What does the level of significance tell us 2024?
I'll answer
Earn 20 gold coins for an accepted answer.20
Earn 20 gold coins for an accepted answer.
40more
40more
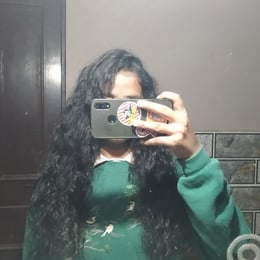
Charlotte Richardson
Studied at the University of Lagos, Lives in Lagos, Nigeria.
As a statistician with a keen interest in data analysis, I am often asked about the importance of statistical significance. The level of significance is a fundamental concept in statistical testing that helps us interpret the results of our data analysis. It is a measure of the likelihood that the observed results are not due to chance but are indicative of a real effect or relationship.
In statistical testing, we often start with a null hypothesis, which is a statement of no effect or no relationship between variables. The alternative hypothesis, on the other hand, posits that there is an effect or relationship. The significance level, typically denoted by alpha (α), is the probability of rejecting the null hypothesis when it is actually true. It is a threshold that we set before conducting the test to decide when the results are statistically significant.
The significance level is crucial for several reasons:
1. Decision Making: It helps us make decisions about the null hypothesis. If the p-value (the probability of observing the data given that the null hypothesis is true) is less than the significance level, we reject the null hypothesis and conclude that there is a statistically significant effect.
2. Type I Error: The significance level also determines the maximum probability of making a Type I error, which is the error of rejecting a true null hypothesis. By setting a low significance level, we can minimize the risk of such errors.
3. Power of the Test: The power of a test is the probability of correctly rejecting a false null hypothesis. There is a trade-off between the significance level and the power of the test. A lower significance level increases the risk of a Type II error (failing to reject a false null hypothesis), which can be mitigated by increasing the sample size.
4. Replicability: A statistically significant result increases the likelihood that the findings can be replicated in future studies. It provides a benchmark for other researchers to build upon.
5. Scientific Communication: In scientific publications, the level of significance is often used as a criterion for determining whether the results are noteworthy and should be reported.
6. Practical Significance: While statistical significance tells us about the likelihood of the observed effect being due to chance, it does not always equate to practical significance. A result might be statistically significant but have little practical impact if the effect size is small.
7.
Multiple Testing: When conducting multiple statistical tests, the likelihood of finding at least one significant result by chance increases. This is known as the multiple comparisons problem, and adjustments to the significance level are often necessary to control the family-wise error rate.
8.
Ethical Considerations: In fields like medicine and psychology, the significance level can have ethical implications. For instance, a treatment might be statistically significant but not clinically significant, meaning it does not provide meaningful benefits to patients.
In conclusion, the level of significance is a critical tool in statistical analysis that helps us determine the reliability of our findings. It is not just about rejecting or failing to reject the null hypothesis; it is about understanding the balance between the risks of Type I and Type II errors, the power of our tests, and the practical implications of our results.
In statistical testing, we often start with a null hypothesis, which is a statement of no effect or no relationship between variables. The alternative hypothesis, on the other hand, posits that there is an effect or relationship. The significance level, typically denoted by alpha (α), is the probability of rejecting the null hypothesis when it is actually true. It is a threshold that we set before conducting the test to decide when the results are statistically significant.
The significance level is crucial for several reasons:
1. Decision Making: It helps us make decisions about the null hypothesis. If the p-value (the probability of observing the data given that the null hypothesis is true) is less than the significance level, we reject the null hypothesis and conclude that there is a statistically significant effect.
2. Type I Error: The significance level also determines the maximum probability of making a Type I error, which is the error of rejecting a true null hypothesis. By setting a low significance level, we can minimize the risk of such errors.
3. Power of the Test: The power of a test is the probability of correctly rejecting a false null hypothesis. There is a trade-off between the significance level and the power of the test. A lower significance level increases the risk of a Type II error (failing to reject a false null hypothesis), which can be mitigated by increasing the sample size.
4. Replicability: A statistically significant result increases the likelihood that the findings can be replicated in future studies. It provides a benchmark for other researchers to build upon.
5. Scientific Communication: In scientific publications, the level of significance is often used as a criterion for determining whether the results are noteworthy and should be reported.
6. Practical Significance: While statistical significance tells us about the likelihood of the observed effect being due to chance, it does not always equate to practical significance. A result might be statistically significant but have little practical impact if the effect size is small.
7.
Multiple Testing: When conducting multiple statistical tests, the likelihood of finding at least one significant result by chance increases. This is known as the multiple comparisons problem, and adjustments to the significance level are often necessary to control the family-wise error rate.
8.
Ethical Considerations: In fields like medicine and psychology, the significance level can have ethical implications. For instance, a treatment might be statistically significant but not clinically significant, meaning it does not provide meaningful benefits to patients.
In conclusion, the level of significance is a critical tool in statistical analysis that helps us determine the reliability of our findings. It is not just about rejecting or failing to reject the null hypothesis; it is about understanding the balance between the risks of Type I and Type II errors, the power of our tests, and the practical implications of our results.
2024-06-01 13:35:14
reply(1)
Helpful(1122)
Helpful
Helpful(2)
Works at the International Criminal Police Organization (INTERPOL), Lives in Lyon, France.
Tests for statistical significance are used to estimate the probability that a relationship observed in the data occurred only by chance; the probability that the variables are really unrelated in the population. They can be used to filter out unpromising hypotheses.
2023-06-19 03:14:01

Isabella Kim
QuesHub.com delivers expert answers and knowledge to you.
Tests for statistical significance are used to estimate the probability that a relationship observed in the data occurred only by chance; the probability that the variables are really unrelated in the population. They can be used to filter out unpromising hypotheses.