What is the central limit theorem for dummies?
I'll answer
Earn 20 gold coins for an accepted answer.20
Earn 20 gold coins for an accepted answer.
40more
40more

Harper Allen
Studied at the University of Zurich, Lives in Zurich, Switzerland.
As an expert in the field of statistics, I'm often asked to explain complex concepts in a way that's accessible to everyone. The Central Limit Theorem (CLT) is one of those concepts that can be daunting at first glance, but it's actually quite straightforward once you grasp the basics. So, let's dive in and break it down.
The Central Limit Theorem is a fundamental theorem in statistics that describes the distribution of sample means. It's important to understand what a "sample" is before we go any further. A sample is a subset of a larger population. For example, if you wanted to know the average height of all people in a country, it would be impractical to measure everyone. Instead, you might take a sample of 1,000 people from various locations and age groups to represent the larger population.
Now, let's talk about the Central Limit Theorem. The CLT states that given a sufficiently large sample size from a population with a finite level of variance, the mean of all samples from the same population will be approximately normally distributed. This is true regardless of the shape of the population's distribution. The key points to take away from this are:
1. Sample Size: The theorem requires a large enough sample size. The general rule of thumb is at least 30 samples, but the larger the sample size, the better the approximation to the normal distribution.
2. Population Variance: The population from which samples are taken must have a finite variance. This means that the data can't be too spread out or have extreme outliers that would distort the mean.
3. Distribution Shape: The original distribution of the population doesn't matter. Whether it's skewed, uniform, or any other shape, the distribution of sample means will tend towards normal as the sample size increases.
4. Approximation: The CLT is an approximation. As the sample size increases, the distribution of sample means gets closer and closer to a normal distribution.
5. Independence: The samples must be independent of each other. This means that the selection of one sample doesn't influence the selection of another.
The practical implications of the CLT are vast. It's the reason why many statistical tests, like the t-test and ANOVA, assume normality. Even when the original data isn't normal, these tests can still be used because the CLT tells us that the sample means will be normally distributed if the sample size is large enough.
Now, let's talk about why the CLT is so powerful. It allows us to make inferences about a population based on a sample. Without the CLT, we would need to know the entire population's distribution to make accurate predictions, which is often impossible. But with the CLT, we can take a sample, calculate its mean, and use that to estimate the population mean with a certain level of confidence.
In summary, the Central Limit Theorem is a statistical workhorse that underpins much of inferential statistics. It's the reason we can generalize from samples to populations, and it's the reason we can use a variety of statistical tests even when the data isn't perfectly normal. It's a theorem that, once understood, can unlock a deeper understanding of how statistics work in the real world.
The Central Limit Theorem is a fundamental theorem in statistics that describes the distribution of sample means. It's important to understand what a "sample" is before we go any further. A sample is a subset of a larger population. For example, if you wanted to know the average height of all people in a country, it would be impractical to measure everyone. Instead, you might take a sample of 1,000 people from various locations and age groups to represent the larger population.
Now, let's talk about the Central Limit Theorem. The CLT states that given a sufficiently large sample size from a population with a finite level of variance, the mean of all samples from the same population will be approximately normally distributed. This is true regardless of the shape of the population's distribution. The key points to take away from this are:
1. Sample Size: The theorem requires a large enough sample size. The general rule of thumb is at least 30 samples, but the larger the sample size, the better the approximation to the normal distribution.
2. Population Variance: The population from which samples are taken must have a finite variance. This means that the data can't be too spread out or have extreme outliers that would distort the mean.
3. Distribution Shape: The original distribution of the population doesn't matter. Whether it's skewed, uniform, or any other shape, the distribution of sample means will tend towards normal as the sample size increases.
4. Approximation: The CLT is an approximation. As the sample size increases, the distribution of sample means gets closer and closer to a normal distribution.
5. Independence: The samples must be independent of each other. This means that the selection of one sample doesn't influence the selection of another.
The practical implications of the CLT are vast. It's the reason why many statistical tests, like the t-test and ANOVA, assume normality. Even when the original data isn't normal, these tests can still be used because the CLT tells us that the sample means will be normally distributed if the sample size is large enough.
Now, let's talk about why the CLT is so powerful. It allows us to make inferences about a population based on a sample. Without the CLT, we would need to know the entire population's distribution to make accurate predictions, which is often impossible. But with the CLT, we can take a sample, calculate its mean, and use that to estimate the population mean with a certain level of confidence.
In summary, the Central Limit Theorem is a statistical workhorse that underpins much of inferential statistics. It's the reason we can generalize from samples to populations, and it's the reason we can use a variety of statistical tests even when the data isn't perfectly normal. It's a theorem that, once understood, can unlock a deeper understanding of how statistics work in the real world.
2024-04-03 04:00:55
reply(1)
Helpful(1122)
Helpful
Helpful(2)
Studied at the University of Oxford, Lives in Oxford, UK.
The Central Limit Theorem (CLT for short) basically says that for non-normal data, the distribution of the sample means has an approximate normal distribution, no matter what the distribution of the original data looks like, as long as the sample size is large enough (usually at least 30) and all samples have the same ...
2023-06-27 03:21:51
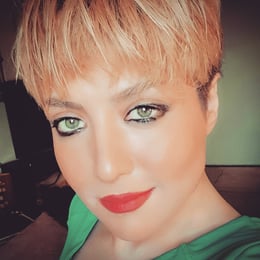
Zoe Campbell
QuesHub.com delivers expert answers and knowledge to you.
The Central Limit Theorem (CLT for short) basically says that for non-normal data, the distribution of the sample means has an approximate normal distribution, no matter what the distribution of the original data looks like, as long as the sample size is large enough (usually at least 30) and all samples have the same ...