When you reject the null hypothesis Do you accept the alternative?
I'll answer
Earn 20 gold coins for an accepted answer.20
Earn 20 gold coins for an accepted answer.
40more
40more
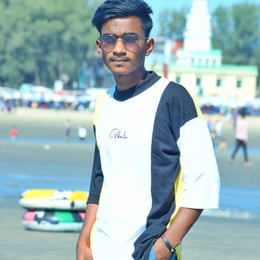
Ethan Campbell
Works at the International Criminal Police Organization (INTERPOL), Lives in Lyon, France.
As a statistical expert, I'd like to clarify the concept of hypothesis testing, which is a fundamental procedure in inferential statistics. When we conduct a hypothesis test, we are essentially making a decision based on the evidence provided by the data. The null hypothesis, denoted as \( H_0 \), represents the default assumption that there is no effect or no difference between groups. The alternative hypothesis, denoted as \( H_1 \) or \( H_a \), is what we consider if the null hypothesis is rejected; it represents the claim that there is an effect or a difference.
The process of hypothesis testing involves calculating a test statistic based on the sample data and comparing it to a critical value from a statistical distribution. If the test statistic is more extreme than the critical value, we say that the result is statistically significant, and we reject the null hypothesis in favor of the alternative hypothesis. However, the decision to reject the null hypothesis is not a definitive proof that the alternative hypothesis is true; rather, it indicates that the data are inconsistent with the null hypothesis at a given level of significance.
The significance level, often denoted as \( \alpha \), is the threshold we set for deciding when to reject the null hypothesis. Commonly used significance levels are 0.05 and 0.01, which means we are willing to accept a 5% or 1% chance, respectively, of rejecting a true null hypothesis (Type I error). If the p-value, which is the probability of observing a test statistic as extreme as, or more extreme than, the one calculated from our sample if the null hypothesis were true, is less than \( \alpha \), we reject the null hypothesis.
It's important to note that failing to reject the null hypothesis does not mean that the null hypothesis is true. It simply means that the data do not provide sufficient evidence to reject it at the chosen significance level. This is known as a failure to reject \( H_0 \), and it could be due to a lack of statistical power, which is the probability that a test will reject a false null hypothesis (Type II error).
In conclusion, hypothesis testing is a critical tool in statistical analysis, but it is not infallible. It is subject to various limitations and assumptions that must be considered when interpreting the results. The decision to reject or fail to reject the null hypothesis is based on the evidence provided by the data and the significance level chosen by the researcher.
The process of hypothesis testing involves calculating a test statistic based on the sample data and comparing it to a critical value from a statistical distribution. If the test statistic is more extreme than the critical value, we say that the result is statistically significant, and we reject the null hypothesis in favor of the alternative hypothesis. However, the decision to reject the null hypothesis is not a definitive proof that the alternative hypothesis is true; rather, it indicates that the data are inconsistent with the null hypothesis at a given level of significance.
The significance level, often denoted as \( \alpha \), is the threshold we set for deciding when to reject the null hypothesis. Commonly used significance levels are 0.05 and 0.01, which means we are willing to accept a 5% or 1% chance, respectively, of rejecting a true null hypothesis (Type I error). If the p-value, which is the probability of observing a test statistic as extreme as, or more extreme than, the one calculated from our sample if the null hypothesis were true, is less than \( \alpha \), we reject the null hypothesis.
It's important to note that failing to reject the null hypothesis does not mean that the null hypothesis is true. It simply means that the data do not provide sufficient evidence to reject it at the chosen significance level. This is known as a failure to reject \( H_0 \), and it could be due to a lack of statistical power, which is the probability that a test will reject a false null hypothesis (Type II error).
In conclusion, hypothesis testing is a critical tool in statistical analysis, but it is not infallible. It is subject to various limitations and assumptions that must be considered when interpreting the results. The decision to reject or fail to reject the null hypothesis is based on the evidence provided by the data and the significance level chosen by the researcher.
2024-04-22 17:14:21
reply(1)
Helpful(1122)
Helpful
Helpful(2)
Studied at the University of São Paulo, Lives in São Paulo, Brazil.
Let's return finally to the question of whether we reject or fail to reject the null hypothesis. If our statistical analysis shows that the significance level is below the cut-off value we have set (e.g., either 0.05 or 0.01), we reject the null hypothesis and accept the alternative hypothesis.
2023-06-25 05:20:48

Amelia Thomas
QuesHub.com delivers expert answers and knowledge to you.
Let's return finally to the question of whether we reject or fail to reject the null hypothesis. If our statistical analysis shows that the significance level is below the cut-off value we have set (e.g., either 0.05 or 0.01), we reject the null hypothesis and accept the alternative hypothesis.