What is hypothesis testing in research methodology 2024?
I'll answer
Earn 20 gold coins for an accepted answer.20
Earn 20 gold coins for an accepted answer.
40more
40more
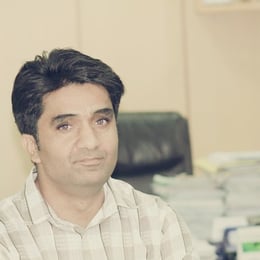
Oliver Gray
Works at the International Criminal Court, Lives in The Hague, Netherlands.
As a research methodology expert with a background in statistics and data analysis, I often delve into the intricacies of hypothesis testing. This is a fundamental concept in the field of research, particularly in quantitative studies where empirical evidence is sought to support or refute a claim or theory.
**Hypothesis Testing in Research Methodology**
Hypothesis testing is a statistical method used to make decisions or draw conclusions about a population based on sample data. It's a systematic process that allows researchers to evaluate whether the results of their study are likely due to chance or if there is a significant relationship between variables. The process involves setting up a null hypothesis (H0) and an alternative hypothesis (H1), which are competing statements about the population parameter.
1. Formulating the Hypotheses: The first step is to clearly state the research question and formulate the null and alternative hypotheses. The null hypothesis typically represents the status quo or the assumption of no effect or no difference, while the alternative hypothesis represents the research question or the expected effect or difference.
2. Choosing a Significance Level: Researchers choose a significance level (denoted as alpha, α), which is the probability of rejecting the null hypothesis when it is actually true (Type I error). Commonly used significance levels are 0.05, 0.01, and 0.001.
3. Determine the Test Statistic: Depending on the nature of the data and the hypotheses, an appropriate test statistic is selected. This could be a t-statistic, F-statistic, chi-square statistic, etc.
4. Calculate the Test Statistic: Using the sample data, the test statistic is calculated. This involves performing specific calculations that are dependent on the test being used.
5. **Determine the P-value or Use a Critical Value**: The P-value is the probability of observing a test statistic as extreme as, or more extreme than, the one calculated from the sample data assuming the null hypothesis is true. If a P-value is not calculated, a critical value from the appropriate distribution is used, based on the chosen significance level.
6. Make a Decision: If the P-value is less than or equal to the significance level, the null hypothesis is rejected in favor of the alternative hypothesis. If the P-value is greater, the null hypothesis cannot be rejected.
7.
Interpret the Results: The results are interpreted in the context of the research question. A significant result (P ≤ α) suggests that the findings are unlikely to have occurred by chance and supports the alternative hypothesis. A non-significant result (P > α) indicates that there is not enough evidence to support the alternative hypothesis.
8.
Consider Effect Size and Power: Even if a result is statistically significant, it's important to consider the effect size, which measures the magnitude of the difference or effect, and the power of the test, which is the probability of correctly rejecting a false null hypothesis.
9.
Report the Findings: The final step is to report the results, including the hypotheses, the test statistic, the P-value or critical value, the decision made, and the interpretation of the results.
Advantages and Limitations
Hypothesis testing has several advantages, including its ability to provide a clear decision-making process, its objectivity, and its wide applicability across different fields. However, it also has limitations such as the potential for Type I and Type II errors, the reliance on sample representativeness, and the possibility of misleading results if the assumptions underlying the test are violated.
Misinterpretations and Misuses
Common misinterpretations include confusing statistical significance with practical significance, overreliance on P-values, and not considering the context and limitations of the study. It's crucial for researchers to understand the principles behind hypothesis testing and to use it appropriately.
In conclusion, hypothesis testing is a powerful tool in the researcher's toolkit. It allows for rigorous evaluation of research questions and contributes to the scientific process by providing a mechanism to either support or refute hypotheses with empirical evidence.
**Hypothesis Testing in Research Methodology**
Hypothesis testing is a statistical method used to make decisions or draw conclusions about a population based on sample data. It's a systematic process that allows researchers to evaluate whether the results of their study are likely due to chance or if there is a significant relationship between variables. The process involves setting up a null hypothesis (H0) and an alternative hypothesis (H1), which are competing statements about the population parameter.
1. Formulating the Hypotheses: The first step is to clearly state the research question and formulate the null and alternative hypotheses. The null hypothesis typically represents the status quo or the assumption of no effect or no difference, while the alternative hypothesis represents the research question or the expected effect or difference.
2. Choosing a Significance Level: Researchers choose a significance level (denoted as alpha, α), which is the probability of rejecting the null hypothesis when it is actually true (Type I error). Commonly used significance levels are 0.05, 0.01, and 0.001.
3. Determine the Test Statistic: Depending on the nature of the data and the hypotheses, an appropriate test statistic is selected. This could be a t-statistic, F-statistic, chi-square statistic, etc.
4. Calculate the Test Statistic: Using the sample data, the test statistic is calculated. This involves performing specific calculations that are dependent on the test being used.
5. **Determine the P-value or Use a Critical Value**: The P-value is the probability of observing a test statistic as extreme as, or more extreme than, the one calculated from the sample data assuming the null hypothesis is true. If a P-value is not calculated, a critical value from the appropriate distribution is used, based on the chosen significance level.
6. Make a Decision: If the P-value is less than or equal to the significance level, the null hypothesis is rejected in favor of the alternative hypothesis. If the P-value is greater, the null hypothesis cannot be rejected.
7.
Interpret the Results: The results are interpreted in the context of the research question. A significant result (P ≤ α) suggests that the findings are unlikely to have occurred by chance and supports the alternative hypothesis. A non-significant result (P > α) indicates that there is not enough evidence to support the alternative hypothesis.
8.
Consider Effect Size and Power: Even if a result is statistically significant, it's important to consider the effect size, which measures the magnitude of the difference or effect, and the power of the test, which is the probability of correctly rejecting a false null hypothesis.
9.
Report the Findings: The final step is to report the results, including the hypotheses, the test statistic, the P-value or critical value, the decision made, and the interpretation of the results.
Advantages and Limitations
Hypothesis testing has several advantages, including its ability to provide a clear decision-making process, its objectivity, and its wide applicability across different fields. However, it also has limitations such as the potential for Type I and Type II errors, the reliance on sample representativeness, and the possibility of misleading results if the assumptions underlying the test are violated.
Misinterpretations and Misuses
Common misinterpretations include confusing statistical significance with practical significance, overreliance on P-values, and not considering the context and limitations of the study. It's crucial for researchers to understand the principles behind hypothesis testing and to use it appropriately.
In conclusion, hypothesis testing is a powerful tool in the researcher's toolkit. It allows for rigorous evaluation of research questions and contributes to the scientific process by providing a mechanism to either support or refute hypotheses with empirical evidence.
2024-06-16 15:53:02
reply(1)
Helpful(1122)
Helpful
Helpful(2)
Works at the International Atomic Energy Agency, Lives in Vienna, Austria.
Hypothesis Testing. When you conduct a piece of quantitative research, you are inevitably attempting to answer a research question or hypothesis that you have set. One method of evaluating this research question is via a process called hypothesis testing, which is sometimes also referred to as significance testing.
2023-06-19 04:02:35
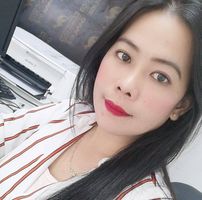
Isabella Carter
QuesHub.com delivers expert answers and knowledge to you.
Hypothesis Testing. When you conduct a piece of quantitative research, you are inevitably attempting to answer a research question or hypothesis that you have set. One method of evaluating this research question is via a process called hypothesis testing, which is sometimes also referred to as significance testing.