What is a test of a hypothesis 2024?
I'll answer
Earn 20 gold coins for an accepted answer.20
Earn 20 gold coins for an accepted answer.
40more
40more
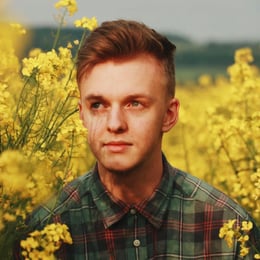
Daniel Harris
Works at Google, Lives in Mountain View, CA
As a domain expert in statistics, I am well-versed in the intricacies of hypothesis testing, a cornerstone of inferential statistics. Hypothesis testing is a process that allows us to make decisions or draw conclusions based on the data we observe. It is a systematic approach to evaluate the validity of a claim or hypothesis concerning a population parameter.
To begin with, let's understand the context in which a hypothesis test is conducted. It is often used when we want to make an inference about a population based on a sample. The process involves two competing statements about a population parameter, which are known as the null hypothesis (H0) and the alternative hypothesis (Ha or H1).
The null hypothesis is a statement of no effect or no difference. It is typically set up to be tested and potentially rejected. It represents a situation of status quo or the assumption that there is no significant relationship between variables or that a treatment has no effect.
On the other hand, the alternative hypothesis represents the research hypothesis. It is what the researcher is actually interested in and is the statement that there is an effect or a difference. It is the claim that we want to support with our data.
The process of hypothesis testing involves several steps:
1. Formulation of Hypotheses: We start by stating our null and alternative hypotheses clearly.
2. Selection of Significance Level (α): This is the probability of rejecting the null hypothesis when it is true. Commonly used levels are 0.05, 0.01, and 0.10.
3. Determination of Test Statistic: Depending on the type of hypothesis test and the data, we choose an appropriate test statistic. This could be a t-statistic, z-score, F-ratio, etc.
4. Rejection Region: We determine the critical value(s) or rejection region(s) based on the significance level and the distribution of the test statistic.
5. Collection of Data: We then collect data from a sample that is representative of the population we are interested in.
6. Calculation of the Test Statistic: Using the collected data, we calculate the value of the test statistic.
7.
Decision Making: We compare the calculated test statistic to the critical value or check if it falls into the rejection region. If it does, we reject the null hypothesis in favor of the alternative hypothesis.
8.
Interpretation of Results: Finally, we interpret the results in the context of the problem. This involves understanding the implications of our findings and communicating them effectively.
It is important to note that hypothesis testing does not prove that the null hypothesis is true; rather, it allows us to reject the null hypothesis if the data provide sufficient evidence against it. Similarly, failing to reject the null hypothesis does not prove it is true; it simply means that the evidence is not strong enough to reject it.
Moreover, hypothesis testing is subject to errors. There are two types of errors:
- Type I Error: This occurs when we reject a true null hypothesis. It is also known as a false positive.
- Type II Error: This occurs when we fail to reject a false null hypothesis. It is also known as a false negative.
The probability of making a Type I error is denoted by α (alpha), and the probability of making a Type II error is denoted by β (beta). The power of a test, which is 1 - β, represents the probability of correctly rejecting a false null hypothesis.
In conclusion, a test of a hypothesis is a rigorous method to evaluate claims about population parameters using sample data. It is a fundamental tool in statistical analysis that helps in making informed decisions based on empirical evidence.
To begin with, let's understand the context in which a hypothesis test is conducted. It is often used when we want to make an inference about a population based on a sample. The process involves two competing statements about a population parameter, which are known as the null hypothesis (H0) and the alternative hypothesis (Ha or H1).
The null hypothesis is a statement of no effect or no difference. It is typically set up to be tested and potentially rejected. It represents a situation of status quo or the assumption that there is no significant relationship between variables or that a treatment has no effect.
On the other hand, the alternative hypothesis represents the research hypothesis. It is what the researcher is actually interested in and is the statement that there is an effect or a difference. It is the claim that we want to support with our data.
The process of hypothesis testing involves several steps:
1. Formulation of Hypotheses: We start by stating our null and alternative hypotheses clearly.
2. Selection of Significance Level (α): This is the probability of rejecting the null hypothesis when it is true. Commonly used levels are 0.05, 0.01, and 0.10.
3. Determination of Test Statistic: Depending on the type of hypothesis test and the data, we choose an appropriate test statistic. This could be a t-statistic, z-score, F-ratio, etc.
4. Rejection Region: We determine the critical value(s) or rejection region(s) based on the significance level and the distribution of the test statistic.
5. Collection of Data: We then collect data from a sample that is representative of the population we are interested in.
6. Calculation of the Test Statistic: Using the collected data, we calculate the value of the test statistic.
7.
Decision Making: We compare the calculated test statistic to the critical value or check if it falls into the rejection region. If it does, we reject the null hypothesis in favor of the alternative hypothesis.
8.
Interpretation of Results: Finally, we interpret the results in the context of the problem. This involves understanding the implications of our findings and communicating them effectively.
It is important to note that hypothesis testing does not prove that the null hypothesis is true; rather, it allows us to reject the null hypothesis if the data provide sufficient evidence against it. Similarly, failing to reject the null hypothesis does not prove it is true; it simply means that the evidence is not strong enough to reject it.
Moreover, hypothesis testing is subject to errors. There are two types of errors:
- Type I Error: This occurs when we reject a true null hypothesis. It is also known as a false positive.
- Type II Error: This occurs when we fail to reject a false null hypothesis. It is also known as a false negative.
The probability of making a Type I error is denoted by α (alpha), and the probability of making a Type II error is denoted by β (beta). The power of a test, which is 1 - β, represents the probability of correctly rejecting a false null hypothesis.
In conclusion, a test of a hypothesis is a rigorous method to evaluate claims about population parameters using sample data. It is a fundamental tool in statistical analysis that helps in making informed decisions based on empirical evidence.
2024-06-16 10:05:12
reply(1)
Helpful(1122)
Helpful
Helpful(2)
Works at Facebook, Lives in Menlo Park, CA
Statistical hypothesis testing. ... A statistical hypothesis, sometimes called confirmatory data analysis, is a hypothesis that is testable on the basis of observing a process that is modeled via a set of random variables. A statistical hypothesis test is a method of statistical inference.
2023-06-22 04:02:26
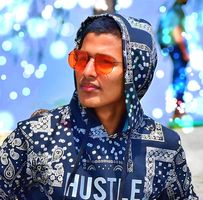
Ethan Davis
QuesHub.com delivers expert answers and knowledge to you.
Statistical hypothesis testing. ... A statistical hypothesis, sometimes called confirmatory data analysis, is a hypothesis that is testable on the basis of observing a process that is modeled via a set of random variables. A statistical hypothesis test is a method of statistical inference.