What is t test and Anova test?
I'll answer
Earn 20 gold coins for an accepted answer.20
Earn 20 gold coins for an accepted answer.
40more
40more

Sophia Robinson
Studied at University of Oxford, Lives in Oxford, UK
Hello, I'm an expert in statistical analysis with a focus on hypothesis testing. Today, I'll be explaining two fundamental statistical tests: the t-test and the ANOVA test. Both of these tests are used to determine if there are statistically significant differences between groups, but they are applied in different scenarios.
The t-test
The t-test is a statistical method used to determine whether there is a significant difference between the means of two groups. It was developed by William Sealy Gosset under the pseudonym "Student" and is therefore also known as Student's t-test. The t-test is based on the t-distribution, which is a type of probability distribution that arises when estimating the population mean for a sample of a normally distributed population when the sample size is small. The t-test can be used in two forms: the one-sample t-test, which compares the mean of a single group to a known value (the population mean), and the two-sample t-test, which compares the means of two independent groups.
Key considerations for using a t-test include:
1. Independence: The observations in each group should be independent of each other.
2. Normality: The data should be approximately normally distributed. This assumption can be relaxed with large sample sizes due to the central limit theorem.
3. Equal Variance: The two-sample t-test assumes that the variances of the two groups are equal, although there are versions of the test (like Welch's t-test) that do not assume equal variances.
The formula for the t-test involves calculating the difference between the sample means, dividing it by the standard error of the difference, and then comparing this value to the critical value from the t-distribution.
The ANOVA test
ANOVA, or Analysis of Variance, is a statistical test that extends the concept of the t-test to compare more than two groups. It is used to determine if there are any statistically significant differences between the means of three or more groups. The term "variance" in ANOVA refers to the variation in the data, and the test essentially examines the amount of variation between groups compared to the variation within groups.
ANOVA works by partitioning the total variability in the data into two components: the variation between groups (the treatment effect) and the variation within groups (the error or individual variation). If the variation between groups is significantly larger than the variation within groups, it suggests that the groups are different from each other.
The basic steps in conducting an ANOVA include:
1. Hypothesis Formulation: Formulate null and alternative hypotheses. The null hypothesis typically states that there is no difference between the group means.
2. Data Collection: Collect data from each group.
3. Assumptions Check: Ensure that the assumptions of ANOVA are met, which include independence of observations, normality, and homogeneity of variances.
4. Calculation: Calculate the sum of squares for both between groups (SSB) and within groups (SSW), the degrees of freedom for each, and the mean squares (MSB and MSW).
5. F-Ratio: Compute the F-ratio, which is the ratio of MSB to MSW.
6. Significance Testing: Compare the calculated F-ratio to a critical value from the F-distribution to determine if the null hypothesis can be rejected.
If the F-ratio is large, it indicates that there is a significant amount of variation between the groups, and the null hypothesis can be rejected in favor of the alternative hypothesis that at least one group mean is different.
ANCOVA
ANCOVA, or Analysis of Covariance, is a more advanced statistical method that combines features of ANOVA with linear regression. It allows for the inclusion of covariates, which are continuous variables that might affect the dependent variable. By controlling for these covariates, ANCOVA can provide a more accurate assessment of the treatment effects.
In summary, the t-test and ANOVA are powerful tools for hypothesis testing in different scenarios. The t-test is used when comparing two groups, while ANOVA is the go-to test when dealing with three or more groups. ANCOVA offers a more nuanced approach by incorporating covariates into the analysis.
Now, let's proceed with the translation into Chinese.
The t-test
The t-test is a statistical method used to determine whether there is a significant difference between the means of two groups. It was developed by William Sealy Gosset under the pseudonym "Student" and is therefore also known as Student's t-test. The t-test is based on the t-distribution, which is a type of probability distribution that arises when estimating the population mean for a sample of a normally distributed population when the sample size is small. The t-test can be used in two forms: the one-sample t-test, which compares the mean of a single group to a known value (the population mean), and the two-sample t-test, which compares the means of two independent groups.
Key considerations for using a t-test include:
1. Independence: The observations in each group should be independent of each other.
2. Normality: The data should be approximately normally distributed. This assumption can be relaxed with large sample sizes due to the central limit theorem.
3. Equal Variance: The two-sample t-test assumes that the variances of the two groups are equal, although there are versions of the test (like Welch's t-test) that do not assume equal variances.
The formula for the t-test involves calculating the difference between the sample means, dividing it by the standard error of the difference, and then comparing this value to the critical value from the t-distribution.
The ANOVA test
ANOVA, or Analysis of Variance, is a statistical test that extends the concept of the t-test to compare more than two groups. It is used to determine if there are any statistically significant differences between the means of three or more groups. The term "variance" in ANOVA refers to the variation in the data, and the test essentially examines the amount of variation between groups compared to the variation within groups.
ANOVA works by partitioning the total variability in the data into two components: the variation between groups (the treatment effect) and the variation within groups (the error or individual variation). If the variation between groups is significantly larger than the variation within groups, it suggests that the groups are different from each other.
The basic steps in conducting an ANOVA include:
1. Hypothesis Formulation: Formulate null and alternative hypotheses. The null hypothesis typically states that there is no difference between the group means.
2. Data Collection: Collect data from each group.
3. Assumptions Check: Ensure that the assumptions of ANOVA are met, which include independence of observations, normality, and homogeneity of variances.
4. Calculation: Calculate the sum of squares for both between groups (SSB) and within groups (SSW), the degrees of freedom for each, and the mean squares (MSB and MSW).
5. F-Ratio: Compute the F-ratio, which is the ratio of MSB to MSW.
6. Significance Testing: Compare the calculated F-ratio to a critical value from the F-distribution to determine if the null hypothesis can be rejected.
If the F-ratio is large, it indicates that there is a significant amount of variation between the groups, and the null hypothesis can be rejected in favor of the alternative hypothesis that at least one group mean is different.
ANCOVA
ANCOVA, or Analysis of Covariance, is a more advanced statistical method that combines features of ANOVA with linear regression. It allows for the inclusion of covariates, which are continuous variables that might affect the dependent variable. By controlling for these covariates, ANCOVA can provide a more accurate assessment of the treatment effects.
In summary, the t-test and ANOVA are powerful tools for hypothesis testing in different scenarios. The t-test is used when comparing two groups, while ANOVA is the go-to test when dealing with three or more groups. ANCOVA offers a more nuanced approach by incorporating covariates into the analysis.
Now, let's proceed with the translation into Chinese.
2024-04-21 01:21:46
reply(1)
Helpful(1122)
Helpful
Helpful(2)
Works at the International Finance Corporation, Lives in Washington, D.C., USA.
The t-test and ANOVA examine whether group means differ from one another. The t-test compares two groups, while ANOVA can do more than two groups. ANCOVA (analysis of covariance) includes covariates, interval independent variables, in the right-hand side to control their impacts.Feb 14, 2016
2023-06-25 05:25:45
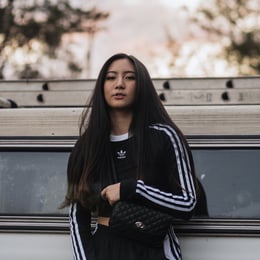
Harper Wright
QuesHub.com delivers expert answers and knowledge to you.
The t-test and ANOVA examine whether group means differ from one another. The t-test compares two groups, while ANOVA can do more than two groups. ANCOVA (analysis of covariance) includes covariates, interval independent variables, in the right-hand side to control their impacts.Feb 14, 2016