What is the difference between z test and t test?
I'll answer
Earn 20 gold coins for an accepted answer.20
Earn 20 gold coins for an accepted answer.
40more
40more
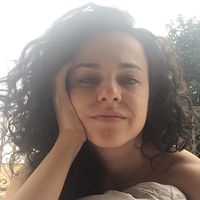
Isabella Hall
Studied at the University of Amsterdam, Lives in Amsterdam, Netherlands.
As a statistical expert with a strong background in data analysis, I have spent considerable time working with various statistical tests to determine the significance of findings in research studies. One of the most common questions that arise in the field is the distinction between the z-test and the t-test. Both are parametric tests used to determine if there is a significant difference between sample means and population means or between two sample means. However, they are used under different circumstances and have different underlying assumptions. Let's delve into the details:
### Z-Test
The z-test is used when you have a large sample size and you know the population standard deviation. It's based on the Z-distribution, which approaches the normal distribution as the sample size increases, due to the Central Limit Theorem. The Z-test is used for hypothesis testing when the following conditions are met:
1. Population Standard Deviation is Known: Unlike the t-test, which is used when the population standard deviation is unknown, the z-test requires this value to be known.
2. Sample Size is Large: Generally, a sample size of 30 or more is considered large enough for the z-test. This is because with large samples, the sampling distribution of the mean will be approximately normally distributed regardless of the population distribution.
3. Normal Distribution: The data should be normally distributed or the sample size should be large enough for the Central Limit Theorem to apply.
The formula for the z-test is as follows:
\[ z = \frac{\bar{x} - \mu}{\sigma / \sqrt{n}} \]
Where:
- \( \bar{x} \) is the sample mean,
- \( \mu \) is the population mean,
- \( \sigma \) is the known population standard deviation,
- \( n \) is the sample size.
### T-Test
On the other hand, the t-test is more appropriate when you have a small sample size and you **do not know the population standard deviation**. It is based on the t-distribution, which is similar to the normal distribution but has heavier tails, making it more forgiving of violations of the normality assumption, especially with smaller sample sizes.
The t-test is used under the following conditions:
1. Population Standard Deviation is Unknown: The t-test uses the sample standard deviation as an estimate for the population standard deviation.
2. Sample Size is Small: Typically, a sample size of less than 30 is considered small for the purposes of using a t-test.
3. Approximately Normal Distribution: The data should be approximately normally distributed or the sample should be large enough for the Central Limit Theorem to ensure that the sampling distribution of the mean is approximately normal.
The formula for the t-test is:
\[ t = \frac{\bar{x} - \mu}{s / \sqrt{n}} \]
Where:
- \( \bar{x} \) is the sample mean,
- \( \mu \) is the population mean,
- \( s \) is the sample standard deviation,
- \( n \) is the sample size.
### Key Differences
1. Population Standard Deviation: Z-test assumes it is known, while the t-test does not.
2. Sample Size: Z-test is used for larger samples, whereas t-test is used for smaller samples.
3. Distribution: Z-test relies on the normal distribution or a large sample size for normality, while t-test is more flexible due to the heavier tails of the t-distribution.
4. Test Statistic: The z-test uses the z-statistic, and the t-test uses the t-statistic, which adjusts for the uncertainty in estimating the population standard deviation from the sample.
### When to Use Each Test
- Use a z-test when you are comparing a sample mean to a known population mean and you have a large sample size or you know the population standard deviation.
- Use a t-test when comparing a sample mean to a population mean when the population standard deviation is unknown and you have a small sample size.
### Conclusion
Choosing between a z-test and a t-test is crucial for the validity of your statistical analysis. The decision is based on the sample size and the knowledge of the population standard deviation. Both tests are parametric and assume normality, but the t-test is more robust to violations of this assumption due to its use with smaller sample sizes.
Now, let's proceed with the translation into Chinese.
