What does the F value mean?
I'll answer
Earn 20 gold coins for an accepted answer.20
Earn 20 gold coins for an accepted answer.
40more
40more

Grace Martinez
Studied at University of Michigan, Lives in Ann Arbor, MI
As a subject matter expert in statistics, I'm often asked about the meaning of the F value in the context of statistical analysis. The F value is a crucial component in various statistical tests, including the Analysis of Variance (ANOVA), which is used to compare the means of three or more groups. It's also used in regression analysis to determine the significance of the model. Let's delve into the details.
The F value is named after Sir Ronald A. Fisher, who introduced it in the 1920s as part of his work on the ANOVA test. It's a ratio that compares the variance within groups to the variance between groups. In essence, the F value is a measure of the variance explained by a factor in your model relative to the unexplained variance.
### Understanding the F Value
1. **Null Hypothesis (H0) and Alternative Hypothesis (Ha)**: When conducting an ANOVA test, you start with a null hypothesis that assumes there are no significant differences between group means. The alternative hypothesis posits that at least one group mean is different.
2. Calculation: The F value is calculated by taking the variance between groups (the mean square between groups, MSB) and dividing it by the variance within groups (the mean square within groups, MSW). The formula is F = MSB/MSW.
3. Interpretation:
- A high F value suggests that the variance between groups is large relative to the variance within groups, which means the null hypothesis is less likely to be true. It indicates that the observed differences are not likely due to random chance.
- A low F value implies that the variance within groups is similar to or larger than the variance between groups, supporting the null hypothesis that there are no significant differences between group means.
4. Significance Level (α): The F value is compared to a critical value from the F-distribution table, which is determined by your chosen significance level (commonly α = 0.05) and the degrees of freedom for both the numerator (between groups) and the denominator (within groups).
5. Degrees of Freedom (df): The F test takes into account the degrees of freedom, which is the number of values in the data set that are free to vary. For the F value, you have two degrees of freedom: one for the between-groups variance (df1 = number of groups - 1) and one for the within-groups variance (df2 = total number of observations - number of groups).
6. **Practical Significance vs. Statistical Significance**: While a high F value indicates statistical significance, it's also important to consider the practical significance. A large F value with a small effect size (the standardized difference between group means) may not be meaningful in a real-world context.
7.
Assumptions: The validity of the F test relies on several assumptions, such as the data being normally distributed, having equal variances (homoscedasticity), and the observations being independent.
8.
Multiple Comparisons: If the F test indicates significant differences, post hoc tests are often conducted to determine which specific groups differ from each other.
9.
Use in Regression: In regression analysis, the F value is used to test the null hypothesis that all regression coefficients are zero. A significant F value suggests that at least one predictor variable has a non-zero coefficient, indicating that the model is useful in predicting the outcome variable.
10.
Software and Calculators: Modern statistical software can calculate the F value and its significance, making it easier to interpret the results without manual calculations.
In conclusion, the F value is a pivotal statistic in determining whether observed differences are likely due to the effects being tested or simply due to random variation. It's a testament to the power of statistical analysis to discern patterns and make informed decisions in the face of complexity.
The F value is named after Sir Ronald A. Fisher, who introduced it in the 1920s as part of his work on the ANOVA test. It's a ratio that compares the variance within groups to the variance between groups. In essence, the F value is a measure of the variance explained by a factor in your model relative to the unexplained variance.
### Understanding the F Value
1. **Null Hypothesis (H0) and Alternative Hypothesis (Ha)**: When conducting an ANOVA test, you start with a null hypothesis that assumes there are no significant differences between group means. The alternative hypothesis posits that at least one group mean is different.
2. Calculation: The F value is calculated by taking the variance between groups (the mean square between groups, MSB) and dividing it by the variance within groups (the mean square within groups, MSW). The formula is F = MSB/MSW.
3. Interpretation:
- A high F value suggests that the variance between groups is large relative to the variance within groups, which means the null hypothesis is less likely to be true. It indicates that the observed differences are not likely due to random chance.
- A low F value implies that the variance within groups is similar to or larger than the variance between groups, supporting the null hypothesis that there are no significant differences between group means.
4. Significance Level (α): The F value is compared to a critical value from the F-distribution table, which is determined by your chosen significance level (commonly α = 0.05) and the degrees of freedom for both the numerator (between groups) and the denominator (within groups).
5. Degrees of Freedom (df): The F test takes into account the degrees of freedom, which is the number of values in the data set that are free to vary. For the F value, you have two degrees of freedom: one for the between-groups variance (df1 = number of groups - 1) and one for the within-groups variance (df2 = total number of observations - number of groups).
6. **Practical Significance vs. Statistical Significance**: While a high F value indicates statistical significance, it's also important to consider the practical significance. A large F value with a small effect size (the standardized difference between group means) may not be meaningful in a real-world context.
7.
Assumptions: The validity of the F test relies on several assumptions, such as the data being normally distributed, having equal variances (homoscedasticity), and the observations being independent.
8.
Multiple Comparisons: If the F test indicates significant differences, post hoc tests are often conducted to determine which specific groups differ from each other.
9.
Use in Regression: In regression analysis, the F value is used to test the null hypothesis that all regression coefficients are zero. A significant F value suggests that at least one predictor variable has a non-zero coefficient, indicating that the model is useful in predicting the outcome variable.
10.
Software and Calculators: Modern statistical software can calculate the F value and its significance, making it easier to interpret the results without manual calculations.
In conclusion, the F value is a pivotal statistic in determining whether observed differences are likely due to the effects being tested or simply due to random variation. It's a testament to the power of statistical analysis to discern patterns and make informed decisions in the face of complexity.
2024-04-18 02:58:14
reply(1)
Helpful(1122)
Helpful
Helpful(2)
Works at the International Fund for Agricultural Development, Lives in Rome, Italy.
A high F value means that your data does not well support your null hypothesis. Or in other words, the alternative hypothesis is compatible with observed data.May 11, 2016
2023-06-23 03:22:04
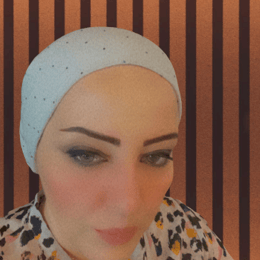
Harper Martin
QuesHub.com delivers expert answers and knowledge to you.
A high F value means that your data does not well support your null hypothesis. Or in other words, the alternative hypothesis is compatible with observed data.May 11, 2016