What does the t test tell us?
I'll answer
Earn 20 gold coins for an accepted answer.20
Earn 20 gold coins for an accepted answer.
40more
40more
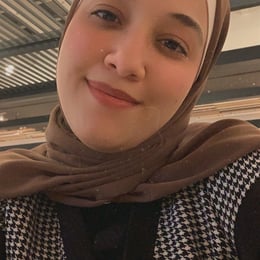
Amelia Patel
Studied at the University of Oxford, Lives in Oxford, UK.
As a domain expert in statistical analysis, I can tell you that the t-test is a statistical method used to determine whether there are significant differences between the means of two groups. It was developed by William Sealy Gosset under the pseudonym "Student", which is why it is often referred to as Student's t-test. The t-test is a type of parametric test, which means it makes certain assumptions about the data, such as the distribution of the data and the variances of the groups being compared.
When you perform a t-test, you're usually trying to find evidence of a significant difference between population means (2-sample t) or between the population mean and a hypothesized value (1-sample t). The t-value measures the size of the difference relative to the variation in your sample data.
Here are the key points that the t-test tells us:
1. Hypothesis Testing: The t-test begins with the formulation of a null hypothesis (H0) and an alternative hypothesis (H1). The null hypothesis typically states that there is no difference between the means of the groups, while the alternative hypothesis suggests that there is a difference.
2. Degrees of Freedom: The t-test takes into account the degrees of freedom, which is the number of values that are free to vary in the calculation of the statistic. For a 2-sample t-test, the degrees of freedom is calculated as the sum of the sample sizes of the two groups minus two.
3. t-Value: The t-value is calculated from the sample data and represents the number of standard errors that the sample mean is away from the hypothesized mean. A larger t-value indicates a greater difference between the sample mean and the hypothesized mean.
4. Significance Level (α): This is the probability of rejecting the null hypothesis when it is actually true. Commonly used significance levels are 0.05, 0.01, and 0.001.
5. p-Value: The p-value is the probability of observing a t-value as extreme as, or more extreme than, the one calculated from the sample data, assuming that the null hypothesis is true. If the p-value is less than the significance level, you reject the null hypothesis in favor of the alternative hypothesis.
6. Confidence Intervals: The t-test can also be used to construct confidence intervals for the difference between means. These intervals provide a range within which the true difference between population means is likely to fall.
7.
Assumptions: The t-test assumes that the data are normally distributed and that the variances of the groups being compared are equal (for the standard t-test). If these assumptions are not met, the results of the t-test may not be valid.
8.
Effect Size: While the t-test can tell you if there is a statistically significant difference, it does not tell you how large that difference is. The effect size is a measure of the magnitude of the difference between groups.
9.
Power of the Test: The power of a t-test is the probability that it will correctly reject a false null hypothesis. A higher power is desirable, as it reduces the chance of a Type II error (failing to reject a false null hypothesis).
10.
One-Tailed vs. Two-Tailed Tests: Depending on the research question, you may conduct a one-tailed test, which tests for a difference in a specific direction, or a two-tailed test, which tests for a difference in either direction.
In conclusion, the t-test is a powerful tool for determining whether there are statistically significant differences between groups. It provides a way to test hypotheses, calculate the likelihood of observing the data under the null hypothesis, and construct confidence intervals. However, it is important to remember the assumptions underlying the test and to interpret the results in the context of the research question and the data being analyzed.
When you perform a t-test, you're usually trying to find evidence of a significant difference between population means (2-sample t) or between the population mean and a hypothesized value (1-sample t). The t-value measures the size of the difference relative to the variation in your sample data.
Here are the key points that the t-test tells us:
1. Hypothesis Testing: The t-test begins with the formulation of a null hypothesis (H0) and an alternative hypothesis (H1). The null hypothesis typically states that there is no difference between the means of the groups, while the alternative hypothesis suggests that there is a difference.
2. Degrees of Freedom: The t-test takes into account the degrees of freedom, which is the number of values that are free to vary in the calculation of the statistic. For a 2-sample t-test, the degrees of freedom is calculated as the sum of the sample sizes of the two groups minus two.
3. t-Value: The t-value is calculated from the sample data and represents the number of standard errors that the sample mean is away from the hypothesized mean. A larger t-value indicates a greater difference between the sample mean and the hypothesized mean.
4. Significance Level (α): This is the probability of rejecting the null hypothesis when it is actually true. Commonly used significance levels are 0.05, 0.01, and 0.001.
5. p-Value: The p-value is the probability of observing a t-value as extreme as, or more extreme than, the one calculated from the sample data, assuming that the null hypothesis is true. If the p-value is less than the significance level, you reject the null hypothesis in favor of the alternative hypothesis.
6. Confidence Intervals: The t-test can also be used to construct confidence intervals for the difference between means. These intervals provide a range within which the true difference between population means is likely to fall.
7.
Assumptions: The t-test assumes that the data are normally distributed and that the variances of the groups being compared are equal (for the standard t-test). If these assumptions are not met, the results of the t-test may not be valid.
8.
Effect Size: While the t-test can tell you if there is a statistically significant difference, it does not tell you how large that difference is. The effect size is a measure of the magnitude of the difference between groups.
9.
Power of the Test: The power of a t-test is the probability that it will correctly reject a false null hypothesis. A higher power is desirable, as it reduces the chance of a Type II error (failing to reject a false null hypothesis).
10.
One-Tailed vs. Two-Tailed Tests: Depending on the research question, you may conduct a one-tailed test, which tests for a difference in a specific direction, or a two-tailed test, which tests for a difference in either direction.
In conclusion, the t-test is a powerful tool for determining whether there are statistically significant differences between groups. It provides a way to test hypotheses, calculate the likelihood of observing the data under the null hypothesis, and construct confidence intervals. However, it is important to remember the assumptions underlying the test and to interpret the results in the context of the research question and the data being analyzed.
2024-04-26 13:37:38
reply(1)
Helpful(1122)
Helpful
Helpful(2)
Studied at Stanford University, Lives in Palo Alto, CA
When you perform a t-test, you're usually trying to find evidence of a significant difference between population means (2-sample t) or between the population mean and a hypothesized value (1-sample t). The t-value measures the size of the difference relative to the variation in your sample data.Nov 4, 2016
2023-06-24 04:17:54

Ethan Wilson
QuesHub.com delivers expert answers and knowledge to you.
When you perform a t-test, you're usually trying to find evidence of a significant difference between population means (2-sample t) or between the population mean and a hypothesized value (1-sample t). The t-value measures the size of the difference relative to the variation in your sample data.Nov 4, 2016