a difference in their means, proportions, or other statistical measures. The key question is whether this difference is likely to have occurred by chance or if it's indicative of a real effect. To answer this, we use statistical tests that help us quantify the probability that the observed difference is due to random chance alone.
The null hypothesis (H0) is a fundamental concept in this context. It typically assumes that there is no difference between the groups or conditions being compared. The alternative hypothesis (H1), on the other hand, posits that there is a difference. Statistical tests are designed to evaluate the evidence against the null hypothesis.
To determine statistical significance, researchers set a significance level, often denoted as α (alpha), which is the probability of rejecting the null hypothesis when it is actually true (Type I error). A common significance level is 0.05, which means there is a 5% chance of observing a difference as extreme as, or more extreme than, the one observed if there truly is no difference. If the p-value, the probability of obtaining the observed results (or more extreme) under the assumption that the null hypothesis is true, is less than α, the result is considered statistically significant.
It's important to note that statistical significance does not imply practical significance. A statistically significant result means that the result is unlikely to be due to chance, but it does not necessarily mean that the result is large enough to be important in a real-world context. For this reason, researchers also consider the effect size, which measures the magnitude of the difference or the strength of the relationship.
Moreover, statistical significance can be influenced by the sample size. Larger sample sizes can lead to statistically significant results even for very small differences, which might not be meaningful in practice. This is known as the problem of **"statistical significance without practical significance"**.
In addition to the p-value, other measures such as confidence intervals provide a range within which the true population parameter is likely to fall. A 95% confidence interval, for instance, suggests that if we were to repeat the study many times, 95% of the confidence intervals calculated from the sample data would contain the true population parameter.
In conclusion, a statistically significant difference is a key concept in statistical analysis that helps researchers make inferences about populations from sample data. It is a measure of the likelihood that observed differences are not due to chance, but it must be interpreted in the context of the study's design, the effect size, and the practical implications of the findings.
2024-04-07 03:50:52
reply(1)
Helpful(1122)
Helpful
Helpful(2)
Studied at Harvard University, Lives in Cambridge, MA
A Significant Difference between two groups or two points in time means that there is a measurable difference between the groups and that, statistically, the probability of obtaining that difference by chance is very small (usually less than 5%).
2023-06-21 06:56:31
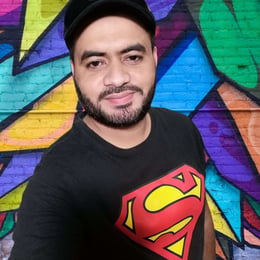
Julian Martin
QuesHub.com delivers expert answers and knowledge to you.
A Significant Difference between two groups or two points in time means that there is a measurable difference between the groups and that, statistically, the probability of obtaining that difference by chance is very small (usually less than 5%).