What is Q in meta analysis?
I'll answer
Earn 20 gold coins for an accepted answer.20
Earn 20 gold coins for an accepted answer.
40more
40more
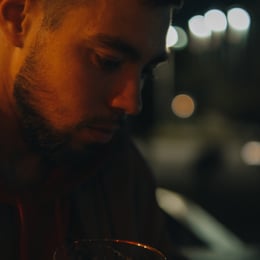
Harper Gonzalez
Studied at Stanford University, Lives in Palo Alto, CA
As a domain expert in statistical analysis, particularly in the realm of meta-analysis, I'm well-versed with the intricacies of combining study results from multiple sources. Meta-analysis is a statistical technique that combines the results of multiple independent studies to produce a single, more precise estimate of the effect size. It's a powerful tool for systematic reviews and evidence-based medicine, allowing researchers to synthesize findings across a range of investigations.
Now, let's delve into the concept of Q in meta-analysis.
Q, often referred to as Cochran's Q, is a statistical test used to assess the heterogeneity among study outcomes in a meta-analysis. Heterogeneity is the presence of variability in the outcomes of the studies that are being combined. It's a critical consideration because it can affect the validity of the pooled results. If the studies are too heterogeneous, it might not be appropriate to combine their results into a single estimate.
The Q statistic is calculated by comparing the weighted sum of the squared differences between each study's effect size and the overall pooled effect size, against a weighted sum of the squared differences within studies. The formula for Q is:
\[ Q = \sum (W_i \times (d_i - d_{pooled})^2) \]
Where:
- \( W_i \) is the weight assigned to the ith study.
- \( d_i \) is the effect size of the ith study.
- \( d_{pooled} \) is the pooled effect size from all studies.
A significant Q statistic suggests that there is heterogeneity among the study results, and the variability is greater than what would be expected by chance alone. However, it's important to note that Q has its limitations. As mentioned in the reference you provided, Q has low power as a comprehensive test of heterogeneity, especially when the number of studies is small, which is often the case in most meta-analyses (Gavaghan et al, 2000). This means that Q might not detect heterogeneity if the number of studies is too low, leading to a false sense of homogeneity.
Furthermore, the Q statistic is influenced by the size of the studies. Larger studies have a greater impact on the Q value, which can skew the perception of heterogeneity. This is why it's often complemented with the I² statistic, which provides an estimate of the percentage of variability in effect estimates that is due to heterogeneity rather than chance.
In conclusion, while Q is a foundational step in assessing heterogeneity, it should be interpreted with caution and in conjunction with other measures of heterogeneity to fully understand the variability among study outcomes in a meta-analysis.
Now, let's delve into the concept of Q in meta-analysis.
Q, often referred to as Cochran's Q, is a statistical test used to assess the heterogeneity among study outcomes in a meta-analysis. Heterogeneity is the presence of variability in the outcomes of the studies that are being combined. It's a critical consideration because it can affect the validity of the pooled results. If the studies are too heterogeneous, it might not be appropriate to combine their results into a single estimate.
The Q statistic is calculated by comparing the weighted sum of the squared differences between each study's effect size and the overall pooled effect size, against a weighted sum of the squared differences within studies. The formula for Q is:
\[ Q = \sum (W_i \times (d_i - d_{pooled})^2) \]
Where:
- \( W_i \) is the weight assigned to the ith study.
- \( d_i \) is the effect size of the ith study.
- \( d_{pooled} \) is the pooled effect size from all studies.
A significant Q statistic suggests that there is heterogeneity among the study results, and the variability is greater than what would be expected by chance alone. However, it's important to note that Q has its limitations. As mentioned in the reference you provided, Q has low power as a comprehensive test of heterogeneity, especially when the number of studies is small, which is often the case in most meta-analyses (Gavaghan et al, 2000). This means that Q might not detect heterogeneity if the number of studies is too low, leading to a false sense of homogeneity.
Furthermore, the Q statistic is influenced by the size of the studies. Larger studies have a greater impact on the Q value, which can skew the perception of heterogeneity. This is why it's often complemented with the I² statistic, which provides an estimate of the percentage of variability in effect estimates that is due to heterogeneity rather than chance.
In conclusion, while Q is a foundational step in assessing heterogeneity, it should be interpreted with caution and in conjunction with other measures of heterogeneity to fully understand the variability among study outcomes in a meta-analysis.
2024-04-02 00:15:34
reply(1)
Helpful(1122)
Helpful
Helpful(2)
Works at the International Fund for Agricultural Development, Lives in Rome, Italy.
Heterogeneity in meta-analysis refers to the variation in study outcomes between studies. ... Q has low power as a comprehensive test of heterogeneity (Gavaghan et al, 2000), especially when the number of studies is small, i.e. most meta-analyses.
2023-06-27 06:39:48

Grace Thompson
QuesHub.com delivers expert answers and knowledge to you.
Heterogeneity in meta-analysis refers to the variation in study outcomes between studies. ... Q has low power as a comprehensive test of heterogeneity (Gavaghan et al, 2000), especially when the number of studies is small, i.e. most meta-analyses.