How does sample size affect Type 2 error?
I'll answer
Earn 20 gold coins for an accepted answer.20
Earn 20 gold coins for an accepted answer.
40more
40more

Olivia Davis
Studied at Princeton University, Lives in Princeton, NJ
As a statistical expert with extensive experience in hypothesis testing and statistical analysis, I am well-equipped to explain the intricate relationship between sample size and the probability of committing a Type II error. Type II error, denoted as \( \beta \), occurs when the null hypothesis is incorrectly not rejected when it is actually false. This is also known as a "false negative" in the context of hypothesis testing.
The sample size plays a pivotal role in the sensitivity of a hypothesis test. When the sample size is increased, the test becomes more sensitive to detecting the effect if it exists. This is because a larger sample size provides more information about the population, which in turn reduces the variability of the sample estimates. Here's a detailed breakdown of how sample size affects Type II error:
1. Reduction in Sampling Error: A larger sample size reduces the sampling error, which is the difference between the sample statistic and the population parameter. Since the sampling error decreases with an increase in sample size, the test statistic is more likely to accurately reflect the true state of the population.
2. Increased Statistical Power: The power of a test, denoted as \( 1 - \beta \), is the probability that the test correctly rejects a false null hypothesis. As the sample size increases, the power of the test also increases. This means that the test is more likely to detect an effect when there is one, thereby reducing the probability of a Type II error.
3. Effect Size and Variability: The ability to detect an effect (effect size) is influenced by the sample size. If the effect size is small, a larger sample size is needed to detect it with statistical significance. Additionally, if the population variability is high, a larger sample size can help to average out this variability and provide a clearer signal of the effect.
4. Level of Significance: The level of significance (denoted as \( \alpha \)) is the probability of committing a Type I error, which is the incorrect rejection of a true null hypothesis. While the level of significance is a separate concept from Type II error, it is important to note that it does not directly affect the probability of a Type II error. However, when designing a study, researchers often set a specific \( \alpha \) level and then determine the necessary sample size to achieve a desired power, which indirectly affects the risk of Type II error.
5. **Practical Significance vs. Statistical Significance**: A larger sample size can lead to statistically significant results that may not be practically significant. This is an important consideration when interpreting the results of a study. Even though a larger sample size reduces the risk of Type II error, it is also crucial to consider the magnitude and relevance of the effect in the context of the real-world application.
6. Cost and Feasibility: While increasing sample size has benefits, it also comes with increased costs and logistical challenges. Researchers must balance the need for a larger sample size to reduce the risk of Type II error with the practical constraints of their study.
7.
Sample Size Determination: Before conducting a study, researchers often use sample size calculations to determine the number of participants needed to achieve a certain power with a given effect size, level of significance, and expected variability in the data.
In conclusion, increasing the sample size generally makes a hypothesis test more sensitive, increasing the power of the test and reducing the probability of making a Type II error. It is a critical factor in the design of any study aiming to accurately assess the presence or absence of an effect in the population.
The sample size plays a pivotal role in the sensitivity of a hypothesis test. When the sample size is increased, the test becomes more sensitive to detecting the effect if it exists. This is because a larger sample size provides more information about the population, which in turn reduces the variability of the sample estimates. Here's a detailed breakdown of how sample size affects Type II error:
1. Reduction in Sampling Error: A larger sample size reduces the sampling error, which is the difference between the sample statistic and the population parameter. Since the sampling error decreases with an increase in sample size, the test statistic is more likely to accurately reflect the true state of the population.
2. Increased Statistical Power: The power of a test, denoted as \( 1 - \beta \), is the probability that the test correctly rejects a false null hypothesis. As the sample size increases, the power of the test also increases. This means that the test is more likely to detect an effect when there is one, thereby reducing the probability of a Type II error.
3. Effect Size and Variability: The ability to detect an effect (effect size) is influenced by the sample size. If the effect size is small, a larger sample size is needed to detect it with statistical significance. Additionally, if the population variability is high, a larger sample size can help to average out this variability and provide a clearer signal of the effect.
4. Level of Significance: The level of significance (denoted as \( \alpha \)) is the probability of committing a Type I error, which is the incorrect rejection of a true null hypothesis. While the level of significance is a separate concept from Type II error, it is important to note that it does not directly affect the probability of a Type II error. However, when designing a study, researchers often set a specific \( \alpha \) level and then determine the necessary sample size to achieve a desired power, which indirectly affects the risk of Type II error.
5. **Practical Significance vs. Statistical Significance**: A larger sample size can lead to statistically significant results that may not be practically significant. This is an important consideration when interpreting the results of a study. Even though a larger sample size reduces the risk of Type II error, it is also crucial to consider the magnitude and relevance of the effect in the context of the real-world application.
6. Cost and Feasibility: While increasing sample size has benefits, it also comes with increased costs and logistical challenges. Researchers must balance the need for a larger sample size to reduce the risk of Type II error with the practical constraints of their study.
7.
Sample Size Determination: Before conducting a study, researchers often use sample size calculations to determine the number of participants needed to achieve a certain power with a given effect size, level of significance, and expected variability in the data.
In conclusion, increasing the sample size generally makes a hypothesis test more sensitive, increasing the power of the test and reducing the probability of making a Type II error. It is a critical factor in the design of any study aiming to accurately assess the presence or absence of an effect in the population.
2024-04-14 01:28:14
reply(1)
Helpful(1122)
Helpful
Helpful(2)
Studied at the University of Johannesburg, Lives in Johannesburg, South Africa.
The correct answer is (A). Increasing sample size makes the hypothesis test more sensitive - more likely to reject the null hypothesis when it is, in fact, false. Thus, it increases the power of the test. ... And the probability of making a Type II error gets smaller, not bigger, as sample size increases.
2023-06-23 06:56:30
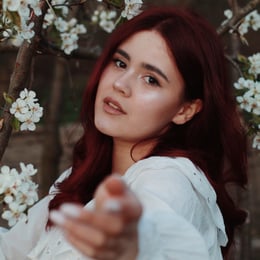
Charlotte Kim
QuesHub.com delivers expert answers and knowledge to you.
The correct answer is (A). Increasing sample size makes the hypothesis test more sensitive - more likely to reject the null hypothesis when it is, in fact, false. Thus, it increases the power of the test. ... And the probability of making a Type II error gets smaller, not bigger, as sample size increases.