What is the Cochran Mantel Haenszel test?
I'll answer
Earn 20 gold coins for an accepted answer.20
Earn 20 gold coins for an accepted answer.
40more
40more
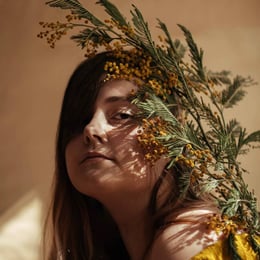
Amelia Taylor
Studied at Stanford University, Lives in Palo Alto, CA
In statistics, the Cochran-Mantel-Haenszel test (CMH) is a test used in the analysis of stratified or matched categorical data. It allows an investigator to test the association between a binary predictor or treatment and a binary outcome, such as case or control status, while taking into account the stratification.
The CMH test is particularly useful when you have data that is stratified by a third variable that could potentially confound the relationship between the predictor and the outcome. For example, in an epidemiological study, you might want to compare the incidence of a disease between two different treatments, but the age of the patients could be a confounding factor. By using the CMH test, you can control for age and other confounding variables to get a clearer picture of the effect of the treatment.
The test was developed by William G. Cochran and later extended by David G. Mantel and William Haenszel. It is a non-parametric test, which means it does not assume a specific distribution for the data. This makes it a versatile tool for analyzing categorical data.
### Assumptions
Before applying the CMH test, it is important to understand its assumptions:
1. Independence within Strata: Observations within each stratum should be independent of each other.
2. Stratification: The data should be stratified by a third variable that is a potential confounder.
3. Binary Outcomes: The outcome variable must be binary.
### Procedure
The CMH test involves the following steps:
1. Stratification: The data is divided into strata based on the confounding variable.
2. Contingency Table: For each stratum, a 2x2 contingency table is created with the rows representing the two levels of the predictor (e.g., treatment and control) and the columns representing the two levels of the outcome (e.g., presence or absence of the disease).
3. Calculation of Expected Frequencies: Using the marginal totals of the contingency tables, expected frequencies for each cell are calculated under the null hypothesis of no association.
4. Test Statistic: The test statistic is calculated based on the observed and expected frequencies. It is a measure of the deviation from the null hypothesis.
5. Degrees of Freedom: The degrees of freedom for the test are determined by the number of strata minus one.
6. Significance Level: The test statistic is compared to a chi-square distribution with the calculated degrees of freedom to determine the p-value.
### Interpretation
If the p-value is less than the chosen significance level (commonly 0.05), the null hypothesis of no association is rejected, indicating a statistically significant association between the predictor and the outcome, after adjusting for the stratification variable.
### Applications
The CMH test is widely used in various fields, including:
- Epidemiology: To control for confounding factors in observational studies.
- Clinical Trials: To analyze data when patients are matched or stratified by certain characteristics.
- Social Sciences: To examine relationships between categorical variables while controlling for other variables.
### Limitations
While the CMH test is a powerful tool, it also has some limitations:
- It may not be appropriate for data that are not well-stratified.
- The test assumes that the stratification variable is a confounder, not an intermediate variable in the causal pathway.
- It may not be the best choice for small sample sizes or when the expected frequencies in some cells are very low.
In conclusion, the Cochran-Mantel-Haenszel test is a valuable statistical method for analyzing stratified categorical data, allowing researchers to control for confounding variables and assess the association between a binary predictor and a binary outcome more accurately.
The CMH test is particularly useful when you have data that is stratified by a third variable that could potentially confound the relationship between the predictor and the outcome. For example, in an epidemiological study, you might want to compare the incidence of a disease between two different treatments, but the age of the patients could be a confounding factor. By using the CMH test, you can control for age and other confounding variables to get a clearer picture of the effect of the treatment.
The test was developed by William G. Cochran and later extended by David G. Mantel and William Haenszel. It is a non-parametric test, which means it does not assume a specific distribution for the data. This makes it a versatile tool for analyzing categorical data.
### Assumptions
Before applying the CMH test, it is important to understand its assumptions:
1. Independence within Strata: Observations within each stratum should be independent of each other.
2. Stratification: The data should be stratified by a third variable that is a potential confounder.
3. Binary Outcomes: The outcome variable must be binary.
### Procedure
The CMH test involves the following steps:
1. Stratification: The data is divided into strata based on the confounding variable.
2. Contingency Table: For each stratum, a 2x2 contingency table is created with the rows representing the two levels of the predictor (e.g., treatment and control) and the columns representing the two levels of the outcome (e.g., presence or absence of the disease).
3. Calculation of Expected Frequencies: Using the marginal totals of the contingency tables, expected frequencies for each cell are calculated under the null hypothesis of no association.
4. Test Statistic: The test statistic is calculated based on the observed and expected frequencies. It is a measure of the deviation from the null hypothesis.
5. Degrees of Freedom: The degrees of freedom for the test are determined by the number of strata minus one.
6. Significance Level: The test statistic is compared to a chi-square distribution with the calculated degrees of freedom to determine the p-value.
### Interpretation
If the p-value is less than the chosen significance level (commonly 0.05), the null hypothesis of no association is rejected, indicating a statistically significant association between the predictor and the outcome, after adjusting for the stratification variable.
### Applications
The CMH test is widely used in various fields, including:
- Epidemiology: To control for confounding factors in observational studies.
- Clinical Trials: To analyze data when patients are matched or stratified by certain characteristics.
- Social Sciences: To examine relationships between categorical variables while controlling for other variables.
### Limitations
While the CMH test is a powerful tool, it also has some limitations:
- It may not be appropriate for data that are not well-stratified.
- The test assumes that the stratification variable is a confounder, not an intermediate variable in the causal pathway.
- It may not be the best choice for small sample sizes or when the expected frequencies in some cells are very low.
In conclusion, the Cochran-Mantel-Haenszel test is a valuable statistical method for analyzing stratified categorical data, allowing researchers to control for confounding variables and assess the association between a binary predictor and a binary outcome more accurately.
2024-04-17 21:10:40
reply(1)
Helpful(1122)
Helpful
Helpful(2)
Works at the International Monetary Fund, Lives in Washington, D.C., USA.
In statistics, the Cochran-CMantel-CHaenszel test (CMH) is a test used in the analysis of stratified or matched categorical data. It allows an investigator to test the association between a binary predictor or treatment and a binary outcome such as case or control status while taking into account the stratification.
2023-06-23 06:39:50
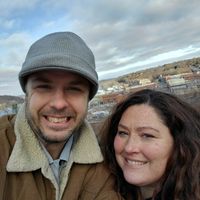
Isabella Garcia
QuesHub.com delivers expert answers and knowledge to you.
In statistics, the Cochran-CMantel-CHaenszel test (CMH) is a test used in the analysis of stratified or matched categorical data. It allows an investigator to test the association between a binary predictor or treatment and a binary outcome such as case or control status while taking into account the stratification.