What does the F value tell you in Anova 2024?
I'll answer
Earn 20 gold coins for an accepted answer.20
Earn 20 gold coins for an accepted answer.
40more
40more
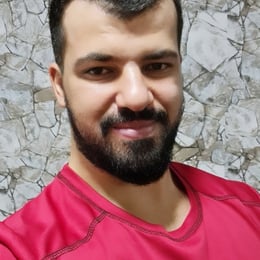
Julian Ward
Works at the International Fund for Agricultural Development, Lives in Rome, Italy.
As a data analyst with extensive experience in statistical methods, I often encounter the F-value in the context of ANOVA (Analysis of Variance) and regression analysis. The F-value is a critical measure that provides insights into the significance of the differences between group means in a dataset. Let's delve into what the F-value signifies and how it is used in statistical analysis.
When you perform an ANOVA test, you are essentially comparing the variance within groups to the variance between groups. The F-value is the ratio of these two variances. It is calculated as:
\[ F = \frac{\text{Mean Square Between (MSB)}}{\text{Mean Square Within (MSW)}} \]
Here, MSB is the variance between the group means, and MSW is the variance within the groups. A high F-value indicates that there is a significant difference between the group means, suggesting that the variation is not due to chance alone.
### Significance of the F-value
1. Hypothesis Testing: The F-value is used in conjunction with a significance level (often denoted as α, typically 0.05 or 0.01) to test the null hypothesis, which typically states that there is no difference between the group means.
2. Degrees of Freedom: The calculation of the F-value takes into account the degrees of freedom for both the numerator (between groups) and the denominator (within groups). These degrees of freedom are critical in determining the appropriate F-distribution to compare the calculated F-value against.
3. Comparison with F-distribution: The calculated F-value is compared with a critical value from the F-distribution table, which is based on the chosen significance level and the degrees of freedom. If the calculated F-value exceeds the critical value, you reject the null hypothesis, indicating that there is a statistically significant difference between the group means.
4. Effect Size: While the F-value tells you if there is a significant difference, it does not tell you how large that difference is. For this, you would look at other measures such as eta-squared (η²), which is a measure of effect size.
5. Multiple Comparisons: In the case of multiple groups, the F-value can indicate significance, but further analysis is often needed to determine which specific groups differ from each other. This is where post-hoc tests come into play.
6. Assumptions: The validity of the F-value relies on several assumptions being met, such as the normality of the data, homogeneity of variances, and independence of observations.
7.
Robustness: The F-test is fairly robust to violations of the normality assumption, especially with large sample sizes. However, if the variances are not equal (homogeneity of variance assumption is violated), alternative tests like Welch's ANOVA may be more appropriate.
8.
Practical Significance: It's important to remember that statistical significance (as indicated by the F-value) does not necessarily imply practical significance. The context and magnitude of the differences should also be considered.
In summary, the F-value in ANOVA is a pivotal statistic that helps determine whether observed differences between group means are likely due to the effect of the independent variable or simply due to random variation. It is a tool for hypothesis testing that, when properly interpreted and applied, can provide valuable insights into the structure of the data and the potential impact of different factors on the outcome variable.
When you perform an ANOVA test, you are essentially comparing the variance within groups to the variance between groups. The F-value is the ratio of these two variances. It is calculated as:
\[ F = \frac{\text{Mean Square Between (MSB)}}{\text{Mean Square Within (MSW)}} \]
Here, MSB is the variance between the group means, and MSW is the variance within the groups. A high F-value indicates that there is a significant difference between the group means, suggesting that the variation is not due to chance alone.
### Significance of the F-value
1. Hypothesis Testing: The F-value is used in conjunction with a significance level (often denoted as α, typically 0.05 or 0.01) to test the null hypothesis, which typically states that there is no difference between the group means.
2. Degrees of Freedom: The calculation of the F-value takes into account the degrees of freedom for both the numerator (between groups) and the denominator (within groups). These degrees of freedom are critical in determining the appropriate F-distribution to compare the calculated F-value against.
3. Comparison with F-distribution: The calculated F-value is compared with a critical value from the F-distribution table, which is based on the chosen significance level and the degrees of freedom. If the calculated F-value exceeds the critical value, you reject the null hypothesis, indicating that there is a statistically significant difference between the group means.
4. Effect Size: While the F-value tells you if there is a significant difference, it does not tell you how large that difference is. For this, you would look at other measures such as eta-squared (η²), which is a measure of effect size.
5. Multiple Comparisons: In the case of multiple groups, the F-value can indicate significance, but further analysis is often needed to determine which specific groups differ from each other. This is where post-hoc tests come into play.
6. Assumptions: The validity of the F-value relies on several assumptions being met, such as the normality of the data, homogeneity of variances, and independence of observations.
7.
Robustness: The F-test is fairly robust to violations of the normality assumption, especially with large sample sizes. However, if the variances are not equal (homogeneity of variance assumption is violated), alternative tests like Welch's ANOVA may be more appropriate.
8.
Practical Significance: It's important to remember that statistical significance (as indicated by the F-value) does not necessarily imply practical significance. The context and magnitude of the differences should also be considered.
In summary, the F-value in ANOVA is a pivotal statistic that helps determine whether observed differences between group means are likely due to the effect of the independent variable or simply due to random variation. It is a tool for hypothesis testing that, when properly interpreted and applied, can provide valuable insights into the structure of the data and the potential impact of different factors on the outcome variable.
2024-06-16 15:30:38
reply(1)
Helpful(1122)
Helpful
Helpful(2)
Studied at University of California, Berkeley, Lives in San Francisco. Entrepreneur passionate about technology and innovation.
An F statistic is a value you get when you run an ANOVA test or a regression analysis to find out if the means between two populations are significantly different.Dec 26, 2017
2023-06-20 03:13:53
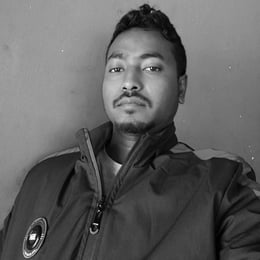
Lucas Davis
QuesHub.com delivers expert answers and knowledge to you.
An F statistic is a value you get when you run an ANOVA test or a regression analysis to find out if the means between two populations are significantly different.Dec 26, 2017