Can you have a P value greater than 1?
I'll answer
Earn 20 gold coins for an accepted answer.20
Earn 20 gold coins for an accepted answer.
40more
40more
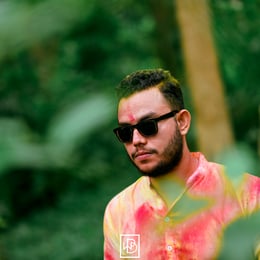
Oliver Patel
Works at the International Organization for Migration, Lives in Geneva, Switzerland.
As a domain expert in statistics, I often encounter questions regarding the interpretation and understanding of statistical terms. One such term is the "p-value," which is a crucial concept in hypothesis testing. Let's delve into the intricacies of p-values and address the question of whether a p-value can be greater than 1.
P-values are used to measure the strength of the evidence against a null hypothesis. They are calculated under the assumption that the null hypothesis is true. The null hypothesis is a statement that there is no effect or no relationship between variables. For example, in a drug trial, the null hypothesis might be that the new drug has no effect on patients' health compared to a placebo.
When conducting a statistical test, we compare the observed data to what we would expect under the null hypothesis. The p-value is the probability of obtaining data as extreme as, or more extreme than, our observed data if the null hypothesis were true. It's important to note that a p-value does not measure the probability that the null hypothesis is true or false; rather, it measures the compatibility of the data with the null hypothesis.
Now, let's consider the possibility of a p-value being greater than 1. According to the definition of a p-value, it is the probability of observing a result at least as extreme as the one calculated under the null hypothesis. By definition, probabilities range from 0 to 1, where 0 indicates an impossible event and 1 indicates a certain event. Therefore, a p-value greater than 1 would imply a probability greater than 100%, which is logically and mathematically impossible.
In practice, if you encounter a p-value greater than 1, it is likely due to an error in the calculation or an incorrect understanding of the data. It could also be a result of a flawed statistical model or an inappropriate application of the statistical test. It's crucial to double-check the methodology and the assumptions underlying the statistical test to ensure that the p-value is calculated correctly.
Furthermore, when interpreting p-values, it's essential to consider the context and the magnitude of the p-value in relation to the significance level (α), which is a threshold set by the researcher before conducting the test. Commonly used significance levels are 0.05, 0.01, and 0.001. If the p-value is less than the significance level, it suggests that there is strong evidence against the null hypothesis, and we might reject it in favor of the alternative hypothesis. Conversely, if the p-value is greater than the significance level, we do not have enough evidence to reject the null hypothesis.
In conclusion, a p-value cannot be greater than 1 because it represents a probability, and probabilities are bounded between 0 and 1. A p-value greater than 1 would defy the fundamental principles of probability theory and statistical inference. It's essential for researchers and practitioners to have a solid understanding of p-values and their proper use in hypothesis testing to avoid misinterpretations and draw accurate conclusions from their data.
P-values are used to measure the strength of the evidence against a null hypothesis. They are calculated under the assumption that the null hypothesis is true. The null hypothesis is a statement that there is no effect or no relationship between variables. For example, in a drug trial, the null hypothesis might be that the new drug has no effect on patients' health compared to a placebo.
When conducting a statistical test, we compare the observed data to what we would expect under the null hypothesis. The p-value is the probability of obtaining data as extreme as, or more extreme than, our observed data if the null hypothesis were true. It's important to note that a p-value does not measure the probability that the null hypothesis is true or false; rather, it measures the compatibility of the data with the null hypothesis.
Now, let's consider the possibility of a p-value being greater than 1. According to the definition of a p-value, it is the probability of observing a result at least as extreme as the one calculated under the null hypothesis. By definition, probabilities range from 0 to 1, where 0 indicates an impossible event and 1 indicates a certain event. Therefore, a p-value greater than 1 would imply a probability greater than 100%, which is logically and mathematically impossible.
In practice, if you encounter a p-value greater than 1, it is likely due to an error in the calculation or an incorrect understanding of the data. It could also be a result of a flawed statistical model or an inappropriate application of the statistical test. It's crucial to double-check the methodology and the assumptions underlying the statistical test to ensure that the p-value is calculated correctly.
Furthermore, when interpreting p-values, it's essential to consider the context and the magnitude of the p-value in relation to the significance level (α), which is a threshold set by the researcher before conducting the test. Commonly used significance levels are 0.05, 0.01, and 0.001. If the p-value is less than the significance level, it suggests that there is strong evidence against the null hypothesis, and we might reject it in favor of the alternative hypothesis. Conversely, if the p-value is greater than the significance level, we do not have enough evidence to reject the null hypothesis.
In conclusion, a p-value cannot be greater than 1 because it represents a probability, and probabilities are bounded between 0 and 1. A p-value greater than 1 would defy the fundamental principles of probability theory and statistical inference. It's essential for researchers and practitioners to have a solid understanding of p-values and their proper use in hypothesis testing to avoid misinterpretations and draw accurate conclusions from their data.
2024-04-24 23:52:28
reply(1)
Helpful(1122)
Helpful
Helpful(2)
Works at the International Renewable Energy Agency, Lives in Abu Dhabi, UAE.
Explanation: A p-value tells you the probability of having a result that is equal to or greater than the result you achieved under your specific hypothesis. ... A p-value higher than one would mean a probability greater than 100% and this can't occur.Mar 21, 2017
2023-06-27 05:25:32
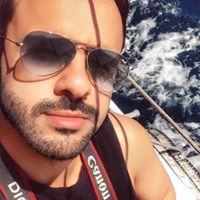
Lincoln Brown
QuesHub.com delivers expert answers and knowledge to you.
Explanation: A p-value tells you the probability of having a result that is equal to or greater than the result you achieved under your specific hypothesis. ... A p-value higher than one would mean a probability greater than 100% and this can't occur.Mar 21, 2017