What is a significant p value?
I'll answer
Earn 20 gold coins for an accepted answer.20
Earn 20 gold coins for an accepted answer.
40more
40more

Oliver Wilson
Works at the International Renewable Energy Agency, Lives in Abu Dhabi, UAE.
As a statistical expert with a deep understanding of hypothesis testing and its implications, I can provide a comprehensive explanation of what a significant p-value is and why it's crucial in statistical analysis.
A p-value, which stands for probability value, is a statistical measure that indicates the strength of the evidence against the null hypothesis. The null hypothesis is a statement that there is no effect or no association between variables, and it's used as a starting point in hypothesis testing. The alternative hypothesis, on the other hand, is what researchers are usually interested in; it posits that there is an effect or an association.
In the majority of analyses, an alpha of 0.05 is used as the cutoff for significance. This alpha level is a threshold that determines when we reject the null hypothesis. If the p-value is less than 0.05, we reject the null hypothesis and conclude that a significant difference does exist. This decision is based on the premise that we are willing to accept a 5% risk of concluding that a difference exists when, in reality, there is none (a false positive).
The p-value is calculated by considering the most extreme results that could occur by chance if the null hypothesis were true. For example, if we're testing the effectiveness of a new drug, the null hypothesis might state that the drug has no effect. The p-value would then represent the probability of observing a result as extreme as, or more extreme than, the one actually observed if the drug truly had no effect.
It's important to note that a p-value does not measure the probability that the null hypothesis is true or the probability that the alternative hypothesis is true. Instead, it's the probability of the observed data under the assumption that the null hypothesis is true.
Another critical aspect to consider is the concept of statistical significance versus practical significance. A result can be statistically significant (i.e., have a small p-value) but may not be practically significant if the effect size is very small. In other words, the difference might be real, but it might not be meaningful or important in a real-world context.
Moreover, the use of a p-value as a binary decision tool (significant or not significant) has been criticized. Some argue that it oversimplifies the complexity of research findings and can lead to misinterpretation. Instead, researchers are encouraged to report the effect size, confidence intervals, and other measures that provide a more nuanced understanding of the results.
Lastly, the determination of significance is also influenced by the study design, sample size, and the variability within the data. A larger sample size, for instance, can lead to a smaller p-value for the same effect size, making it more likely to be deemed statistically significant.
In conclusion, a significant p-value is a statistical tool that helps researchers make decisions about whether to reject the null hypothesis. It is a cornerstone of hypothesis testing, but it should be interpreted within the broader context of the study's design, the effect size, and the practical implications of the findings.
A p-value, which stands for probability value, is a statistical measure that indicates the strength of the evidence against the null hypothesis. The null hypothesis is a statement that there is no effect or no association between variables, and it's used as a starting point in hypothesis testing. The alternative hypothesis, on the other hand, is what researchers are usually interested in; it posits that there is an effect or an association.
In the majority of analyses, an alpha of 0.05 is used as the cutoff for significance. This alpha level is a threshold that determines when we reject the null hypothesis. If the p-value is less than 0.05, we reject the null hypothesis and conclude that a significant difference does exist. This decision is based on the premise that we are willing to accept a 5% risk of concluding that a difference exists when, in reality, there is none (a false positive).
The p-value is calculated by considering the most extreme results that could occur by chance if the null hypothesis were true. For example, if we're testing the effectiveness of a new drug, the null hypothesis might state that the drug has no effect. The p-value would then represent the probability of observing a result as extreme as, or more extreme than, the one actually observed if the drug truly had no effect.
It's important to note that a p-value does not measure the probability that the null hypothesis is true or the probability that the alternative hypothesis is true. Instead, it's the probability of the observed data under the assumption that the null hypothesis is true.
Another critical aspect to consider is the concept of statistical significance versus practical significance. A result can be statistically significant (i.e., have a small p-value) but may not be practically significant if the effect size is very small. In other words, the difference might be real, but it might not be meaningful or important in a real-world context.
Moreover, the use of a p-value as a binary decision tool (significant or not significant) has been criticized. Some argue that it oversimplifies the complexity of research findings and can lead to misinterpretation. Instead, researchers are encouraged to report the effect size, confidence intervals, and other measures that provide a more nuanced understanding of the results.
Lastly, the determination of significance is also influenced by the study design, sample size, and the variability within the data. A larger sample size, for instance, can lead to a smaller p-value for the same effect size, making it more likely to be deemed statistically significant.
In conclusion, a significant p-value is a statistical tool that helps researchers make decisions about whether to reject the null hypothesis. It is a cornerstone of hypothesis testing, but it should be interpreted within the broader context of the study's design, the effect size, and the practical implications of the findings.
2024-04-10 06:24:35
reply(1)
Helpful(1122)
Helpful
Helpful(2)
Studied at the University of Sydney, Lives in Sydney, Australia.
When you perform a hypothesis test in statistics, a p-value helps you determine the significance of your results. ... The p-value is a number between 0 and 1 and interpreted in the following way: A small p-value (typically -- 0.05) indicates strong evidence against the null hypothesis, so you reject the null hypothesis.
2023-06-20 03:14:04
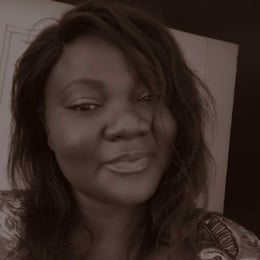
Harper Cooper
QuesHub.com delivers expert answers and knowledge to you.
When you perform a hypothesis test in statistics, a p-value helps you determine the significance of your results. ... The p-value is a number between 0 and 1 and interpreted in the following way: A small p-value (typically -- 0.05) indicates strong evidence against the null hypothesis, so you reject the null hypothesis.