What is the h0 for?
I'll answer
Earn 20 gold coins for an accepted answer.20
Earn 20 gold coins for an accepted answer.
40more
40more
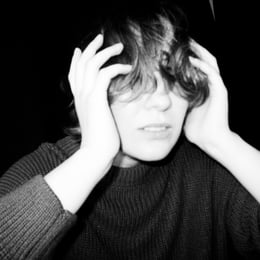
Sophia Lee
Studied at the University of Adelaide, Lives in Adelaide, Australia.
As a field expert in statistical analysis, I can provide you with a comprehensive understanding of the null hypothesis, often denoted as \( H_0 \), and its role in hypothesis testing, which is a fundamental aspect of scientific inquiry and statistical inference.
The null hypothesis is a crucial concept in the realm of statistics. It is a statement that assumes there is no effect, no difference, or no association between variables. It serves as the starting point for statistical testing and is the default position that researchers assume to be true before conducting an experiment or study. The null hypothesis is tested against an alternative hypothesis, denoted as \( H_a \) or \( H_1 \), which posits the opposite—that there is an effect, a difference, or an association.
The process of hypothesis testing involves collecting data and using statistical methods to determine whether the results are consistent with the null hypothesis or if they suggest that the alternative hypothesis is more likely to be true. If the data provide strong evidence against the null hypothesis, it is rejected in favor of the alternative hypothesis. However, failure to reject the null hypothesis does not prove it to be true; it simply means that the evidence is not sufficient to conclude otherwise.
The null hypothesis is formulated to be testable and falsifiable. It is typically stated in a way that allows for a clear, quantitative assessment. For example, in an experiment testing the effectiveness of a new drug, the null hypothesis might be that the drug has no effect on the condition being treated, while the alternative hypothesis would be that the drug does have an effect.
The significance level, often denoted by \( \alpha \), is a predetermined threshold that determines when the null hypothesis will be rejected. If the p-value, which is the probability of observing the data given that the null hypothesis is true, is less than \( \alpha \), the null hypothesis is rejected. Common significance levels are 0.05, 0.01, and 0.001.
It's important to note that the null hypothesis is a working assumption rather than a statement of absolute truth. Researchers often have a specific direction in mind for their research question, which is reflected in the alternative hypothesis. For instance, a researcher might hope to demonstrate that a new teaching method improves student performance, which would be stated as the alternative hypothesis.
The type I error occurs when the null hypothesis is rejected when it is actually true. This is a false positive and its probability is equal to the significance level \( \alpha \). Conversely, a type II error occurs when the null hypothesis is not rejected when it is false. This is a false negative, and its probability is denoted by \( \beta \), with the power of the test (1 - \( \beta \)) indicating the probability of correctly rejecting a false null hypothesis.
In summary, the null hypothesis is a foundational concept in statistical testing that allows researchers to systematically evaluate evidence and make inferences about the population from which their sample is drawn. It is a statement of no effect or no difference that is tested against an alternative hypothesis, with the decision to reject or fail to reject based on the data collected and the chosen significance level.
The null hypothesis is a crucial concept in the realm of statistics. It is a statement that assumes there is no effect, no difference, or no association between variables. It serves as the starting point for statistical testing and is the default position that researchers assume to be true before conducting an experiment or study. The null hypothesis is tested against an alternative hypothesis, denoted as \( H_a \) or \( H_1 \), which posits the opposite—that there is an effect, a difference, or an association.
The process of hypothesis testing involves collecting data and using statistical methods to determine whether the results are consistent with the null hypothesis or if they suggest that the alternative hypothesis is more likely to be true. If the data provide strong evidence against the null hypothesis, it is rejected in favor of the alternative hypothesis. However, failure to reject the null hypothesis does not prove it to be true; it simply means that the evidence is not sufficient to conclude otherwise.
The null hypothesis is formulated to be testable and falsifiable. It is typically stated in a way that allows for a clear, quantitative assessment. For example, in an experiment testing the effectiveness of a new drug, the null hypothesis might be that the drug has no effect on the condition being treated, while the alternative hypothesis would be that the drug does have an effect.
The significance level, often denoted by \( \alpha \), is a predetermined threshold that determines when the null hypothesis will be rejected. If the p-value, which is the probability of observing the data given that the null hypothesis is true, is less than \( \alpha \), the null hypothesis is rejected. Common significance levels are 0.05, 0.01, and 0.001.
It's important to note that the null hypothesis is a working assumption rather than a statement of absolute truth. Researchers often have a specific direction in mind for their research question, which is reflected in the alternative hypothesis. For instance, a researcher might hope to demonstrate that a new teaching method improves student performance, which would be stated as the alternative hypothesis.
The type I error occurs when the null hypothesis is rejected when it is actually true. This is a false positive and its probability is equal to the significance level \( \alpha \). Conversely, a type II error occurs when the null hypothesis is not rejected when it is false. This is a false negative, and its probability is denoted by \( \beta \), with the power of the test (1 - \( \beta \)) indicating the probability of correctly rejecting a false null hypothesis.
In summary, the null hypothesis is a foundational concept in statistical testing that allows researchers to systematically evaluate evidence and make inferences about the population from which their sample is drawn. It is a statement of no effect or no difference that is tested against an alternative hypothesis, with the decision to reject or fail to reject based on the data collected and the chosen significance level.
2024-04-06 07:17:47
reply(1)
Helpful(1122)
Helpful
Helpful(2)
Studied at the University of Oxford, Lives in Oxford, UK.
The null hypothesis (H0) is a statement of --no difference,-- --no association,-- or --no treatment effect.-- ? The alternative hypothesis, Ha is a statement of --difference,-- --association,-- or --treatment effect.-- H0 is assumed to be true until proven otherwise. However, Ha is the hypothesis the researcher hopes to bolster.
2023-06-22 04:25:50

Scarlett Adams
QuesHub.com delivers expert answers and knowledge to you.
The null hypothesis (H0) is a statement of --no difference,-- --no association,-- or --no treatment effect.-- ? The alternative hypothesis, Ha is a statement of --difference,-- --association,-- or --treatment effect.-- H0 is assumed to be true until proven otherwise. However, Ha is the hypothesis the researcher hopes to bolster.