What is a Type 3 error?
I'll answer
Earn 20 gold coins for an accepted answer.20
Earn 20 gold coins for an accepted answer.
40more
40more
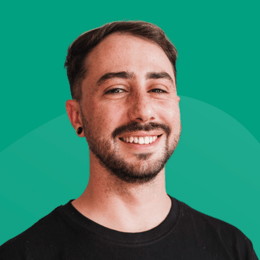
Alexander Wright
Works at Facebook, Lives in Menlo Park. Graduated from Massachusetts Institute of Technology (MIT) with a degree in Computer Science.
As an expert in statistical analysis and hypothesis testing, I'm often asked about different types of errors that can occur during these processes. One of the less commonly discussed, yet important, is the Type III error. It's a nuanced concept that doesn't get as much attention as its more famous counterparts, the Type I and Type II errors, but it's crucial for anyone conducting statistical analyses to understand.
### Understanding Hypothesis Testing
Before diving into the specifics of a Type III error, it's important to understand the basics of hypothesis testing. When we perform a statistical test, we're typically trying to determine whether there's evidence to support a certain claim or hypothesis. This claim is known as the null hypothesis (H0), and it's usually a statement of no effect or no difference. The alternative hypothesis (H1 or Ha) represents the opposite of the null hypothesis, suggesting an effect or a difference.
### Types of Errors in Hypothesis Testing
There are four main types of errors that can occur in hypothesis testing:
1. Type I Error: Also known as a false positive, this occurs when the null hypothesis is incorrectly rejected. It means we conclude there's an effect or difference when there isn't one.
2. Type II Error: Also known as a false negative, this happens when the null hypothesis is not rejected when it should have been. We fail to detect an effect or difference that actually exists.
3. Type III Error: This is where the concept starts to diverge from common understanding. A Type III error involves correctly rejecting the null hypothesis, but the reasoning behind the rejection is flawed or based on the wrong aspects of the data.
4. Type IV Error: This is less commonly discussed but refers to failing to detect a difference when there is one, but not due to the usual reasons associated with Type II errors.
### The Nature of Type III Error
A Type III error is particularly insidious because, on the surface, it appears that the correct decision has been made—the null hypothesis is rejected. However, the basis for this rejection is incorrect. This can occur when the test is sensitive to factors other than the one specified in the alternative hypothesis. For instance, if a study is designed to measure the effect of a new drug on blood pressure, but the rejection of the null hypothesis is actually due to an unaccounted factor like diet, then a Type III error has occurred.
### Consequences of Type III Errors
The consequences of a Type III error can be significant, especially in fields like medicine, where incorrect conclusions can lead to inappropriate treatments or interventions. It's also problematic in research, where it can lead to the publication of misleading results.
### Detecting and Avoiding Type III Errors
Detecting a Type III error can be challenging because, by definition, the null hypothesis is correctly rejected. However, a thorough understanding of the data and the context in which it was collected is crucial. Researchers should:
- Ensure the Test is Appropriate: The statistical test used should be appropriate for the research question and the type of data collected.
- Control Confounding Variables: It's important to identify and control for variables that could affect the outcome but are not the focus of the study.
- Use Multiple Measures: Employing a variety of statistical measures can help ensure that conclusions are not based on a single, potentially misleading, aspect of the data.
- Peer Review and Replication: Rigorous peer review and attempts at replication by other researchers can help identify and correct Type III errors.
### Conclusion
While a Type III error might not be as well-known as the other types of errors in hypothesis testing, it's a critical concept for anyone involved in statistical analysis. By understanding the nature of these errors and taking steps to avoid them, researchers can ensure that their conclusions are based on sound statistical reasoning and are more likely to reflect true effects or differences in the data.
### Understanding Hypothesis Testing
Before diving into the specifics of a Type III error, it's important to understand the basics of hypothesis testing. When we perform a statistical test, we're typically trying to determine whether there's evidence to support a certain claim or hypothesis. This claim is known as the null hypothesis (H0), and it's usually a statement of no effect or no difference. The alternative hypothesis (H1 or Ha) represents the opposite of the null hypothesis, suggesting an effect or a difference.
### Types of Errors in Hypothesis Testing
There are four main types of errors that can occur in hypothesis testing:
1. Type I Error: Also known as a false positive, this occurs when the null hypothesis is incorrectly rejected. It means we conclude there's an effect or difference when there isn't one.
2. Type II Error: Also known as a false negative, this happens when the null hypothesis is not rejected when it should have been. We fail to detect an effect or difference that actually exists.
3. Type III Error: This is where the concept starts to diverge from common understanding. A Type III error involves correctly rejecting the null hypothesis, but the reasoning behind the rejection is flawed or based on the wrong aspects of the data.
4. Type IV Error: This is less commonly discussed but refers to failing to detect a difference when there is one, but not due to the usual reasons associated with Type II errors.
### The Nature of Type III Error
A Type III error is particularly insidious because, on the surface, it appears that the correct decision has been made—the null hypothesis is rejected. However, the basis for this rejection is incorrect. This can occur when the test is sensitive to factors other than the one specified in the alternative hypothesis. For instance, if a study is designed to measure the effect of a new drug on blood pressure, but the rejection of the null hypothesis is actually due to an unaccounted factor like diet, then a Type III error has occurred.
### Consequences of Type III Errors
The consequences of a Type III error can be significant, especially in fields like medicine, where incorrect conclusions can lead to inappropriate treatments or interventions. It's also problematic in research, where it can lead to the publication of misleading results.
### Detecting and Avoiding Type III Errors
Detecting a Type III error can be challenging because, by definition, the null hypothesis is correctly rejected. However, a thorough understanding of the data and the context in which it was collected is crucial. Researchers should:
- Ensure the Test is Appropriate: The statistical test used should be appropriate for the research question and the type of data collected.
- Control Confounding Variables: It's important to identify and control for variables that could affect the outcome but are not the focus of the study.
- Use Multiple Measures: Employing a variety of statistical measures can help ensure that conclusions are not based on a single, potentially misleading, aspect of the data.
- Peer Review and Replication: Rigorous peer review and attempts at replication by other researchers can help identify and correct Type III errors.
### Conclusion
While a Type III error might not be as well-known as the other types of errors in hypothesis testing, it's a critical concept for anyone involved in statistical analysis. By understanding the nature of these errors and taking steps to avoid them, researchers can ensure that their conclusions are based on sound statistical reasoning and are more likely to reflect true effects or differences in the data.
2024-04-02 20:02:53
reply(1)
Helpful(1122)
Helpful
Helpful(2)
Studied at the University of Tokyo, Lives in Tokyo, Japan.
A type III error is where you correctly reject the null hypothesis, but it's rejected for the wrong reason. This compares to a Type I error (incorrectly rejecting the null hypothesis) and a Type II error (not rejecting the null when you should).Jan 16, 2015
2023-06-24 06:48:02
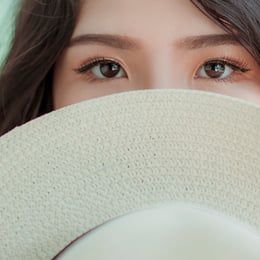
Madison Turner
QuesHub.com delivers expert answers and knowledge to you.
A type III error is where you correctly reject the null hypothesis, but it's rejected for the wrong reason. This compares to a Type I error (incorrectly rejecting the null hypothesis) and a Type II error (not rejecting the null when you should).Jan 16, 2015