How do you know if P value is significant?
I'll answer
Earn 20 gold coins for an accepted answer.20
Earn 20 gold coins for an accepted answer.
40more
40more
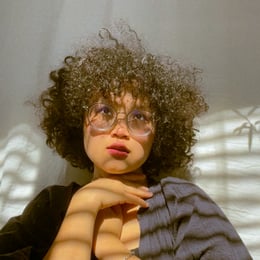
Madeline Cook
Studied at University of Cambridge, Lives in Cambridge, UK
As a statistical expert with extensive experience in data analysis and hypothesis testing, I have worked with numerous datasets across various fields, including economics, biology, and social sciences. My expertise lies in helping researchers and professionals interpret statistical results, including the significance of p-values in hypothesis testing.
When conducting a hypothesis test, we often aim to determine whether there is enough evidence to reject the null hypothesis in favor of the alternative hypothesis. The p-value plays a crucial role in this process. It is a measure of the strength of the evidence against the null hypothesis. Here's a step-by-step guide on how to interpret p-values and determine significance:
1. Understanding the Null Hypothesis: The null hypothesis (H0) is a statement of no effect or no difference. It is the default assumption that there is no relationship between the variables being studied.
2. Defining the Alternative Hypothesis: The alternative hypothesis (H1 or Ha) is what you might believe to be true, and it's what you're testing against the null hypothesis.
3. Setting the Significance Level (α): Before you conduct the test, you must decide on the significance level, which is the probability of rejecting the null hypothesis when it is actually true (Type I error). Common significance levels are 0.05, 0.01, and 0.001.
4. Calculating the P-value: The p-value is the probability of observing a test statistic as extreme as, or more extreme than, the one calculated from your sample data, assuming the null hypothesis is true.
5. **Comparing the P-value to the Significance Level**: If the p-value is less than or equal to the significance level, you reject the null hypothesis. This suggests that the results are statistically significant.
6. Interpreting the Result: If the p-value is smaller than the significance level, you have evidence to suggest that the alternative hypothesis might be true. It indicates that the observed effect is unlikely to have occurred by chance alone.
7.
Considering the Effect Size: Even if the p-value is significant, it's important to consider the effect size, which measures the magnitude of the difference or relationship.
8.
Avoiding P-value Misinterpretation: A common mistake is to interpret a small p-value as the probability that the null hypothesis is true, which is incorrect. The p-value is the probability of the observed data under the assumption that the null hypothesis is true.
9.
Recognizing the Limitations: P-values do not provide evidence for the alternative hypothesis, nor do they measure the size of an effect. They are also influenced by sample size, which can lead to significant results even when the effect is practically insignificant.
10.
Reporting the Results: When reporting, it's important to state both the p-value and the significance level used in the test. For example, if the significance level is 5%, and the p-value is less than 0.05, you would report it as "p < 0.05".
In conclusion, the significance of a p-value is determined by comparing it to a pre-decided significance level. A p-value that is smaller than this level indicates that there is a low probability of observing the data under the null hypothesis, and thus, provides evidence against it. However, it's crucial to interpret p-values in the context of the study design, effect size, and potential biases.
When conducting a hypothesis test, we often aim to determine whether there is enough evidence to reject the null hypothesis in favor of the alternative hypothesis. The p-value plays a crucial role in this process. It is a measure of the strength of the evidence against the null hypothesis. Here's a step-by-step guide on how to interpret p-values and determine significance:
1. Understanding the Null Hypothesis: The null hypothesis (H0) is a statement of no effect or no difference. It is the default assumption that there is no relationship between the variables being studied.
2. Defining the Alternative Hypothesis: The alternative hypothesis (H1 or Ha) is what you might believe to be true, and it's what you're testing against the null hypothesis.
3. Setting the Significance Level (α): Before you conduct the test, you must decide on the significance level, which is the probability of rejecting the null hypothesis when it is actually true (Type I error). Common significance levels are 0.05, 0.01, and 0.001.
4. Calculating the P-value: The p-value is the probability of observing a test statistic as extreme as, or more extreme than, the one calculated from your sample data, assuming the null hypothesis is true.
5. **Comparing the P-value to the Significance Level**: If the p-value is less than or equal to the significance level, you reject the null hypothesis. This suggests that the results are statistically significant.
6. Interpreting the Result: If the p-value is smaller than the significance level, you have evidence to suggest that the alternative hypothesis might be true. It indicates that the observed effect is unlikely to have occurred by chance alone.
7.
Considering the Effect Size: Even if the p-value is significant, it's important to consider the effect size, which measures the magnitude of the difference or relationship.
8.
Avoiding P-value Misinterpretation: A common mistake is to interpret a small p-value as the probability that the null hypothesis is true, which is incorrect. The p-value is the probability of the observed data under the assumption that the null hypothesis is true.
9.
Recognizing the Limitations: P-values do not provide evidence for the alternative hypothesis, nor do they measure the size of an effect. They are also influenced by sample size, which can lead to significant results even when the effect is practically insignificant.
10.
Reporting the Results: When reporting, it's important to state both the p-value and the significance level used in the test. For example, if the significance level is 5%, and the p-value is less than 0.05, you would report it as "p < 0.05".
In conclusion, the significance of a p-value is determined by comparing it to a pre-decided significance level. A p-value that is smaller than this level indicates that there is a low probability of observing the data under the null hypothesis, and thus, provides evidence against it. However, it's crucial to interpret p-values in the context of the study design, effect size, and potential biases.
2024-04-27 20:02:08
reply(1)
Helpful(1122)
Helpful
Helpful(2)
Works at the International Air Transport Association, Lives in Montreal, Canada.
The p-value is compared with the desired significance level of our test and, if it is smaller, the result is significant. That is, if the null hypothesis were to be rejected at the 5% significance level, this would be reported as --p < 0.05".Small p-values suggest that the null hypothesis is unlikely to be true.
2023-06-18 03:13:56

Oliver White
QuesHub.com delivers expert answers and knowledge to you.
The p-value is compared with the desired significance level of our test and, if it is smaller, the result is significant. That is, if the null hypothesis were to be rejected at the 5% significance level, this would be reported as --p < 0.05".Small p-values suggest that the null hypothesis is unlikely to be true.