### Z-Test
The z-test is used when you have a large sample size and you know the population standard deviation. It's based on the Z-distribution, which approaches the normal distribution as the sample size increases, due to the Central Limit Theorem. The Z-test is used for hypothesis testing when the following conditions are met:
1. Population Standard Deviation is Known: Unlike the t-test, which is used when the population standard deviation is unknown, the z-test requires this value to be known.
2. Sample Size is Large: Generally, a sample size of 30 or more is considered large enough for the z-test. This is because with large samples, the sampling distribution of the mean will be approximately normally distributed regardless of the population distribution.
3. Normal Distribution: The data should be normally distributed or the sample size should be large enough for the Central Limit Theorem to apply.
The formula for the z-test is as follows:
\[ z = \frac{\bar{x} - \mu}{\sigma / \sqrt{n}} \]
Where:
- \( \bar{x} \) is the sample mean,
- \( \mu \) is the population mean,
- \( \sigma \) is the known population standard deviation,
- \( n \) is the sample size.
### T-Test
On the other hand, the t-test is more appropriate when you have a small sample size and you **do not know the population standard deviation**. It is based on the t-distribution, which is similar to the normal distribution but has heavier tails, making it more forgiving of violations of the normality assumption, especially with smaller sample sizes.
The t-test is used under the following conditions:
1. Population Standard Deviation is Unknown: The t-test uses the sample standard deviation as an estimate for the population standard deviation.
2. Sample Size is Small: Typically, a sample size of less than 30 is considered small for the purposes of using a t-test.
3. Approximately Normal Distribution: The data should be approximately normally distributed or the sample should be large enough for the Central Limit Theorem to ensure that the sampling distribution of the mean is approximately normal.
The formula for the t-test is:
\[ t = \frac{\bar{x} - \mu}{s / \sqrt{n}} \]
Where:
- \( \bar{x} \) is the sample mean,
- \( \mu \) is the population mean,
- \( s \) is the sample standard deviation,
- \( n \) is the sample size.
### Key Differences
1. Population Standard Deviation: Z-test assumes it is known, while the t-test does not.
2. Sample Size: Z-test is used for larger samples, whereas t-test is used for smaller samples.
3. Distribution: Z-test relies on the normal distribution or a large sample size for normality, while t-test is more flexible due to the heavier tails of the t-distribution.
4. Test Statistic: The z-test uses the z-statistic, and the t-test uses the t-statistic, which adjusts for the uncertainty in estimating the population standard deviation from the sample.
### When to Use Each Test
- Use a z-test when you are comparing a sample mean to a known population mean and you have a large sample size or you know the population standard deviation.
- Use a t-test when comparing a sample mean to a population mean when the population standard deviation is unknown and you have a small sample size.
### Conclusion
Choosing between a z-test and a t-test is crucial for the validity of your statistical analysis. The decision is based on the sample size and the knowledge of the population standard deviation. Both tests are parametric and assume normality, but the t-test is more robust to violations of this assumption due to its use with smaller sample sizes.
Now, let's proceed with the translation into Chinese.
2024-04-11 13:45:55
reply(1)
Helpful(1122)
Helpful
Helpful(2)
Works at the International Fund for Agricultural Development, Lives in Rome, Italy.
A t-test is used for testing the mean of one population against a standard or comparing the means of two populations if you do not know the populations' standard deviation and when you have a limited sample (n < 30). If you know the populations' standard deviation, you may use a z-test.Dec 5, 2010
2023-06-24 05:25:36
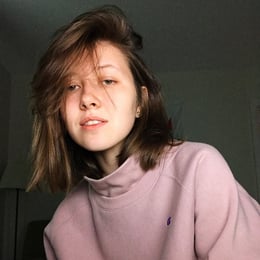
Lily Gonzales
QuesHub.com delivers expert answers and knowledge to you.
A t-test is used for testing the mean of one population against a standard or comparing the means of two populations if you do not know the populations' standard deviation and when you have a limited sample (n < 30). If you know the populations' standard deviation, you may use a z-test.Dec 5, 2010