Is pr f the p value?
I'll answer
Earn 20 gold coins for an accepted answer.20
Earn 20 gold coins for an accepted answer.
40more
40more
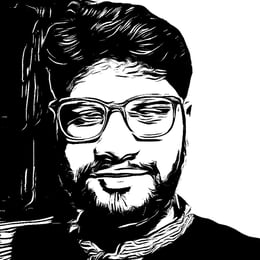
Lucas Carter
Works at Google, Lives in Mountain View. Holds a degree in Computer Science from Stanford University.
Hello there! As an expert in statistical analysis, I'm here to help clarify some misconceptions about statistical terms and their interpretations. Let's dive into the relationship between the p-value and the F-statistic, and how they are used in hypothesis testing.
Firstly, it's important to understand what a p-value is. The p-value is a statistic that measures the strength of the evidence against the null hypothesis. Specifically, it's the probability of obtaining a test statistic as extreme as, or more extreme than, the one calculated from my sample data assuming that the null hypothesis is true. In other words, it's a way to quantify the surprise we might feel if the null hypothesis were actually true.
Now, let's talk about the F-statistic. The F-statistic is a scalar that measures the ratio of the variance between groups to the variance within groups. It's commonly used in analysis of variance (ANOVA) and regression analysis. The F-statistic is calculated from the test statistic, which is the square of the standardized coefficient (beta coefficient) in the case of regression.
When we talk about "Pr > F," we're referring to the p-value associated with the F-statistic. This p-value is used to evaluate the null hypothesis that a given predictor (in the context of regression) has no effect on the outcome variable. The null hypothesis is a statement of no effect or no difference, and it's what we test against our alternative hypothesis, which posits that there is an effect or a difference.
Here's how it works in practice:
1. Set up the Hypotheses: The first step is to define the null hypothesis (H0) and the alternative hypothesis (H1). The null hypothesis typically states that there is no effect or no relationship between the variables.
2. Calculate the Test Statistic: Next, you calculate the F-statistic from your sample data. This involves looking at the variance explained by the model (the regression line) and the variance not explained (the residuals).
3. Determine the p-value: After calculating the F-statistic, you use a statistical table or software to find the associated p-value. This tells you the probability of observing a test statistic as extreme as yours if the null hypothesis were true.
4. Make a Decision: You compare the p-value to your chosen significance level, often denoted by α (alpha). If the p-value is less than α, you reject the null hypothesis in favor of the alternative. If it's greater, you fail to reject the null hypothesis.
5. Interpret the Results: The decision to reject or not reject the null hypothesis leads to a conclusion about whether the evidence supports the existence of an effect or relationship.
It's crucial to remember that a low p-value does not mean that the null hypothesis is false, nor does a high p-value confirm it. It simply indicates the strength of the evidence against the null hypothesis.
Now, let's move on to the translation part.
Firstly, it's important to understand what a p-value is. The p-value is a statistic that measures the strength of the evidence against the null hypothesis. Specifically, it's the probability of obtaining a test statistic as extreme as, or more extreme than, the one calculated from my sample data assuming that the null hypothesis is true. In other words, it's a way to quantify the surprise we might feel if the null hypothesis were actually true.
Now, let's talk about the F-statistic. The F-statistic is a scalar that measures the ratio of the variance between groups to the variance within groups. It's commonly used in analysis of variance (ANOVA) and regression analysis. The F-statistic is calculated from the test statistic, which is the square of the standardized coefficient (beta coefficient) in the case of regression.
When we talk about "Pr > F," we're referring to the p-value associated with the F-statistic. This p-value is used to evaluate the null hypothesis that a given predictor (in the context of regression) has no effect on the outcome variable. The null hypothesis is a statement of no effect or no difference, and it's what we test against our alternative hypothesis, which posits that there is an effect or a difference.
Here's how it works in practice:
1. Set up the Hypotheses: The first step is to define the null hypothesis (H0) and the alternative hypothesis (H1). The null hypothesis typically states that there is no effect or no relationship between the variables.
2. Calculate the Test Statistic: Next, you calculate the F-statistic from your sample data. This involves looking at the variance explained by the model (the regression line) and the variance not explained (the residuals).
3. Determine the p-value: After calculating the F-statistic, you use a statistical table or software to find the associated p-value. This tells you the probability of observing a test statistic as extreme as yours if the null hypothesis were true.
4. Make a Decision: You compare the p-value to your chosen significance level, often denoted by α (alpha). If the p-value is less than α, you reject the null hypothesis in favor of the alternative. If it's greater, you fail to reject the null hypothesis.
5. Interpret the Results: The decision to reject or not reject the null hypothesis leads to a conclusion about whether the evidence supports the existence of an effect or relationship.
It's crucial to remember that a low p-value does not mean that the null hypothesis is false, nor does a high p-value confirm it. It simply indicates the strength of the evidence against the null hypothesis.
Now, let's move on to the translation part.
2024-05-12 10:07:36
reply(1)
Helpful(1122)
Helpful
Helpful(2)
Studied at the Sorbonne University, Lives in Paris.
Pr > F -C This is the p-value associated with the F statistic of a given effect and test statistic. The null hypothesis that a given predictor has no effect on either of the outcomes is evaluated with regard to this p-value. For a given alpha level, if the p-value is less than alpha, the null hypothesis is rejected.
2023-06-24 03:14:10

Alexander Adams
QuesHub.com delivers expert answers and knowledge to you.
Pr > F -C This is the p-value associated with the F statistic of a given effect and test statistic. The null hypothesis that a given predictor has no effect on either of the outcomes is evaluated with regard to this p-value. For a given alpha level, if the p-value is less than alpha, the null hypothesis is rejected.